The Journey of AI: Past Milestones and Future Directions
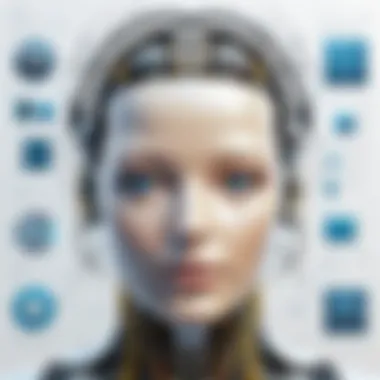
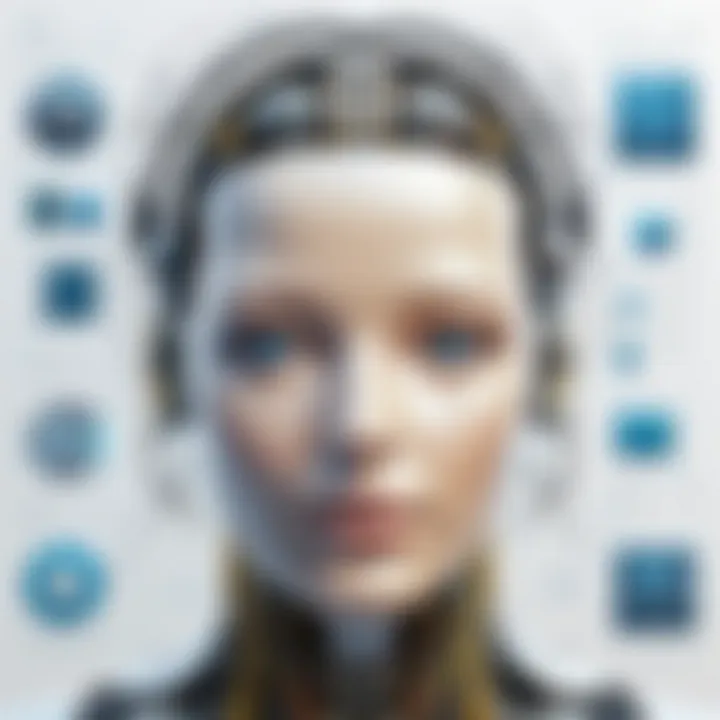
Intro
Artificial intelligence has transitioned from theoretical concepts and laboratory experiments into a real force shaping industries and daily life. In the beginning, the term itself was often clouded in mystery, with visions of machines that could think and reason. Different historical figures have made marked contributions to what we now understand AI to be, leading us to this moment of both excitement and trepidation regarding its future.
As we delve into the evolution of artificial intelligence, it is essential to recognize the foundations that were built upon the hard work of visionary thinkers. From Alan Turing's pioneering work in computation to the recent breakthroughs in neural networks, the journey of AI is rich with innovation and transformation. Each milestone not only shares a piece of history but also helps us grasp the functionality and implications of today's technologies.
The narrative of AI's development is not merely about the technology itself; it encompasses discussions on ethics, societal impacts, and future directions. This piece will guide you through understanding how AI has evolved, the principles driving its advancements, and what could lie ahead as we continue to balance human responsibility and technological growth.
Expect to encounter not only technical insights but also philosophical explorations that question the very fabric of intelligence and consciousness. With a lens focused on both past and future, the following sections will provide a well-rounded overview that speaks to both enthusiasts and professionals in the field.
The Historical Framework of Artificial Intelligence
Understanding the historical framework of artificial intelligence provides a crucial context for appreciating its advancements and implications. This section traces the roots and significant moments that shaped AI, allowing readers to gain insights into how technology developed and what influenced its path. Knowing where AI came from can illuminate current trends and future directions, shedding light on why certain technologies flourish and others falter.
Origins of Artificial Intelligence
The origins of artificial intelligence can be found in ancient myths and stories that pondered the idea of intelligent machines. However, it wasn’t until the mid-20th century that tangible concepts began to materialize. One might say that the spark for AI ignited with the work of pioneers like Alan Turing and John McCarthy.
