Exploring the Significance of SAS in Analytics: A Comprehensive Overview
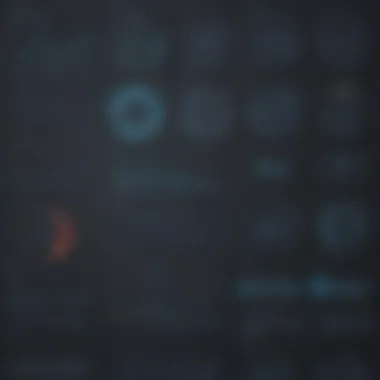
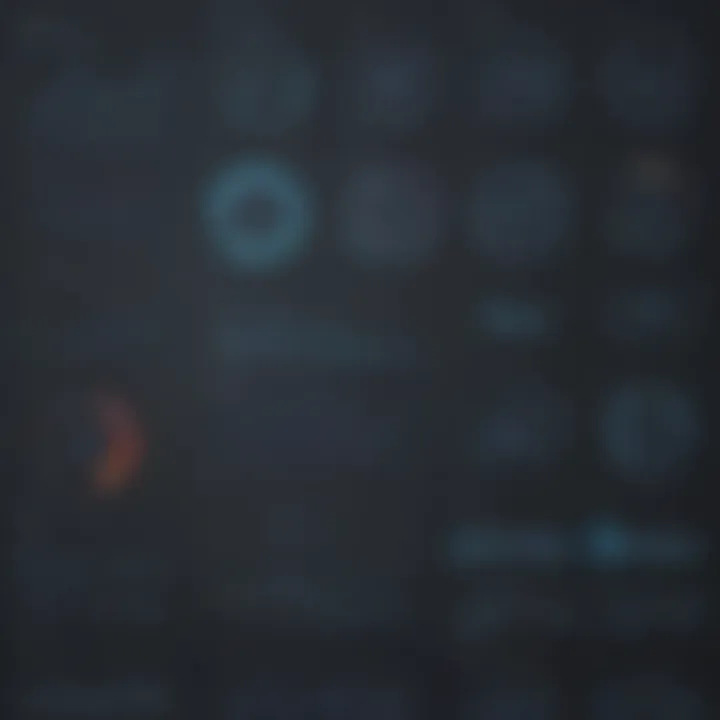
Software Overview
SAS (Statistical Analysis System) stands as a powerful tool in the realm of analytics. With a rich history tracing back decades, SAS has cemented its position as a leading software choice in data analysis. Its main features encompass a wide array of statistical functions, data manipulation tools, and sophisticated visualization capabilities. Navigating through the SAS interface proves to be intuitive, offering a seamless user experience even for beginners. Moreover, SAS boasts strong compatibility with various operating systems and integrates well with other popular analytical tools, enhancing its utility in diverse tech environments.
Pros and Cons
In terms of strengths, SAS excels in its robust statistical analysis features, providing advanced modeling techniques for data interpretation. Additionally, its comprehensive data management capabilities offer users the flexibility to handle large datasets efficiently. However, one of the notable weaknesses of SAS lies in its relatively high learning curve, which may pose a challenge for novice users accustomed to more user-friendly interfaces. When compared to similar software like R and Python, SAS often stands out for its enterprise-level offerings and extensive customer support options.
Pricing and Plans
When evaluating pricing and plans, SAS offers distinct subscription options tailored to varied user needs. From individual licenses to enterprise packages, users can choose a plan that aligns with their budget and requirements. Furthermore, SAS provides a free trial, enabling potential customers to explore its features before committing to a paid subscription. The value for money aspect of SAS hinges on the user's specific analytic needs and the level of support required, making it essential to assess the features vis-a-vis the pricing structure for optimal decision-making.
