Exploring the Pi Survey Sample Test: Methods and Uses
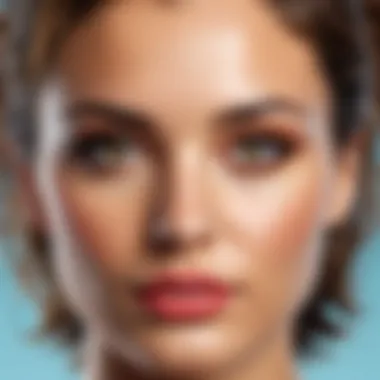
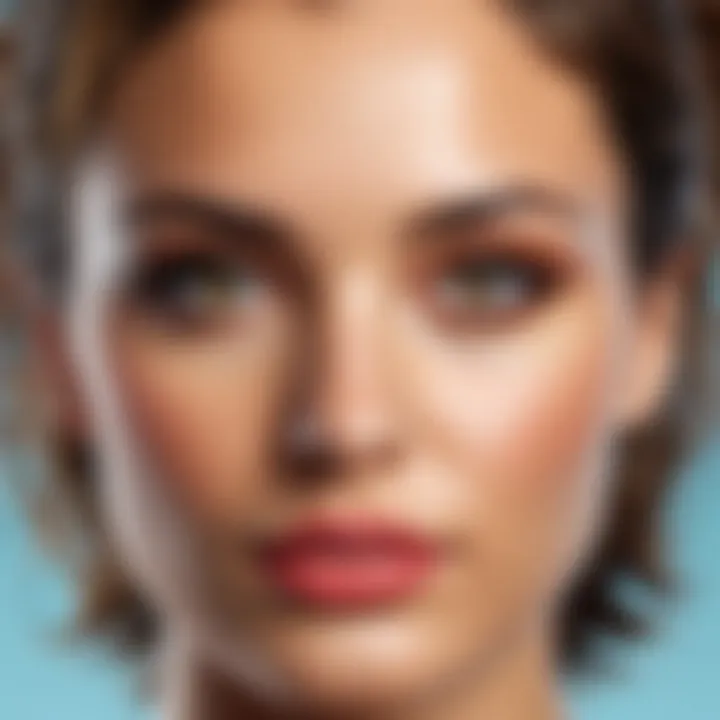
Intro
In today's research landscape, the ability to effectively gather and analyze data is essential. Among the various statistical tools available, the Pi survey sample test holds a vital position. This article delves into the methodologies and applications related to this test, offering both a clear understanding of its mechanics and showcasing practical insights. By unearthing the foundational principles related to its design and sampling strategies, we open a discussion on its use in contemporary data analysis across various fields, including social sciences, market research, and academia.
Software Overview
Features and Functionalities Overview
The Pi survey sample test is a sophisticated tool that facilitates efficient data handling. One key feature includes its customizable survey design, which allows for tailored questions suited to distinct research needs. Moreover, it supports multiple response formats, such as Likert scales and open-ended questions. A built-in data analytics module adds significant value by allowing users to rapidly process and interpret the collected data, providing insights into trends and correlations.
User Interface and Navigation
Ease of use is a pivotal factor in software adoption. The Pi survey sample test boast a clean and intuitive user interface. Users generally find the navigation straightforward, making it accessible for both seasoned researchers and those new to data gathering. The setup process is also streamlined, enabling efficient survey configuration without an extensive learning curve. Gaining insights quickly is also supported through simple data visualization tools, which present findings in a readily comprehensible manner.
Compatibility and Integrations
When discussing software suitability, compatibility is key. The Pi survey sample test operates effectively on a range of devices, including PCs, tablets, and smartphones. Furthermore, it can integrate with popular data storage solutions such as Google Drive and Dropbox. This flexibility enhances accessibility, allowing users to work seamlessly across different platforms.
Pros and Cons
Strengths
The primary advantages of the Pi survey sample test stem from its adaptability and robust analytics capabilities. Its customizable nature means it can cater to various research needs while maintaining high-quality output. Also, the analytics modules inform decision-making through actionable insights derived from complex data aggregation.