Alan Turing, a British mathematician, conceptualized a machine that could simulate any human brain process through what’s called a "universal machine." He set the stage for modern computing and asked the pivotal question: "Can machines think?" In 1956, John McCarthy, an American computer scientist, coined the term "artificial intelligence" during the Dartmouth Conference, which marked the birth of AI as an academic field. This conference, often referred to as the birthplace of AI, brought together talented minds who laid the groundwork for future explorations in this evolving domain.
The Early Years of AI Research
Early AI research was akin to a double-edged sword. While it held great promise, it often faced skepticism and challenges. The 1950s and 1960s were characterized by optimistic predictions, where researchers wrote programs that demonstrated rudimentary problem-solving capabilities. Notably, programs like the Logic Theorist and General Problem Solver showcased AI's potential by solving mathematical problems or optimizing solutions.
However, as research progressed into the 1970s, the challenges became more pronounced. The limitations of existing technologies and a lack of substantial computing power led to what is now referred to as the "AI winter," a period in which funding and interest in AI research dramatically declined. Many questioned whether machines could ever truly replicate human intelligence, relegating AI to the sidelines.
Significant Milestones in AI Development
The path of AI has been punctuated by significant milestones. One such milestone came in 1997 when IBM's Deep Blue defeated world chess champion Garry Kasparov, a pivotal moment that showcased AI's potential in surpassing human intellect in specific tasks. AI hadn't just entered the room; it had taken a seat at the head of the table.
As the years rolled on, the development of machine learning, particularly deep learning techniques, changed the dynamics of what AI could achieve. The introduction of large datasets and robust computing power breathed new life into AI, culminating in breakthroughs in areas like image recognition and natural language processing. For instance, Google’s AlphaGo beat Go champion Lee Sedol in 2016, another landmark pointing to the amazing strides AI can make in understanding complex strategies.
Then we entered a more collaborative era. AI systems began to play well with humans, integrating into daily applications like recommendation algorithms, virtual assistants, and smart home devices. This period saw AI transforming industries—from healthcare with predictive analytics to finance with real-time fraud detection. It was clear that AI was not just a tool but an indispensable part of modern life and business.
Theoretical Foundations of AI
Understanding the theoretical foundations of artificial intelligence is crucial as it sets the stage for why AI behaves the way it does, influences its development, and shapes future innovations. This section dives into the philosophical underpinnings, fundamental tests of intelligence, and the methodologies that guide machine learning. By grasping these theories, IT professionals, software developers, and students can better navigate the complex landscape of AI technologies.
Key Philosophical Concepts
Philosophical debates surrounding AI touch upon essential questions about intelligence, consciousness, and the very nature of knowledge. Several foundational concepts stem from these discussions:
- Descartes’ Mind-Body Problem: This addresses whether artificial systems can possess consciousness akin to human beings. Are machines truly capable of thought, or do they merely simulate human cognition?
- The Chinese Room Argument: Proposed by John Searle, this thought experiment questions if a computer running a program can truly "understand" language or if it is simply manipulating symbols without comprehension.
- Ethics of AI: Debates on whether machines can or should make moral decisions present another dimension, especially as we integrate AI into sectors like healthcare, finance, and autonomous driving.
These philosophical concepts aren't mere academic musings; they influence the design of algorithms, the direction of research funding, and the public's trust in developing AI technologies.
The Turing Test and Its Implications
In the realm of AI, one of the most well-known philosophical frameworks is the Turing Test, first posed by Alan Turing in 1950. The essence of this evaluation is simple yet profound: can a machine exhibit intelligent behavior indistinguishable from that of a human?