Expert Verdict
Introduction to SAS in Analytics
In this article, we embark on a thorough exploration of the pivotal role played by SAS (Statistical Analysis System) in the realm of analytics. Understanding the intricacies of SAS is crucial for software developers, IT professionals, and students alike, as it serves as a foundational tool in data analysis processes. Delving into the nuances of SAS sheds light on its multifaceted applications and significance within the analytics landscape, offering invaluable insights into the complexities of this robust system.
Definition of SAS
Why SAS is Important in Analytics
The significance of SAS in analytics lies in its unparalleled ability to streamline complex data processing and analysis tasks. SAS serves as a cornerstone within the field of analytics by providing a comprehensive suite of tools for data manipulation, statistical modeling, and report generation. Its robust features facilitate data-driven decision-making, empowering users to extract actionable insights from vast datasets efficiently.
Evolution of SAS
The Origins of SAS
The origins of SAS trace back to its inception as a project at North Carolina State University, where it was developed to assist in agricultural research. Over time, SAS evolved into a full-fledged statistical analysis tool, gaining widespread recognition for its reliability and capability to handle diverse data analysis tasks. The principles established during its nascent stages continue to underpin SAS's functionality, making it a versatile and indispensable tool in modern analytics.
Development of SAS Over Time
The evolution of SAS over the years has been marked by continuous innovation and adaptation to changing technological landscapes. With each iteration, SAS has incorporated advanced features and capabilities to meet the evolving demands of data analytics. From enhanced data visualization tools to sophisticated machine learning algorithms, SAS has remained at the forefront of analytical tools, catering to the diverse needs of professionals across various industries.
Key Features of SAS
Data Management
At the core of SAS lies its robust data management capabilities, allowing users to efficiently organize, clean, and manipulate large datasets. The intuitive interface and advanced data handling functions make SAS a preferred choice for handling complex data structures and ensuring data consistency and accuracy.
Statistical Analysis Capabilities
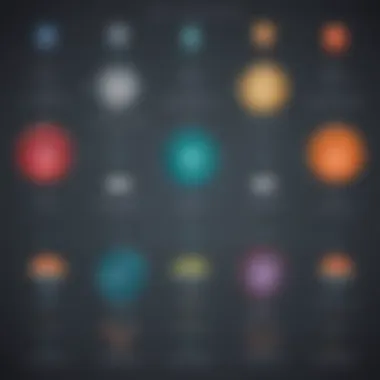
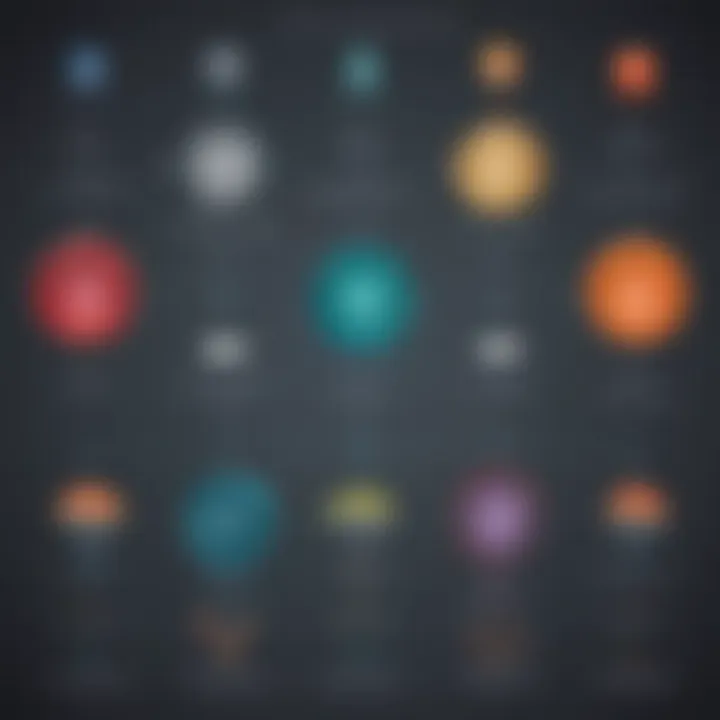
SAS's statistical analysis capabilities set it apart from traditional data analysis tools, offering a wide array of statistical functions for hypothesis testing, regression analysis, and predictive modeling. The seamless integration of statistical procedures simplifies the analytical process, enabling users to derive meaningful insights from data with ease.