Weaknesses
Despite its strengths, the Pi survey sample test does have limitations. Some users may find the extensive options overwhelming, especially if they desire a simple data collection method. Moreover, the analytics can be complex for those not proficient in statistical methodologies, possibly leading to misinterpretations.
Comparison with Similar Software
In contrast to alternatives such as SurveyMonkey and Qualtrics, the Pi survey sample test offers a more granular approach to data collection and processing. While SurveyMonkey focuses heavily on ease of use for basic surveys, the Pi survey sample test’s strengths lie in advanced analytics. Qualtrics, on the other hand, provides extensive customization options but may lack the same level of pragmatic guidance in survey design that the Pi survey sample test offers.
Pricing and Plans
Subscription Options
The Flexible pricing plans suit various budgets and project scopes. There are options for individual users, teams, and larger organizations. Each plan differs in the number of surveys allowed per month and additional features like automated insights.
Free Trial or Demo Availability
A free trial is available for new users, giving them a chance to test the software’s capabilities without risk. This opportunity enables potential users to evaluate the tool thoroughly before making a financial commitment.
Value for Money
Considering its features and functionalities, many users find the Pi survey sample test to offer good value for money. The balance of versatility in survey design and depth of analytics generally align with or exceed user expectations within similar priced tools.
Expert Verdict
Final Thoughts and Recommendations
Overall, the Pi survey sample test proves to be a viable tool for those looking to enhance their data collection strategies. Its blend of design flexibility and analytics provides a strong framework for gathering and interpreting data. An informed decision is best, supported by its robust features.
Target Audience Suitability
This software is most appealing to researchers, data analysts, and businesses needing efficient, customizable survey tools. Those in academic settings can benefit immensely from its analytical capabilities, while businesses can also tailor findings to optimize their market strategies.
Potential for Future Updates
As with all technology, consistent updates will be necessary. Upcoming improvements might focus on enhancing user support and further simplifying advanced analytics to accommodate a broader audience. Overall, the Pi survey sample test has potential to adapt and refine according to emerging data trends and user feedback.
Intro to the Pi Survey Sample Test
The Pi Survey Sample Test holds significant weight in data collection and analysis across various fields. Understanding this test provides professionals and scholars with valuable insights into methodologies that are particularly relevant in today's information age. With the increasing volume of data, the efficiency and accuracy of survey methodologies can impact research outcomes and decision-making processes significantly.
In this section, we will delve into the definition and historical context of the Pi Survey Sample Test. Each of these subsections plays a crucial role in grasping what's behind the application and interpretation of this statistical tool.
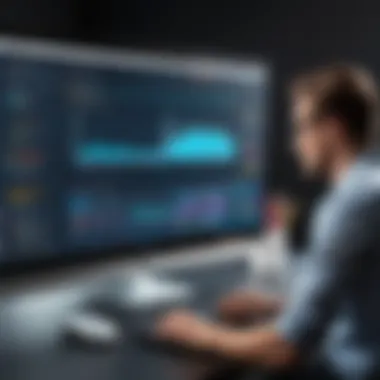
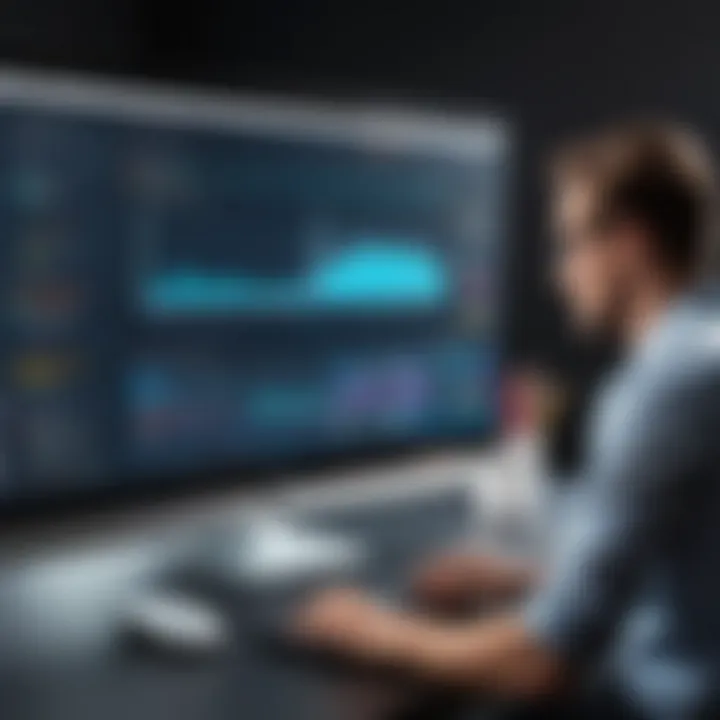
Definition and Purpose
The Pi Survey Sample Test refers to a systematic approach used to gather information from a subset, or sample, of a larger population. Its purpose is multifaceted, as it aids researchers and analysts in drawing valid inferences about a population based on limited data collection. This is vital, especially when assessing time-consuming or resource-intensive scenarios without the necessity of studying every individual in a population.