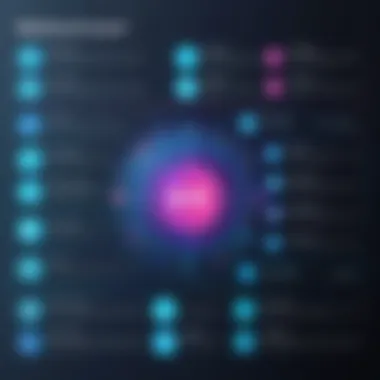
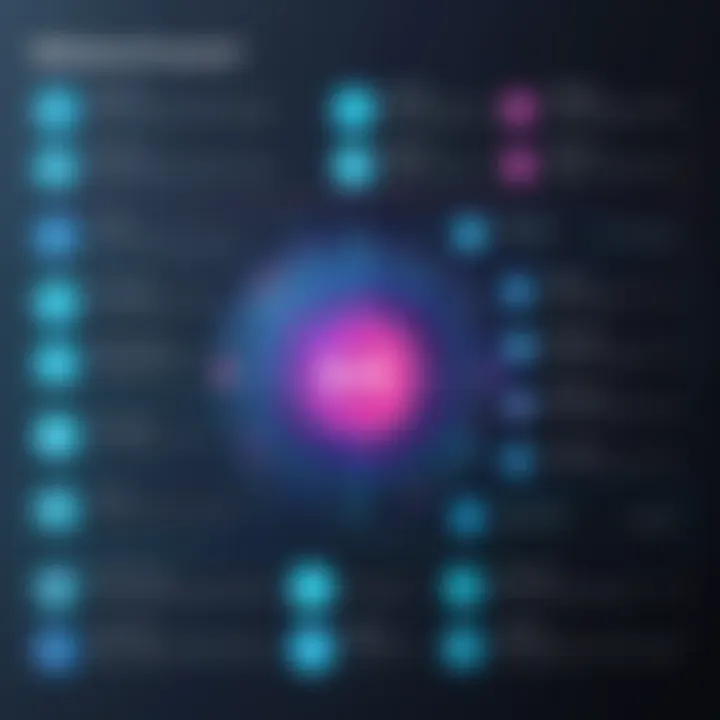
The Turing Test serves not only as a benchmark for machine intelligence but also prompts discussions on the boundaries of consciousness.
While passing the test does not equate to true understanding or consciousness, it raises important questions about what we define as intelligence. Turing's proposition carries significant implications:
- Defining Intelligence: It forces us to consider what attributes we value in intelligence and how we recognize it.
- Impacts on AI Evaluation: The test has influenced AI research, pushing developers to create more sophisticated systems that can interact naturally with humans.
- Philosophical Challenges: Numerous critiques challenge its validity, suggesting that the test does not fully account for the nuances of human intelligence and understanding.
Approaches to Machine Learning
Diving deeper into the practicalities of AI, there are several approaches to machine learning, each rooted in theoretical constructs.
- Supervised Learning: This technique involves training a model on a labeled dataset, where the input-output relationship is defined. Developers tinker with algorithms to optimize performance, often leveraging tools like Python's scikit-learn library.
- Unsupervised Learning: Contrary to supervised learning, this method looks for patterns in data without predetermined labels. Here, clustering and dimensionality reduction come into play, leading to applications in market segmentation and anomaly detection.
- Reinforcement Learning: Taking cues from behavioral psychology, this approach relies on agents learning to make decisions by receiving rewards or penalties. It's a dynamic way to train AI, enhancing its ability to solve complex problems over time.
- Examples include image recognition and spam detection.
- Think of it as a detective trying to solve a case without knowing the suspects.
- In gaming or robotics, agents learn through trial and error, improving their strategies based on feedback.
These foundational theories underline how AI advances, showcasing the blend of philosophical inquiry and practical application. As these approaches evolve, so too does the landscape of AI, making it imperative for today's tech professionals to stay ahead of the game.
Technological Advancements in AI
Technological advancements in artificial intelligence have revolutionized the way we interact with technology, paving the way for remarkable developments that influence various sectors. These advancements are not just trends; they represent a fundamental shift in how machines think, learn, and ultimately operate in our daily lives. Understanding the role of these advancements is crucial for grasping the broader implications of AI on society, the economy, and interpersonal dynamics.
The Role of Data in AI Development
At the heart of AI development lies data, often dubbed the "new oil" of our era. Data fuels statistical algorithms enabling machines to learn from patterns and make predictions. The sheer volume of data generated daily—be it from social media, online transactions, or sensors—has made it imperative to harness this information effectively.
- Big Data Analytics: Companies now utilize big data analytics to extract actionable insights. For instance, retail giants like Amazon gather data on consumer behavior, driving their recommendation systems which suggest products tailored to individual preferences.
- Quality Over Quantity: While large amounts of data are beneficial, data quality plays a significant role. Poor quality data can lead to incorrect models and biases. Take, for example, an AI system trained on skewed data that lacks diversity; the outcomes may not accurately reflect broader societal views.
"AI can't shine without a substantial and clean dataset—it’s the fuel that keeps the engine running."