Graphical Representation
The graphical representation features of SAS provide users with dynamic visualization tools for presenting data in a comprehensive and visually appealing manner. From customizable graphs to interactive dashboards, SAS excels in transforming raw data into insightful visualizations, enhancing the communicative power of analytics outcomes.
Applications of SAS in Analytics
In this crucial section of the comprehensive guide dedicated to understanding SAS in analytics, we delve into the vital applications of SAS software. As a cornerstone of data analysis, SAS plays a pivotal role in modern analytics, offering a robust platform for various analytical tasks. Organizations worldwide rely on SAS for its exceptional data management capabilities, in-depth statistical analysis features, and advanced graphical representation tools. The integration of SAS into analytical workflows enhances efficiency, accuracy, and the overall quality of data-driven decision-making processes.
Data Analysis
Statistical Modeling
Statistical modeling is a fundamental aspect of analytical processes facilitated by SAS software. By employing statistical models, analysts can derive meaningful insights from vast datasets, uncover patterns, and make reliable predictions based on historical data. The proficiency of SAS in statistical modeling lies in its ability to handle complex algorithms, large datasets, and diverse variables with precision and efficiency. The unique feature of SAS in statistical modeling is its adaptability to various regression techniques, time series analysis, and predictive modeling, offering unparalleled flexibility in exploring data relationships and making informed decisions. While statistical modeling in SAS empowers analysts with robust analytical capabilities, ensuring accurate results, it may entail a steep learning curve for novice users.
Predictive Analytics
Predictive analytics stands out as a key component of SAS applications in analytics, leveraging historical data to forecast future trends and outcomes. SAS excels in predictive analytics by integrating advanced machine learning algorithms, artificial intelligence techniques, and sophisticated modeling tools, enabling organizations to proactively identify patterns, mitigate risks, and optimize resource allocation. The distinctive feature of SAS in predictive analytics is its predictive modeling environment, empowering users to build, validate, and deploy predictive models seamlessly. While predictive analytics in SAS offers strategic advantages in decision-making and performance optimization, it demands meticulous data preparation, model tuning, and validation processes to ensure reliable predictions.
Business Intelligence
Reporting and Dashboards
Reporting and dashboards play a pivotal role in harnessing insights from data analytics, enabling stakeholders to visualize and interpret data effectively. SAS empowers users with interactive reporting tools, customizable dashboards, and intuitive visualization features that facilitate data-driven decision-making. The key characteristic of SAS in reporting and dashboards is its comprehensive reporting capabilities, allowing users to generate customizable reports, perform ad-hoc analysis, and share insights across organizational levels. However, the complexity of report creation and dashboard customization in SAS may pose challenges for users with limited technical expertise.
Decision Support Systems
Decision support systems offered by SAS provide decision-makers with valuable insights, recommendations, and predictive models to drive strategic initiatives. SAS enables the integration of data analytics into decision-making processes, facilitating evidence-based decisions, scenario analysis, and risk assessment. The unique feature of SAS decision support systems lies in their ability to integrate with diverse data sources, conduct sensitivity analysis, and streamline decision workflows. While SAS decision support systems enhance the analytical capabilities of organizations, ensuring data accuracy, their implementation may require significant resources, training, and organizational alignment.
Healthcare Analytics
Patient Data Analysis
In healthcare analytics, patient data analysis serves as a cornerstone for understanding health trends, optimizing patient care, and improving clinical outcomes. SAS enables healthcare providers to analyze patient data comprehensively, identify patterns, and tailor medical interventions based on predictive insights. The key characteristic of SAS in patient data analysis is its compatibility with healthcare data standards, regulatory requirements, and privacy protocols, ensuring data security and compliance. The unique feature of SAS in patient data analysis is its integration of clinical data, genomic information, and Io T data streams, facilitating holistic patient care management. However, the complexity of healthcare data analysis in SAS necessitates specialized training, domain knowledge, and continuous monitoring for accurate outcomes.
