Unveiling the Significance of IBM Hybrid Data Management in Modern Technology
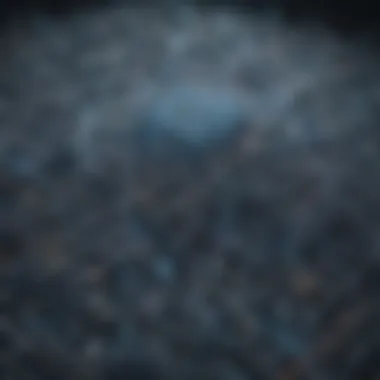
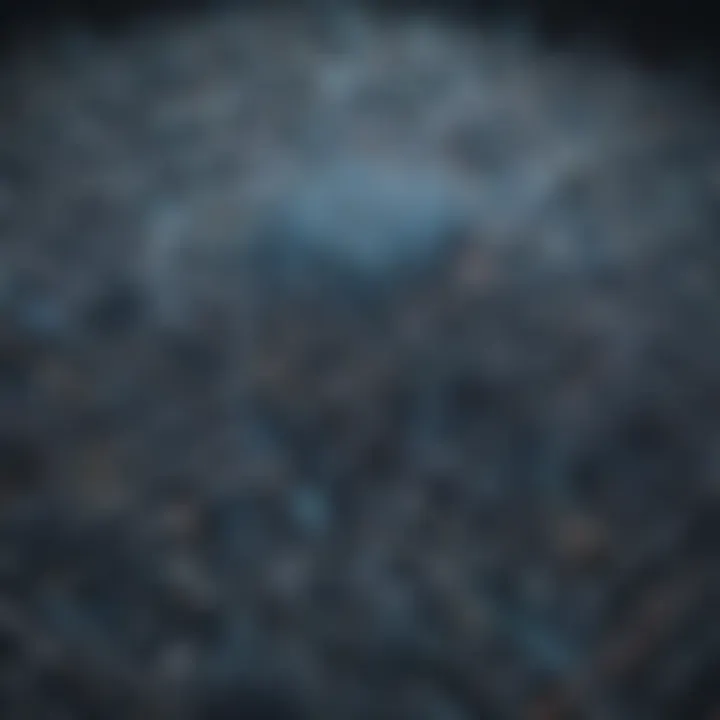
Software Overview
Pros and Cons
Delving deeper into the intricate layers of IBM Hybrid Data Management, it is essential to dissect its strengths and weaknesses. The software boasts an array of strengths, including its robust data integration capabilities, enhanced data security protocols, and scalable architecture. However, it does come with certain limitations, such as the potential complexity in setup and configuration. In comparison with similar software products, IBM Hybrid Data Management stands out for its unmatched data security measures and seamless scalability.
Pricing and Plans
Within the realm of IBM Hybrid Data Management lies a spectrum of pricing and plans to cater to diverse user needs. Subscription options vary, offering flexibility based on the scale of usage and specific requirements. Users can opt for different plans tailored to their individual or organizational needs. Free trial or demo versions offer users the opportunity to experience the software's capabilities firsthand before committing to a subscription. Assessing the value for money entails evaluating the pricing vis-a-vis the features and functionalities on offer, ensuring a harmonious balance.
Expert Verdict
As we navigate through the intricacies of IBM Hybrid Data Management, the expert verdict encapsulates a culmination of insights and recommendations. The software stands as a stalwart in the realm of data management, offering unparalleled security measures and seamless scalability. target audience suitability is wide-ranging, encompassing software developers, IT professionals, and students seeking to delve into the intricacies of hybrid data management. Speculating on potential future updates opens doors to a realm of possibilities, envisioning enhancements and optimizations to further elevate the software's functionality and user experience.
Introduction to IBM Hybrid Data Management
In this exploration of IBM hybrid data management, we delve into a crucial aspect of data handling in modern tech. Understanding and implementing hybrid data management have become increasingly important in this era of diverse data sources and complex infrastructures. It plays a pivotal role in streamlining processes, improving efficiency, and ensuring optimal data utilization in organizations.