Neural Networks and Deep Learning
Neural networks are the backbone of many modern AI systems. Inspired by the human brain, these systems consist of interconnected nodes or neurons, making them remarkably adept at recognizing patterns. Deep learning, a subset of neural networks, enables the processing of data in layers, extracting more complex features as information passes through.
- Applications: Deep learning has found applications in various areas, including image and speech recognition.
- For instance, Google Photos uses deep learning to sort and search through vast libraries of images.
- Similarly, voice assistants like Apple's Siri employ deep learning for natural-sounding speech recognition and response.
- Limitations: However, these systems are not without their challenges. Training deep learning models requires substantial computing power and data. Additionally, interpretability remains an issue; understanding how a model reaches a particular decision can often feel like deciphering hieroglyphics.
Natural Language Processing Breakthroughs
Natural Language Processing, or NLP, has seen remarkable growth, enabling machines to understand and respond to human language more effectively. This domain of AI is essential for applications that involve text and speech. NLP combines linguistics and machine learning to analyze and generate human language.
- Impact on Communication: Companies like OpenAI and Google have made leaps with language models that can generate coherent text and assist in various tasks, from customer service chatbots to automated content generation.
- Ethical Concerns: Yet, the rise of NLP also raises ethical questions. The potential for leveraging the technology to create misleading information or deepfakes poses a real threat to truth and transparency in communication.
In summary, technological advancements in AI are not just technical achievements but represent a shift in various aspects of life and work. The integration of data, neural networks, and natural language processing forms a triad essential for understanding the current landscape of AI. As these technologies evolve, so too will both their implications and applications in our world.
Current Applications of AI
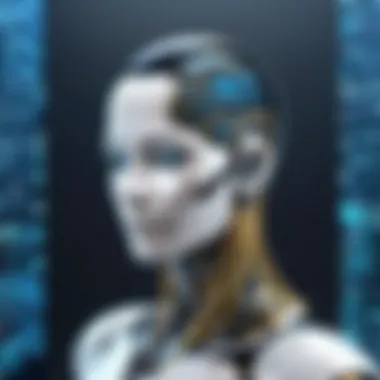
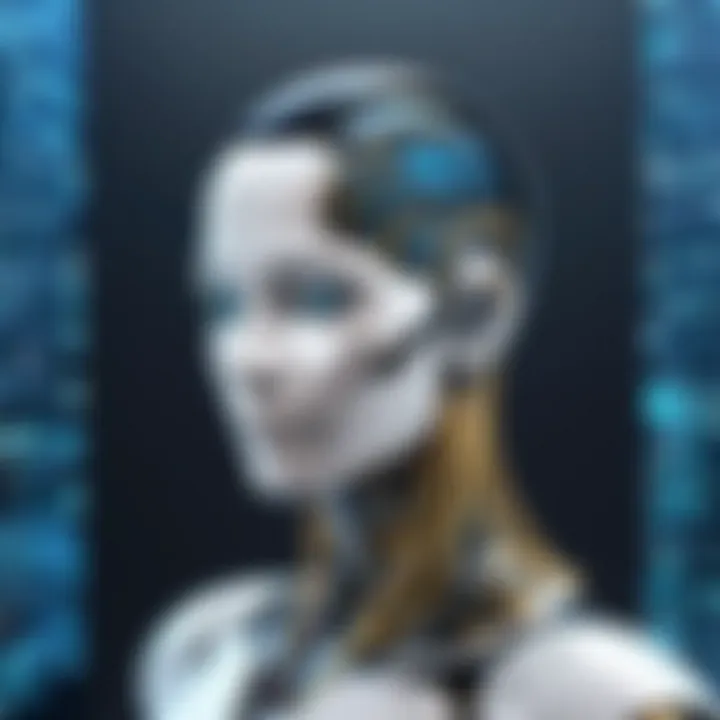
Artificial Intelligence is no longer confined to the realm of science fiction. It has seeped into various sectors of everyday life, becoming an indispensable part of modern infrastructure. In this section, we will delve into some prominent applications of AI, particularly in healthcare, financial services, and automation. These technologies not only streamline processes but also make them more efficient and accurate, allowing for innovations that impact society positively.