Disease Outbreak Prediction
Disease outbreak prediction emerges as a critical application of SAS in healthcare analytics, enabling early detection, timely response, and effective mitigation strategies. SAS equips healthcare organizations with predictive models, epidemiological tools, and data visualization capabilities to forecast disease outbreaks, analyze transmission patterns, and allocate resources efficiently. The distinctive feature of SAS in disease outbreak prediction is its real-time data processing, geospatial analytics, and trend monitoring functionalities, enabling rapid response and containment measures. While disease outbreak prediction in SAS enhances healthcare preparedness and response mechanisms, it requires robust data infrastructure, interdisciplinary collaboration, and continuous model refinement to address evolving healthcare challenges.
Benefits of Using SAS in Analytics
In the realm of analytics, the utilization of SAS (Statistical Analysis System) holds paramount importance in optimizing data processes and enhancing analytical outcomes. The significance of employing SAS stems from its multifaceted benefits that encompass efficiency, accuracy, and adaptability across diverse industry domains. By delving into the efficiency and accuracy aspects provided by SAS, users can harness automated processes and robust data analysis capabilities to streamline operations and elevate decision-making processes.
Efficiency and Accuracy
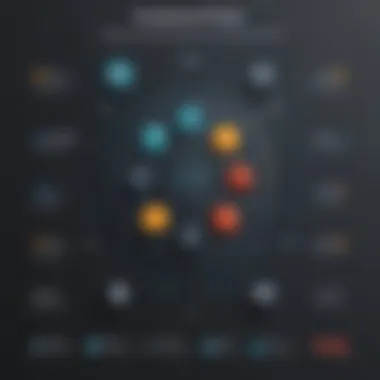
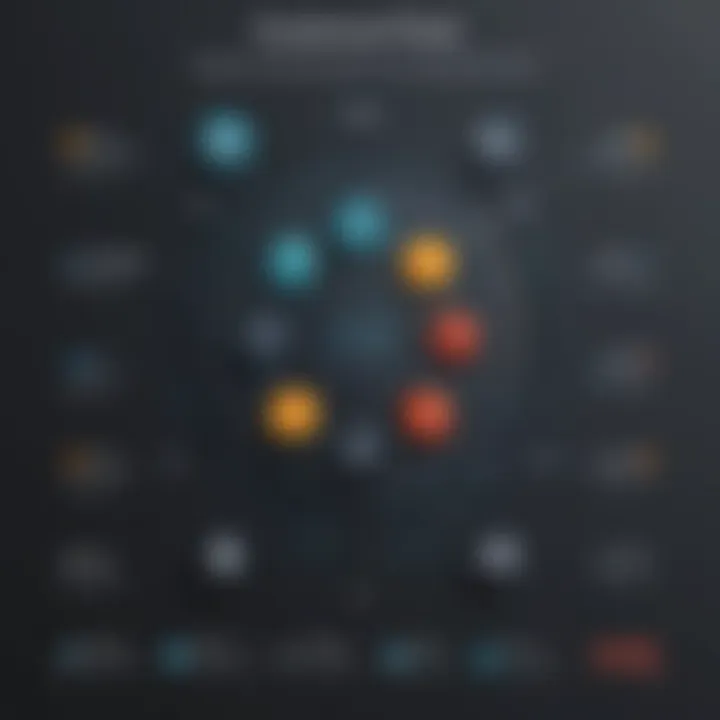
Automation of Processes
The automation of processes within SAS plays a crucial role in expediting data management tasks and statistical analysis procedures. By integrating automation features, users can significantly reduce manual intervention, thus minimizing errors and enhancing overall operational efficiency. This automation aspect within SAS not only saves time but also ensures consistent and reliable results, making it a popular choice for organizations seeking precision and speed in their analytics workflows.