Definition and Scope
Understanding the concept of hybrid data management
Hybrid data management incorporates both traditional on-premise and cloud-based solutions to optimize data storage, processing, and analysis. This approach blends the reliability of on-premise systems with the scalability and flexibility of cloud services, offering a comprehensive data management strategy. Companies benefit from cost-effective data handling, agile data processing, and enhanced data security.
Scope of IBM hybrid data management in tech industry
The tech industry embraces IBM hybrid data management for its ability to adapt to evolving data needs seamlessly. This solution caters to the diverse data requirements of modern organizations, integrating various data sources and formats. Its scope extends to enhancing data accessibility, ensuring compliance with regulations, and facilitating data-driven decision-making processes. However, the integration complexity and potential data silos may pose challenges that need strategic planning.
Evolution of Data Management
Historical perspective on data management
Data management has evolved significantly over the years from manual processes to sophisticated automated systems. Traditional methods were resource-intensive and often limited in scalability, whereas modern data management technologies offer streamlined processes, real-time insights, and data governance capabilities. This evolution underscores the importance of adapting to new data trends and technologies to remain competitive.
Emergence of hybrid approaches
Hybrid approaches have emerged as a response to the limitations of solely on-premise or cloud-based solutions. By combining the strengths of both environments, organizations can leverage the best of both worlds. Hybrid approaches enable seamless data integration, flexibility in resource allocation, and optimization of data workflows. However, managing hybrid infrastructures requires specialized expertise and robust integration frameworks.
Significance in Modern Technology
Impact of hybrid data management on businesses
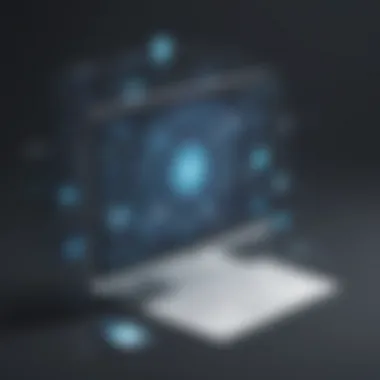
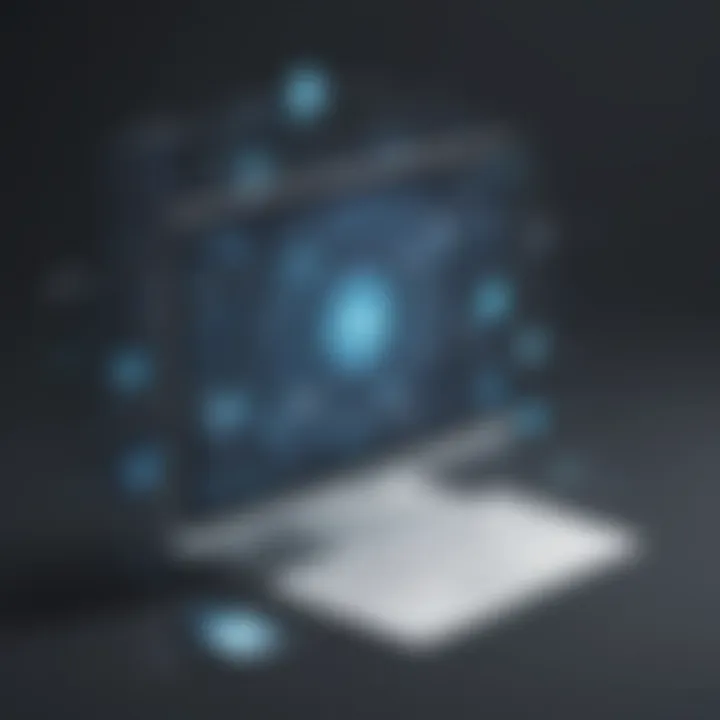
Hybrid data management has revolutionized how businesses handle and utilize their data assets. By incorporating hybrid strategies, organizations can achieve operational efficiencies, improved data quality, and accelerated decision-making processes. The scalability and adaptability of hybrid data solutions make them invaluable in today's dynamic business landscape.