AI in Healthcare
AI's influence in healthcare is profound, reshaping how diagnostics, treatment planning, and patient care are approached. For instance, IBM Watson Health uses AI to analyze massive amounts of medical data, assisting doctors in diagnosing diseases more accurately and rapidly than ever before. Imagine a doctor with access to the medical knowledge of seasoned professionals across the globe at their fingertips. This capability significantly enhances clinical decision-making.
Moreover, predictive analytics, powered by AI, can forecast patient outcomes and optimize treatment strategies. For instance, hospitals use AI tools to predict patient deterioration based on historical data. As a result, healthcare professionals can intervene proactively, potentially saving lives.
"AI is changing the very foundation of healthcare, turning mountains of data into valuable insights that can save lives."
AI in Financial Services
In the financial sector, AI is transforming how institutions operate. Automated trading systems use algorithms to analyze market trends, allowing traders to execute orders more efficiently. Companies like Goldman Sachs utilize AI to identify risks and make informed investment decisions, maintaining a competitive edge in a fast-paced environment.
Additionally, AI plays a crucial role in enhancing customer experience. Chatbots and virtual assistants are becoming common, assisting customers with queries and transactions 24/7. This not only saves time for both parties but also personalizes the user journey, making finance less intimidating. Through AI-driven analytics, financial firms can also detect fraudulent activities almost in real-time, ensuring the safety of customer data.
AI in Automation and Robotics
Automation powered by AI is revolutionizing industries. In manufacturing, robots equipped with AI technology can perform tasks with precision and efficiency, reducing human error. Companies such as Boston Dynamics are at the forefront, developing robots capable of intricate tasks that were once thought possible only for humans.
Furthermore, AI-driven automation doesn't just stop at factories. It extends into logistics with systems that optimize shipping routes and inventory management, dramatically reducing operational costs. In agricultural settings, AI is transforming traditional farming practices through precision agriculture, allowing farmers to optimize resource use and increase productivity.
By integrating AI into the core of these sectors, enterprises are not only driving down costs but also setting the stage for future growth. The possibilities are endless, and the effectiveness of AI applications continues to expand as technology evolves.
By understanding the current applications of AI, we can appreciate its potential to blend seamlessly into everyday life, fundamentally altering various sectors for the better.
Ethical Considerations in AI Evolution
In the bustling landscape of technology, where innovation often races ahead, ethical considerations in artificial intelligence ring alarm bells that cannot be ignored. As AI stitches itself deeper into the fabric of our daily lives, from medical diagnostics to social media algorithms, it prompts introspection about the responsibilities we hold as creators and users of such powerful tools. Recognizing these ethical dimensions is not just about compliance with legal standards; it’s about fostering a future where technology aligns with human values and enhances well-being, rather than undermining it.
Moral Implications of AI Decision-Making
Artificial intelligence systems, particularly those employed in critical sectors like healthcare and criminal justice, have decision-making capabilities that can significantly affect people's lives. Consider a scenario where an AI system denies an individual's insurance claim based on data inputs that simulate risk profiles. The moral crux here lies in determining how these algorithms prioritize factors and what biases may inadvertently shape their choices. As machines take on roles traditionally held by humans, we must grapple with questions of accountability and fairness. In the absence of a human conscience, how do we ensure that AI systems operate ethically?
Moreover, the question of transparency arises. Users deserve to understand the reasons behind certain decisions. The more opaque an AI system becomes, the harder it is to accept its authority in decision-making processes. It’s akin to having a black box churn out verdicts without a clue about the inner workings—hardly a recipe for trust.
"The ethical landscape for AI is not a mere footnote but a cornerstone of its evolution."
Bias and Fairness in AI Algorithms
The algorithms that drive AI often reflect the data on which they are trained, imperfect though that training data may be. This reality introduces a critical ethical concern: bias. If historical data reflect societal prejudices, the AI trained on such data is likely to perpetuate them. For example, an AI recruitment tool could disadvantage candidates from specific demographic groups simply because past hiring practices were biased.
In light of such challenges, addressing bias means not only cleaning and curating data but also continuously measuring and refining these algorithms post-deployment. Collaborating with diverse teams to develop these systems can also bring fresh perspectives and help identify potential pitfalls. It’s not merely about creating fair algorithms but ensuring they are nurtured in environments where fairness is a cultural norm.