Employing the Pi Survey Sample Test can facilitate numerous aspects of research, including survey designing, extracting information, and analyzing results. It serves as a foundation for decision-makers to validate trends and support hypotheses regarding different behaviors and attitudes.
Historical Context
The development of survey sampling techniques can be traced back to the early 20th century. The evolution of statistics as a discipline, heavily influenced the design of survey methodologies. Notably, pioneers in statistics, such as Sir Ronald Fisher and Karl Pearson, laid the groundwork for many statistical methods still in use today.
Over decades, the Pi Survey Sample Test adapted to incorporate technological advancements. For example, the advent of computer technology allowed for more complex sampling strategies and better data analysis tools. As research started becoming increasingly sophisticated, so too did the Pi Survey Sample Test, reflecting the new demands of both social and business research environments. Now, it represents a sophisticated yet accessible method of producing data, supported by statistical software tools like R and SPSS.
The consolidation of these ideas signifies a critical turning point in survey testing. It illustrates how historical shifts in research methodologies connected with statistical fundamentals have contributed to the nuanced understanding we have today in engaging with the Pi Survey Sample Test.
Theoretical Framework
Understanding the theoretical framework in the context of the Pi Survey Sample Test is essential. It serves as the backbone that supports the methodologies employed in data collection and analysis. This framework encompasses various statistical principles that ensure findings maintain validity, reliability, and relevance.
Statistical Foundations
The Pi Survey Sample Test relies on robust statistical foundations. These foundations include probability theory and estimation techniques, which are fundamental to drawing conclusions from sampled data. In essence, probability theory informs how likely it is to observe specific data patterns. This relevance is crucial for understanding a population from a manageable sample size. By employing techniques such as random sampling, researchers minimize bias, enhancing the accuracy of their results.
Central to the use of statistical foundations is the role of descriptive and inferential statistics. Descriptive statistics summarize data and provide a clear picture of the surveyed population. In contrast, inferential statistics allow researchers to draw broader inferences about a population based on sample data. This balance between describing data and making predictions underlines the strength of the Pi Survey Sample Test.
Assumptions of the Pi Test
For the Pi Survey Sample Test to yield valid results, several assumptions must hold true. Understanding these assumptions affects both study design and interpretation of results. Key assumptions include:
- Random Sampling: Each member of the target population should have an equal chance of being selected, ensuring that the sample represents the broader group effectively.
- Independence: Responses from participants must be independent of one another. This assumption helps to guarantee that the data collected is not confounded by external variables.
- Normality: While the sample test can work with other distributions, many statistical techniques perform best when the data approximates normal distribution.
Considering these assumptions early in the research design aids in the construction of better questionnaires and sampling methods. Failing to adhere to these principles could result in systemic errors, distorting findings.
In summary, the theoretical framework forms the groundwork of the Pi Survey Sample Test, driving methodologies that support accurate data analysis. By firmly understanding statistical foundations and the underlying assumptions, researchers restart work with a strong evidence base, significantly affecting the credibility of their studies.