Robust Data Analysis
On the other hand, robust data analysis capabilities offered by SAS empower users to delve deep into complex datasets, extract meaningful insights, and generate accurate statistical models. The robustness of SAS lies in its ability to handle extensive data volumes, perform intricate calculations with precision, and visualize data trends effectively. Despite its complexity, robust data analysis in SAS provides users with a comprehensive toolkit for data exploration and decision support, thereby bolstering the analytical prowess of organizations.
Versatility and Flexibility
Adaptability to Various Industries
SAS's adaptability to various industries underscores its versatility and flexibility in catering to diverse analytical needs. By seamlessly integrating with different industry-specific data formats and requirements, SAS proves to be a versatile tool that can address varying data challenges across sectors such as finance, healthcare, and retail. This adaptability feature makes SAS a preferred choice for organizations looking to customize their analytics processes according to specific industry demands.
Customization Options
Moreover, the customization options embedded within SAS further amplify its flexibility in accommodating unique analytical requirements. The ability to tailor statistical models, data visualization tools, and reporting formats to suit specific business objectives distinguishes SAS as a highly customizable analytics platform. This customization feature not only enhances user experience but also empowers organizations to align their analytics strategies with their overarching goals.
Cost-Effectiveness
Savings in Time and Resources
One of the primary advantages of utilizing SAS lies in the significant savings in time and resources that it offers. By automating repetitive tasks, optimizing data processes, and accelerating analytical workflows, SAS enables organizations to streamline operations and maximize resource utilization effectively. This time and resource efficiency translates into cost savings and improved productivity, making SAS a cost-effective solution for data-intensive activities.
Long-Term Value
The long-term value proposition of SAS extends beyond immediate cost considerations to encompass sustained business impact and strategic advantages. Organizations that invest in SAS not only benefit from its immediate efficiencies but also unlock long-term value through enhanced decision-making, data-driven insights, and competitive edge. The enduring value of SAS lies in its adaptability to evolving analytics trends and its capability to future-proof organizations against data challenges, positioning it as a viable long-term investment in analytical excellence.
Challenges and Limitations of SAS
In this section of our comprehensive guide on Understanding SAS in Analytics, we delve into the crucial aspects of Challenges and Limitations of SAS. Understanding the challenges posed by SAS is imperative for software developers, IT professionals, and students interested in analytics. By exploring these limitations, we gain a clearer perspective on how SAS functions in real-world applications and its constraints within complex data environments.
Complexity and Learning Curve
Training and Skill Requirements
Training and skill requirements play a pivotal role in mastering the intricacies of SAS. The complexity of SAS necessitates a detailed understanding of statistical modeling, data management, and graphical representation. Professionals embarking on SAS utilization must undergo comprehensive training to navigate its extensive functionalities effectively. The demand for individuals with proficiency in SAS has surged, making skill acquisition in this area a valuable asset in the competitive landscape of analytics. While the learning curve may be steep, the expertise gained in training and skill development enables enhanced data analysis capabilities, setting individuals apart in the field of analytics.
Integration Challenges
Integrating SAS with existing tools and systems poses a challenge due to its unique data processing methods. The integration process requires a deep understanding of data structures and formats to ensure seamless flow of information between different platforms. Overcoming integration challenges demands meticulous planning and execution to avoid data loss or misinterpretation. Despite these hurdles, successful integration enhances the overall efficiency and accuracy of analytics processes, making it a worthwhile endeavor for organizations keen on harnessing the power of SAS.