Privacy Concerns with AI Technologies
As AI permeates our everyday lives, the question of privacy can feel like walking on a tightrope. Many AI technologies thrive on vast amounts of personal data. By analyzing social media activity, shopping habits, or even health records, these systems become more effective. However, this data-driven approach raises critical concerns about who gets access to what data, how it is stored, and how it is used.
Individuals often surrender their data without fully understanding the trade-offs involved. This lack of awareness can open floodgates to data misuse, leading to potential harm. Tools such as machine learning can also make inferences about individuals that go beyond mere statistical correlation. A seemingly benign dataset could reveal sensitive information, like someone's health condition or political affiliation.
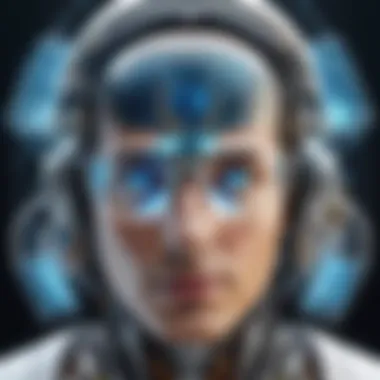
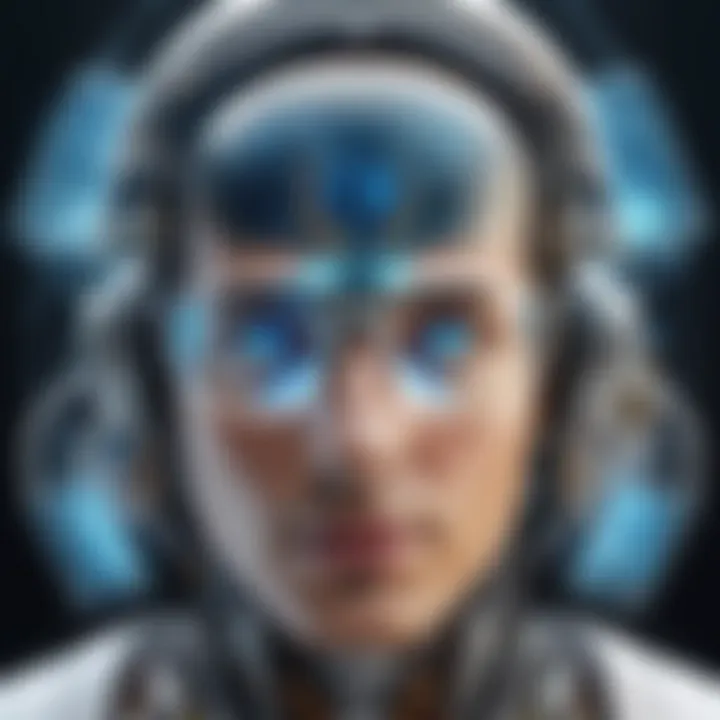
Legitimate concerns about privacy necessitate robust frameworks. These frameworks must not only protect individual rights but also promote accountability for organizations that manage sensitive information. Establishing the principle of informed consent, clarity on data use, and rigorous security practices are vital steps in the journey towards ethical AI.
As we steer towards a future interwoven with AI, acknowledging ethical considerations is crucial. It lays the groundwork for responsible development, ensuring these technologies advance in harmony with the values we hold dear.
For more in-depth discussions on AI ethics, check sources like Wikipedia or articles on Britannica.
Future Trajectories of Artificial Intelligence
The realm of artificial intelligence (AI) is swirling with possibilities that could reshape our understanding of technology in profound ways. This section digs deeper into the concept of future trajectories of AI, highlighting why it matters. It's not just about what’s coming next, but also about understanding the broader implications – how these advancements could influence various sectors of life and industry.
As we prepare ourselves for the future of AI, it's important to grasp the significant benefits and considerations swirling around. Technologies and systems emerge at a dizzying pace, and being aware of these trends helps us anticipate and adjust to future shifts in both the tech landscape and our daily lives.
Predicted Technological Advancements
Emerging technologies are always a hot topic in discussions about AI's future. In this terrain, experts predict advancements in machine learning strategies, increasing computational power, and a growing emphasis on interpretability and transparency in AI models.