Understanding the assumptions of the Pi Survey Sample Test is critical to ensure the relevance and accuracy of the research outcomes.
These considerations not only bolster statistical rigor but help underline the method's value across various application areas.
Designing the Pi Survey Sample Test
The design of the Pi Survey Sample Test is fundamental to ensure that the outcomes derived from it are robust and meaningful. A well-constructed survey is not merely about asking questions; it encompasses careful planning across several key dimensions. By focusing on designs that are rigorous and thoughtful, researchers can gather more reliable data that can substantently influence their analyses and conclusions.
The following elements highlight the importance of effectively designing the Pi Survey Sample Test, addressing the specific considerations that accompany each aspect:
Sampling Methods
There exist multiple sampling methods, each with characteristics that render them suitable for different research scenarios. Comprehensive understanding of these methods can foster sound decisions, affecting the reliability of the data acquired.
- Random Sampling: This method ensures each participant in the target population has an equal chance of selection, promoting objectivity.
- Systematic Sampling: This involves selecting every nth individual. It places a structured formula on sampling.
- Stratified Sampling: This divides the population into distinct subgroups and samples proportionately, addressing any potential variances within those subgroups.
Utilizing the appropriate sampling method can help mitigate errors in data collection, making findings more representational of the entire population.
Target Population Identification
Identifying the target population is imperative. It reflects who the study aims to represent and influences the depth of the findings. Failure to precisely delineate the group can result in sampling bias, leading to questionable generalizations.
Effective strategies for identifying the target population include:
- Defining demographic characteristics like age, gender, and location.
- Utilizing pre-existing databases or registries incentivized with rigorous statistical testing.
- Considering practicalities involved in outreach and engagement with the target group during data collection.
An accurate target population needs careful consideration aligned with the study's objectives, as it can significantly influence the validity of outcomes.
Questionnaire Development
The development of the questionnaire is another significant component in designing a Pi Survey Sample Test. Well-crafted questions can explore the intricacies of respondents’ thoughts, beliefs, and behaviors. Invalid survey questions can lead to misunderstanding and misrepresentation of data.
When constructing a questionnaire, it would be prudent to focus on:
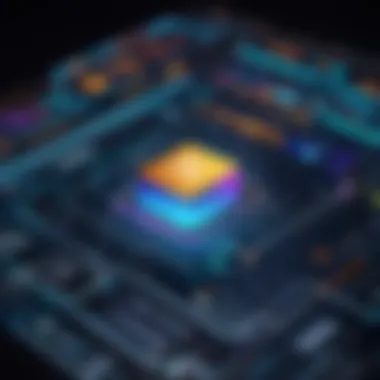
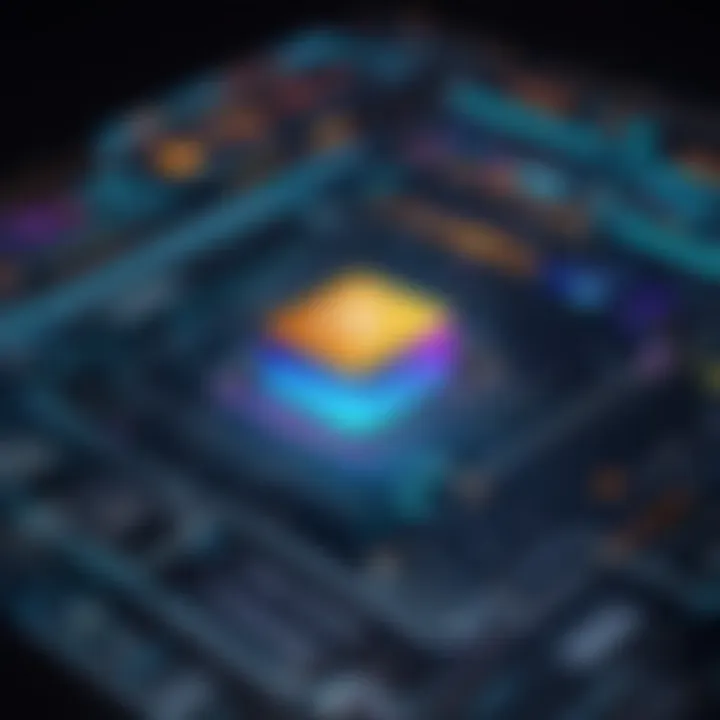
- Clarity and Conciseness: The questions should be straightforward, avoiding unnecessarily complex jargon to aid understanding.