Compatibility Issues
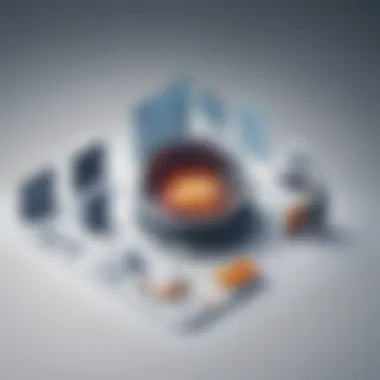
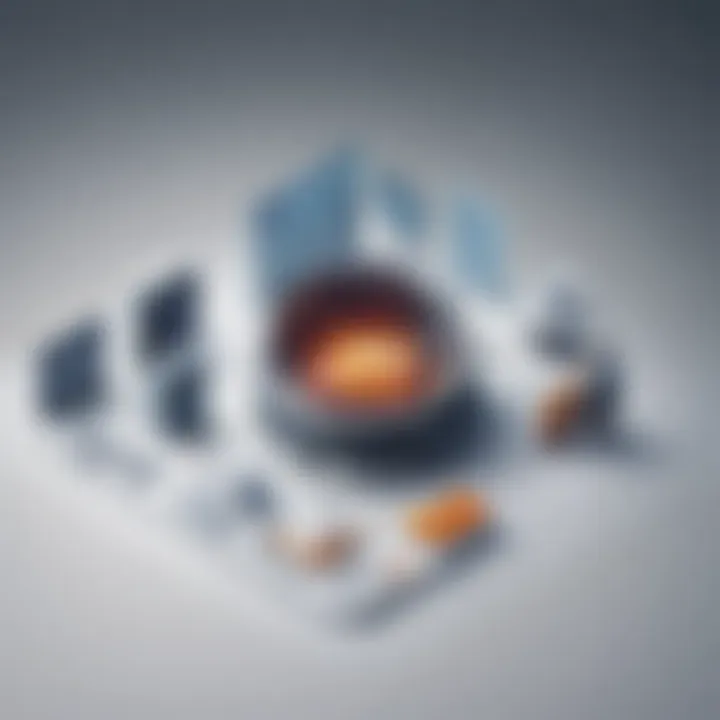
Integration with Other Tools
The compatibility of SAS with other analytics tools is crucial for streamlined data workflows. Incorporating SAS into existing analytics ecosystems requires seamless integration to leverage its full potential. Compatibility with popular tools such as R, Python, and Tableau facilitates comprehensive data analysis and visualization, enhancing the overall analytics process. However, ensuring compatibility demands a thorough understanding of each tool's capabilities and limitations to optimize the collaborative functionalities effectively.
Data Format Support
The compatibility of SAS with diverse data formats influences its usability across different data sources. Supporting various data formats such as CSV, Excel, and SQL databases enhances the versatility of SAS in handling heterogeneous datasets. However, challenges may arise in managing non-standard data formats, necessitating additional preprocessing steps for data normalization. Despite these challenges, the robust data format support of SAS elevates its capabilities in data processing and analysis, making it a preferred choice for organizations dealing with diverse data sources.
Cost of Implementation
Licensing and Maintenance Costs
The cost considerations associated with licensing and maintaining SAS impact the feasibility of its implementation. Licensing fees for SAS software and ongoing maintenance costs constitute a significant portion of the overall expenses. Organizations adopting SAS must evaluate the long-term benefits against the financial investment required to acquire and sustain the software. While the costs may seem prohibitive initially, the robust analytical features and comprehensive support provided by SAS justify the expenditure, offering substantial value in return.
Initial Setup Expenses
Setting up SAS infrastructure incurs initial expenses related to hardware requirements, software installation, and configuration. The complexity of SAS deployment necessitates skilled professionals and specialized resources for seamless implementation. While the initial setup expenses may present a financial barrier, organizations can capitalize on the scalability and versatility of SAS for long-term analytics operations. The strategic investment in setting up SAS infrastructure pays dividends in enhanced data analytics capabilities and streamlined decision-making processes, positioning organizations for sustainable growth and success.