For example, the ability to process large data sets with unprecedented speed could eventually lead to new tools that assist in medical diagnosis or environmental sustainability efforts. Key advancements might include:
- Quantum Computing: With the potential to process information in ways classical computers can't, quantum computing could majorly boost AI capabilities, particularly in complex simulations and optimizations.
- Explainable AI (XAI): As AI systems become integral in high-stakes decisions, the demand for systems that can explain their reasoning is increasing. This concept is crucial for accountability and trust in AI applications.
- Integration with the Internet of Things (IoT): As smart devices become ubiquitous, the synergy between AI and IoT could enhance our understanding of patterns in real-time data, driving smarter cities and homes.
The trajectory of AI technology is both exciting and somewhat daunting; it beckons us to think critically about how we integrate these systems into our world.
The Potential of General AI
General AI represents a tantalizing yet controversial aspect of the future landscape of artificial intelligence. Unlike narrow AI, which specializes in specific tasks, general AI envisions a machine possessing the ability to understand, learn, and apply intelligence across multiple domains, much like a human. Although we may still be in the early innings of this concept, discussions about its potential raise important questions about control, ethics, and collaboration.
Some of the aspects to ponder include:
- Enhanced Decision-Making: General AI could streamline complex decision-making processes, leading to drastically improved efficiency in various fields, such as disaster response or urban planning.
- Limits on Autonomy: With great power comes great responsibility. There are valid concerns about who programs these systems and how they might develop their own objectives that diverge from human interests.
- Philosophical Implications: The emergence of general AI may force us to reevaluate the essence of consciousness, identity, and what it means to be human. This brings rich philosophical discussions into focus, as we question our relationship with intelligent machines.
The implications are vast, leaving us to navigate a landscape that is fraught with both possibility and uncertainty.
Impacts on Employment and Society
With AI's rapid advancements, one of the most pressing topics involves its impacts on employment and broader society. Many professionals are eyeing the horizon, both excited and anxious at the thought of job displacement, while others grapple with new opportunities and roles that emerge as a result of AI integration.
Key considerations include:
- Job Transformation: While certain jobs may disappear, new roles will likely arise. Workers skilled in AI management, data analysis, and ethical oversight could become increasingly valuable.
- Education and Reskilling: Adaptation will demand a focus on education and reskilling initiatives to prepare the workforce for evolving roles. This creates collaborative opportunities for educational institutions and industries.
- Societal Challenges: As automation increases, society might experience significant shifts in economic structures and social dynamics, raising questions about wealth distribution and access to technology.
In essence, the trajectory AI takes not only steers its development but also necessitates an ongoing dialogue about how we navigate these changes in employment and societal structures.
"To reshape the future, we must first understand the nuances of today's technology and its evolving implications.”
All in all, as we peer into the future of artificial intelligence, being aware of these potential advancements and their impacts will help us be prepared for the transformation awaiting us.
End: Reflections on AI's Journey
As we wrap up this exploration into the evolution of artificial intelligence, it's clear the discussion doesn't stop here. The story of AI, from its humble beginnings to its current prominence, sheds light on numerous pivotal elements worth contemplating. Each aspect of this evolution serves as a bridge to understand the broader implications of this technology on our daily lives.
Throughout the article, we've discussed significant milestones that have shaped AI, emphasizing how early research laid the groundwork for the sophisticated systems we see today. Early concepts like the Turing Test prompted reconsideration of what it means for machines to think. This has not only fuelled academic inquiry but also practical application, making it relevant in real-world scenarios.
Let’s take a moment to consider the following points:
- Technological Impact: The advancements in neural networks and natural language processing have revolutionized industries ranging from healthcare to finance. Understanding how these technologies emerged is crucial for anyone in IT-related fields, as it provides context for future developments.
- Philosophical Implications: The ethical discussions surrounding AI's evolution are as significant as the technological progress. Topics such as bias in algorithms or the moral implications of machine decision-making raise important questions about responsibility and accountability.
- Societal Change: We can’t ignore how AI’s trajectory is influencing society. With advancements predicted in general AI, the impact on employment and the dynamics of everyday life can't be understated. As such, a nuanced understanding of these developments is key for software developers and technology professionals alike.
“The journey of AI is not just about machines; it's about the world we live in.”