- Question Type: Deciding between open-ended questions that encourage detailed responses or closed-ended questions that streamline data analysis.
- Pilot Testing: Running a pilot test can help assess effectiveness and reveal areas needing adjustment before full-scale administration of the survey.
A structured questionnaire holds weight in enhancing response rates and fidelity of responses.
“The careful design of each component in the Pi Survey Sample Test directly impacts both the quality of the survey results and the credibility associated with it.”
Conducting the Pi Survey
Conducting the Pi Survey is a critical component of this statistical toolt. This phase impacts both the quality and reliability of the data collected. Thus, it receives careful attention from researchers. Effective survey conduct allows for precise data generation necessary for making informed decisions across various fields.
Data Collection Techniques
The methods of how data is collected are foundational in conducting the Pi Survey. Different techniques can influence both the volume and quality of the data gathered. Common datà collection methods include surveys, interviews, observations, and experiments. Each technique has its advantages.
- Surveys allow for large sample sizes, making it possible to gather data from diverse populations quickly. They are cost-effective and can be distributed easily, particularly with the advent of online platforms.
- Interviews provide more in-depth insights into participant thoughts and perspectives. This qualitative method allows a researcher to customize questions based on responses.
- Observations can yield valuable information as they consider actual behaviors rather than reported behaviors.
- Experiments help to track causal relationships through controlled studies.
Choosing the best technique depends on the survey's goals and objectives. Survey creators must carefully consider what is most suitable for capturing precise information while minimizing potential responses biases.
Role of Technology in Data Capture
Technology plays an essential role in collecting and managing survey data. The impact is seen in beautifully designed data gathering tools as well as analytics software. Tech integration enhances accuracy, efficiency, and real-time data collection. In the digital age, tools such as Google Forms, SurveyMonkey, and Qualtrics streamline gathering inputs.
- Online survey platforms offer specialized templates and survey logic to capture respondents creatively.
- Mobile applications facilitate data collection, allowing researchers to gather data in the field without needing physical forms and maintaining efficiency.
- Data management systems, like Excel or SPSS, help in processing complex data for analysis.
Automated data capture reduces human error, fosters data integrity, and enhances the overall participant experience. With that, privacy and ethical protocols also play a significant role, especially when dealing with personal data.
Utilization of modern technology in data capture improves both the accuracy and efficiency of data collected, an important factor in the credibility of the Pi Survey.
Incorporating both appropriate data collection techniques and technology facilitates the successful execution of the Pi Survey. A strategic approach in these areas enables researchers to attain valid outcomes and interpret findings with confidence.
Data Analysis in Pi Survey Testing
Data analysis is a fundamental aspect of the Pi Survey Sample Test. This phase determines how the collected data is processed and how insightful conclusions can be drawn from it. Through systematic evaluation, researchers gain understanding as it affects decision-making and policy formulations in diverse contexts, like academia, healthcare, and business analytics.
In this section, we will explore the methodologies used in data analysis for the Pi Survey Sample Test, the statistical tools involved, and the interpretation of the results that may impact future research.
Statistical Tools and Software
In data analysis, choosing the right tools is essential. Various statistical software options facilitate complex data manipulation, making it easier to conduct meaningful analysis. Commonly employed statistical tools include:
- R: An open-source programming language specialized for statistical computing and graphics. Its extensive library aids in diverse data analysis tasks.
- SPSS: A software package commonly used in social science for statistical analysis. It allows both novices and experts to perform complex analyses easily.
- Python: Particularly popular among data scientists, Python supports libraries like Pandas and SciPy for data manipulation and analysis, alongside other machine learning applications.
These tools are designed to handle large datasets effectively and to perform various statistical tests, such as regression analysis and ANOVA. Their capacity to generate visualizations helps clarify insights, enhancing presentation for stakeholders and contributing to better explanatory frameworks.