Role in enhancing data security and accessibility
Ensuring data security and accessibility are paramount in the digital age, and hybrid data management plays a critical role in achieving these objectives. By implementing robust security measures and efficient data access controls, organizations can mitigate risks and maintain data integrity. Hybrid data management also enables effective data sharing across disparate systems while safeguarding sensitive information.
Key Components of IBM Hybrid Data Management
In the realm of IBM Hybrid Data Management, understanding the key components is paramount. Data integration solutions play a crucial role in seamlessly combining diverse data sources. By utilizing ETL processes, organizations can ensure a smooth flow of data, enhancing efficiency and accuracy. APIs also contribute significantly by bridging the gap between different systems, enabling seamless communication and integration. These components are vital as they lay the foundation for effective data management and operational success, a pivotal point that this article will delve into with meticulous detail and comprehensive analysis.
Data Integration Solutions
Utilizing ETL processes for seamless data integration
Delving into the intricacies of data integration, the utilization of ETL processes stands out as a cornerstone in the hybrid data management landscape. ETL processes, comprising Extract, Transform, and Load phases, facilitate the extraction of data from various sources, transformation into a usable format, and loading into the target database. The key characteristic of ETL lies in its ability to handle large volumes of data efficiently, ensuring accurate processing and synchronization across systems. This method proves to be a popular choice in modern data environments due to its effectiveness in managing complex data integration tasks with precision and speed.
Role of APIs in connecting diverse data sources
Another pivotal aspect of data integration is the role of Application Programming Interfaces (APIs) in connecting diverse data sources. APIs act as intermediaries, allowing different software applications to communicate and share data seamlessly. The key characteristic of APIs lies in their ability to establish secure and efficient connections between systems, promoting interoperability and data exchange. This feature serves as a beneficial choice for this article as it highlights the role of APIs in enhancing connectivity and data accessibility, regardless of the source or format. While APIs offer enhanced connectivity, they also bring considerations such as security protocols and maintenance challenges that organizations need to address.
Data Governance Strategies
Ensuring compliance and data quality
When it comes to data governance, ensuring compliance and maintaining data quality are paramount. Compliance measures guarantee that data handling practices adhere to regulatory standards and internal policies, reducing the risk of legal implications and data breaches. The key characteristic of compliance-focused data governance is its emphasis on transparency and accountability in data processes. This focus on compliance proves to be a popular choice for this article as it underscores the importance of regulatory adherence and upholding data integrity. However, rigorous compliance measures may bring challenges in terms of complexity and resource allocation that organizations must carefully navigate.
Implementing effective data governance frameworks
In the intricate landscape of data governance, the implementation of effective frameworks plays a crucial role. Establishing comprehensive frameworks ensures that data is managed consistently and securely across the organization. The key characteristic of these frameworks lies in their ability to define roles, responsibilities, and processes for data management, promoting structured governance practices. This strategic approach remains a beneficial choice for this article as it exemplifies the significance of structured frameworks in maintaining data integrity and operational efficiency. Despite its benefits, implementing data governance frameworks may pose challenges in terms of scalability and adaptability to evolving business needs.
Hybrid Cloud Infrastructure
Integration of on-premise and cloud-based resources
Hybrid cloud infrastructure represents a fusion of on-premise and cloud resources, offering organizations a flexible and scalable data management solution. This integration allows businesses to leverage the benefits of both environments, combining the control of on-premise systems with the scalability and accessibility of the cloud. The key characteristic of hybrid cloud infrastructure lies in its ability to optimize resource utilization and accommodate dynamic data requirements. This strategic choice for this article emphasizes the importance of hybrid environments in modern data ecosystems, showcasing the advantages of hybrid cloud models in enhancing data accessibility and scalability. However, integrating on-premise and cloud resources may introduce complexity in data migration and integration processes that organizations need to address proactively.
Scalability and flexibility in data management
Scalability and flexibility are essential aspects of data management in a hybrid environment. Organizations must have the flexibility to scale resources based on varying workloads and requirements, ensuring optimal performance and cost-efficiency. The key characteristic of scalability and flexibility in data management lies in their ability to adapt to changing business needs seamlessly, accommodating fluctuations in data volumes and processing demands. This feature is a crucial factor for this article as it underscores the significance of dynamic resource allocation and operational agility in hybrid data environments. While scalability and flexibility enhance operational efficiency, they also bring considerations such as resource optimization and performance tuning that organizations need to prioritize.