Future Trends in SAS Analytics
In the fast-evolving landscape of analytics, keeping abreast of future trends is crucial to stay competitive. The section on Future Trends in SAS Analytics sheds light on the emerging developments shaping the industry. By delving into Integration with AI and Machine Learning, Cloud-Based SAS Solutions, and Enhanced Security Features, this segment offers insights into the progressive aspects steering SAS applications. The discourse aims to illuminate how SAS is adapting to the changing technological milieu, ensuring that readers are well-informed on what lies ahead.
Integration with AI and Machine Learning
Enhanced Predictive Modeling
Enhanced Predictive Modeling signifies a monumental shift in data analysis practices. This innovative approach harnesses the power of AI and Machine Learning algorithms to enhance predictive accuracy. Its key characteristic lies in its ability to detect complex patterns and relationships within datasets, enabling more precise forecasting. This feature is especially beneficial for organizations seeking to optimize decision-making processes through data-driven insights. Despite its advantages, Enhanced Predictive Modeling may pose challenges in terms of interpretability and model transparency, factors that need to be carefully navigated for optimal results in SAS analytics.
Automated Decision Making
Automated Decision Making streamlines operational efficiency by automating routine decision processes. This function allows organizations to expedite workflows and reduce human error, ultimately leading to enhanced productivity. The key characteristic of Automated Decision Making is its ability to process vast amounts of data swiftly, enabling real-time decision-making. This automation proves advantageous for tasks requiring rapid responses and minimizes the margin for human-induced errors. However, the reliance on algorithms for decision-making can present concerns regarding bias and accountability, necessitating stringent oversight in SAS analytics implementations.
Cloud-Based SAS Solutions
Scalability and Accessibility
The integration of Scalability and Accessibility in SAS analytics offers enhanced operational flexibility and resource utilization. This feature enables seamless adaptation to varying workloads by scaling computing resources as needed. Its key characteristic lies in the on-demand provisioning of resources, ensuring optimal performance during peak usage periods. The unique feature of Scalability and Accessibility optimizes cost-efficiency and promotes agile decision-making processes within organizations. Despite its advantages, this aspect may encounter limitations in terms of data security and privacy, warranting robust protocols to safeguard sensitive information.
Collaborative Analytics
Collaborative Analytics fosters teamwork and knowledge sharing within an organization's analytical endeavors. This feature enables multiple users to collaborate on data analysis projects in real-time, stimulating creativity and collective problem-solving. The key characteristic of Collaborative Analytics is its ability to synchronize insights and inputs from diverse stakeholders, enriching the analytical outcomes. The unique feature of Collaborative Analytics promotes transparency and synergistic decision-making processes, catalyzing innovation and efficiency. However, challenges may arise in terms of data governance and version control, necessitating clear guidelines for effective collaboration in SAS analytics settings.
Enhanced Security Features
Data Encryption Standards
Data Encryption Standards fortify data protection measures by encrypting sensitive information, rendering it unintelligible to unauthorized access. This key characteristic ensures data confidentiality and integrity, safeguarding against potential security breaches. The unique feature of Data Encryption Standards lies in its compliance with regulatory requirements and industry standards, reassuring stakeholders of data security adherence. Despite its advantages, implementing Data Encryption Standards may entail performance overhead and complexity in key management, factors that need to be addressed for seamless integration in SAS analytics ecosystems.
Compliance Protocols
Compliance Protocols establish governance frameworks to ensure adherence to legal and regulatory mandates within SAS analytics practices. This pivotal aspect encompasses data handling, processing, and storage protocols to mitigate risks and uphold industry standards. The key characteristic of Compliance Protocols lies in their alignment with data governance best practices, fostering a culture of compliance and accountability. The unique feature of Compliance Protocols lies in their role as enablers of trust and transparency in data-driven decision-making processes, cultivating stakeholder confidence. However, challenges may emerge in terms of complexity and adaptability to evolving regulatory landscapes, necessitating continuous monitoring and updates for sustainable compliance in SAS analytics environments.