Interpreting Results
After analysis, interpreting results is a strategic process. It involves converting numerical outputs into actionable insights that reflect on the question posed at the beginning of the survey. Several considerations are crucial here:
- Statistical Significance: Understanding whether results support hypothesized trends or create new theories.
- Context: Delivery of insights in relation to the initial goals. Researchers must consider surrounding circumstances that may have influenced behavior.
- Practical Implications: Highlighting how findings could influence real-world applications, benefiting organizations and communities.
Moreover, presenting results clearly is paramount. Well-structured tables, charts, and graphs should accompany verbal explanations. This enhances clarity and exhibits thorough investigation behind findings.
Applications of the Pi Survey Sample Test
The applications of the Pi Survey Sample Test are significant in both academic research and the business sector. Utilizing this effective statistical tool enables researchers and analysts to gather meaningful data efficiently and accurately. The main purpose of applying the Pi Survey is to draw insights about a population based on sample data. Understanding its applications helps in appreciating how this method shapes findings across various fields.
Use in Academic Research
The Pi Survey plays a vital role in academic research. Firstly, it simplifies complex data collection processes which researchers face in diverse studies. Given the rigor required for academic work, researchers need reliable samples that accurately reflect larger populations. This test facilitates gathering pertinent information by applying statistically sound methodologies.
In social sciences, for example, the Pi Survey allows for the analysis of behavioral patterns within specified demographics. Researchers study loyalty trends or educational outcomes based on systematic samples. This gives room for generalsing results to a larger group while maintaining a high focus on precision.
Furthermore, the rigor in study design using the Pi Survey can lead to publishing opportunities in peer-reviewed journals. Such publications often give validity and reliability to conclusions drawn from well-executed surveys. To elaborate, standardized surveys that meet academic criteria enhance credibility.
Implications for Business Analytics
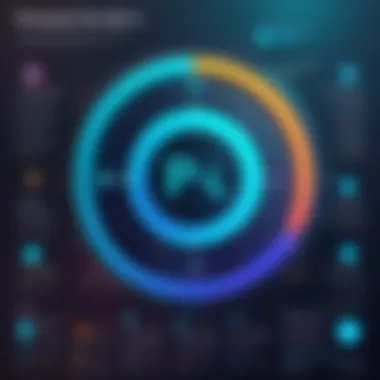
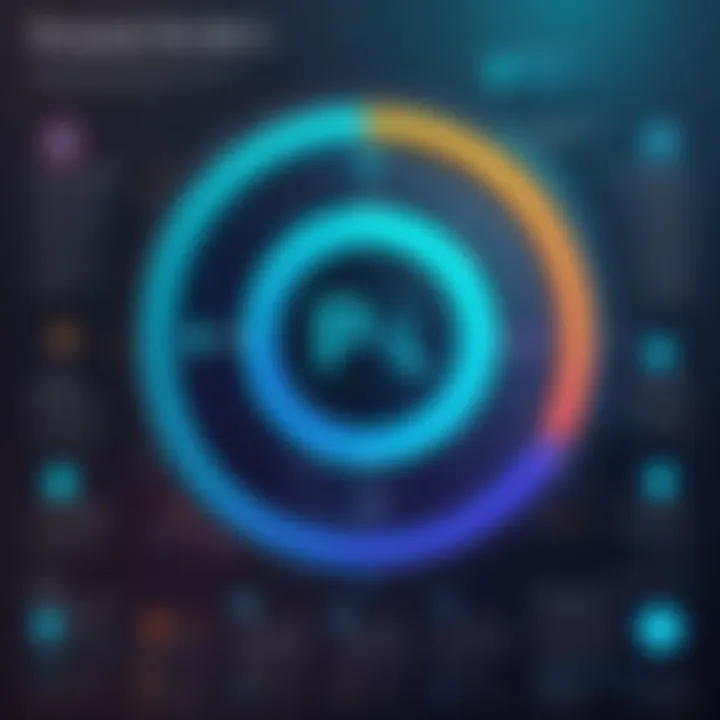
In business analytics, using the Pi Survey carries several implications. Companies rely on data-driven decisions. By implementing this survey method, firms gain vital insights into consumer behaviors, preferences, and trends. This helps in understandng who the target audince is and tailoring marketing strategies effectively.