Implementing IBM Hybrid Data Management Solutions
In the intricate web of IBM hybrid data management, the implementation of solutions holds utmost importance. This section meticulously dissects the significance of Implementing IBM Hybrid Data Management Solutions to ensure a seamless data handling process. One cannot underestimate the critical role that implementation plays in the efficacy of hybrid data management strategies. From streamlining workflows to optimizing data access, the robust implementation of IBM solutions is a cornerstone in achieving organizational data management goals.
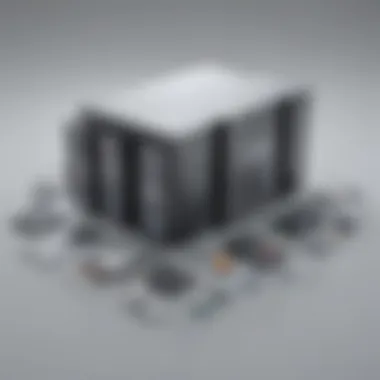
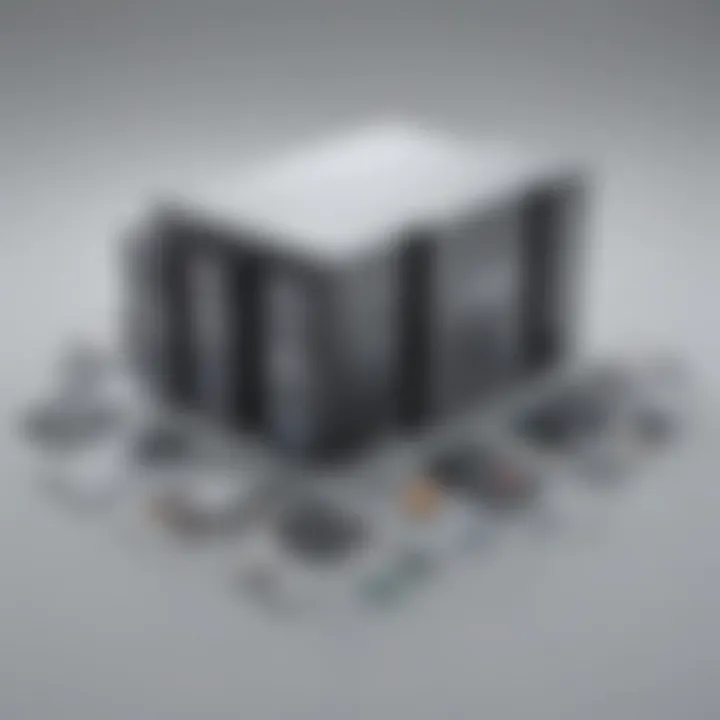
Best Practices
- Adopting a Phased Approach to Implementation: Within the realm of Implementing IBM Hybrid Data Management Solutions, the adoption of a phased approach stands out as a strategic maneuver. This methodical process allows for a structured implementation plan, breaking down complex tasks into manageable stages. The phased approach not only facilitates smoother execution but also enables better tracking of progress and issues, paving the way for effective decision-making. Embracing this structured approach is vital for organizations aiming to navigate the intricacies of hybrid data management successfully.
- Training and Upskilling Employees for Hybrid Environments: A pivotal aspect of Implementing IBM Hybrid Data Management Solutions is the training and upskilling of employees to adapt to hybrid environments. Equipping staff with the necessary skills and knowledge to operate within a hybrid data ecosystem is paramount for the success of data management initiatives. By investing in employee training, organizations can enhance efficiency, foster innovation, and bridge the skills gap in evolving data landscapes. The emphasis on continual learning and development in hybrid environments is key to maximizing the potential of IBM solutions.
Challenges and Solutions
- Addressing Data Security Concerns: When delving into the challenges faced in Implementing IBM Hybrid Data Management Solutions, addressing data security concerns emerges as a critical hurdle to overcome. Ensuring the confidentiality, integrity, and availability of data in a hybrid environment demands robust security measures. By implementing encryption, access controls, and monitoring mechanisms, organizations can fortify their data defenses and mitigate security risks effectively.