Moreover, using the Pi Survey enables businesses to tackle issues like product development and customer feedback efficiently. With appropriate design and execution, firms can uncover insights into customer satisfaction. Information obtained can guide improvements for quality and services.
Additionally, data from these surveys serve well for optimizing resources. When companies have precise audience data, budgeting becomes simpler. Investments are made in areas with confirmed value, ensuring less wastage of funds.
The Pi Survey empowers decision-makers by presenting relevant data, dramatically improving outcomes.
Challenges and Limitations
Understanding the challenges and limitations of the Pi Survey Sample Test is imperative for meaningful data interpretation. Each methodological tool comes with inherent weaknesses. Ignoring them can lead to serious miscalculations and incorrect conclusions. High-stakes decisions often lean on these results, making it vital to identify these issues and address them appropriately.
Sampling Bias Issues
Sampling bias is one significant concern when employing the Pi Survey Sample Test. It arises when the sample collection does not accurately represent the target population. This can be due to various factors, including non-random selection, opt-in sampling biases, or over-representation of certain groups. The consequence of sampling bias is considerable; the survey outcomes can be skewed, leading to potentially flawed conclusions.
Consider this: if the survey predominantly includes responses from a specific demographic, the overall findings may misrepresent trends or opinions in a broader context. For analysts and researchers, this emphasizes the necessity for representative sampling. Here are some suggestions to overcome sampling bias:
- Strive for random sampling techniques to enhance the reliability of results.
- Employ stratified sampling to ensure that key subgroups within the population are adequately represented.
- Regularly review and calibrate sampling methods to mitigate unforeseen biases.
Data Integrity Concerns
Equally paramount are data integrity concerns within the context of the Pi Survey Sample Test. Data integrity refers to the accuracy and consistency of data throughout the survey process. If data integrity is compromised, the outcome loses its value. Issues can arise from poor collection methods, human error, or technological malfunctions during data capture. The implications can be dire and echo across various sectors. Misleading data can affect decisions in businesses, governance, and academic research. Moreover, if data is found to be erroneous, it poses another challenge regarding credibility and trustworthiness of results.
“Data isn’t just numbers; it holds the key to better decisions.”
To maintain high levels of data integrity, researchers should consider the following measures:
- Utilize robust data validation techniques during and after data collection.
- Regularly audit collected data to identify and resolve discrepancies promptly.
- Invest in training for personnel involved in data collection and analysis.
These challenges underscore the complexities of using the Pi Survey Sample Test. Despite its efficacy as a statistical tool, a clear awareness of its limitations proves essential for producing reliable, accurate results.
Improving the Pi Survey Sample Test
Improving the Pi Survey Sample Test is crucial for ensuring that it can provide reliable and valid results. This section will explore specific elements that can enhance its effectiveness. A comprehensive understanding of these improvements not only adds value to the test but also helps stakeholders derive meaningful interpretations from the data gathered.
Strategies for Enhanced Accuracy
- Refining Sampling Techniques
Choosing the right sampling method is fundamental. Employing stratified sampling can offer more precise estimates as it divides the's entire population into subgroups. This can better represent the diversity within the population surveyed. - Pre-testing the Survey Instruments
Conducting pilot tests before full-scale implementation allows researchers to identify potential issues. Feedback from test respondents can shed light on confusing questions or flawed measurement Tmethods. - Addressing Non-Response Bias
Non-responses can lead to skewed data. Developing follow-up protocols to encourage responses is vital. approaches like offering incentives or simplifying the response process may yield better participant engagement. - Utilizing Advanced Analytical Techniques
Leveraging machine learning and predictive analytics in data analysis can uncover deeper insights. These innovative methods could identify patterns that traditional methods might overlook. - Implementing Quality Control Measures
Establishing guidelines for data entry and processing mitigates human error. Regular audits of captured data ensure that the quality remains high throughout the research process.
Future Trends in Survey Sampling
As technology continues to evolve, so do the methodologies surrounding survey sampling. Here are several anticipated trends scaling from now to the future:
- Integration of Artificial Intelligence
AI is set to transform data collection and analysis. By harnessing natural language processing, surveys can more effectively address open-ended questions and distill sentiments swings through larger data. - Mobile and Remote Data Collection
The move towards mobile survey convenience is rising. Tools and applications tailored for mobile surveys gain traction, enabling researchers toThe reach a wider and more diverse participant base. - Real-time Data Analysis Using Cloud Technologies
With cloud-based tools, researchers can conduct analyses concurrently with data collection. This enables quick adjustments and refined strategies based on immediate feedback. - Focus on Privacy and Data Ethics
Future developments must contend with rising data privacy concerns. Establishing strict protocols and ensuring participant informed consent will build trust and uphold ethical research norms.
Adopting these strategies and trends will create an ongoing process crucial for enhancing the Pi Survey Sample Test's effectiveness, thereby making a robust analysis of any research undertaking possible.
It is essential to emphasize ongoing improvement in survey methodologies as they lay the groundwork for reliable data analysis and interpretation.
This targeted approach supports professionals and students in comprehending the layered intricacies of survey sampling tests, promoting a culture of continuous calibration and development.
Finale
The conclusion serves as a critical decision point for any analysis, pulling together insights gained through exploring the Pi Survey Sample Test. It encapsulates the essence of the entire discussion and emphasizes the practical and theoretical ramifications of the methodologies presented.
This article highlighted the importance of understanding survey methodologies in both academic and business contexts. A key element is recognizing the diverse applications of the Pi survey sample test as a tool in data collection. Understanding its design and methodology not only equips practitioners with a framework for efficient problem-solving but also enhances the validity of their findings.
Summary of Findings
As we conclude, several findings become apparent:
- The Pi survey sample test provides a structured approach to data collection.
- Sampling methods must align with the target population for accurate analysis.
- Data integrity is crucial, which requires conscientious efforts in the implementation of data collection strategies.
- Limitations exist, yet they serve as areas for improvement and refinement of survey processes.
A review of these elements underscores how robust survey methodologies contribute to significant conclusions, allowing for reliable insights that foster informed decision-making. The systemic understanding of each phase prepares researchers and business professionals alike to adapt and make further gains in their fields.
Call for Further Research
While the existing conversation illuminates numerous aspects of the Pi survey sample test, there remain unexplored territories. Future research should be directed at:
- Enhancing Methodologies: Investigating new approaches within the framework to address current limitations such as sampling bias or data accuracy when using digital platforms.
- Field Applications: Exploring real-world case studies that demonstrate the effectiveness of the Pi survey in various sectors beyond academia.
- Technological Integration: Analyzing how emerging technologies can refine data collection processes, especially with big data dynamics.
Moreover, continual exploration into these areas will foster innovations that directly influence how data-driven decisions are made in the future. Therefore, the call to pursue detailed studies is clear and crucial for the advancement of both theory and practice in the landscape of survey sampling.

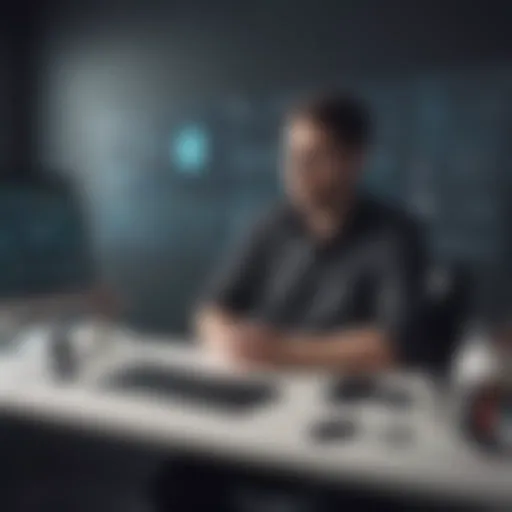