- Overcoming Compatibility Issues Between Systems: Another challenge that organizations encounter in Implementing IBM Hybrid Data Management Solutions is navigating compatibility issues between different systems. Integrating diverse data sources, applications, and platforms poses compatibility challenges that can hinder seamless data flow. By leveraging interoperability solutions, standardizing data formats, and conducting thorough compatibility testing, organizations can streamline operations and achieve data harmonization across varied systems.
Case Studies
- Real-world Examples of Successful Hybrid Data Management: Examining real-world examples of successful hybrid data management initiatives sheds light on best practices and proven strategies. By analyzing case studies of organizations that have excelled in integrating on-premise and cloud resources, we can extract valuable insights into successful hybrid data management adoption. These examples serve as pragmatic benchmarks for organizations seeking to optimize their data management frameworks effectively.
- Lessons Learned from Implementation Experiences: Drawing lessons from implementation experiences in hybrid data management environments offers a unique vantage point for growth and improvement. By reflecting on past challenges, successes, and failures, organizations can refine their strategies, enhance their processes, and avoid repeating past mistakes. The iterative nature of learning from implementation experiences is instrumental in fostering continuous improvement and innovation in hybrid data management practices.
Future Trends in IBM Hybrid Data Management
Future Trends in IBM Hybrid Data Management is a pivotal aspect of this article, distinguishing the trajectory of data management strategies in the technological landscape. Understanding the forthcoming trends shapes the foundation for adapting to evolving data environments effectively. It underscores the intrinsic relationship between technological advancements and data management practices in enabling businesses to stay ahead in competitive markets.
AI and Machine Learning Integration
Utilizing AI for predictive analytics in hybrid data environments
The utilization of AI for predictive analytics in hybrid data environments revolutionizes decision-making processes by leveraging machine intelligence to forecast trends and behaviors. This integration enhances data-driven insights, enabling businesses to anticipate market shifts and customer preferences. The predictive capabilities of AI optimize operational efficiencies and strategic planning efforts, providing a competitive edge in dynamic industries.
Key characteristics
AI's ability to analyze vast datasets swiftly and identify patterns empowers organizations to make informed decisions promptly. This rapid analysis facilitates proactive responses to changing market conditions, minimizing risks and maximizing opportunities. The real-time predictive capabilities of AI contribute to agile decision-making, enhancing business agility in responsive environments.
Advantages
The utilization of AI for predictive analytics in hybrid data environments streamlines decision-making processes, enabling proactive interventions before issues escalate. This preemptive approach minimizes operational disruptions and optimizes resource allocation, improving overall operational performance.
Enhancing data processing with machine learning algorithms
Enhancing data processing with machine learning algorithms optimizes data management tasks, increasing efficiency and accuracy in information processing. Machine learning algorithms contribute to automated data analysis, enabling faster insights generation and enhanced data quality. The integration of machine learning enhances data processing speed and scalability, meeting the demands of complex analytical tasks efficiently.
Key characteristics
Machine learning algorithms' adaptability to diverse datasets and evolving patterns ensures continuous improvement in data processing capabilities. The iterative learning process of machine learning enhances algorithm performance over time, adapting to changing data dynamics and improving predictive accuracy.
Advantages
The integration of machine learning algorithms in data processing enhances predictive modeling accuracy and efficiency. This accelerates decision-making processes and improves the quality of insights generated from data analysis. Machine learning algorithms automate repetitive tasks, reducing manual intervention and minimizing the potential for human errors.
Automation and Orchestration
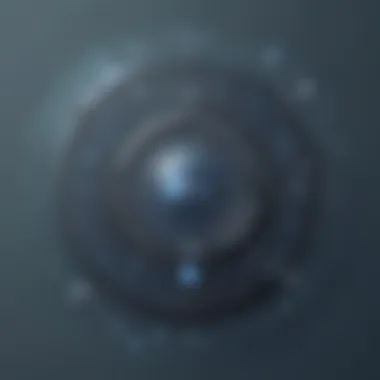
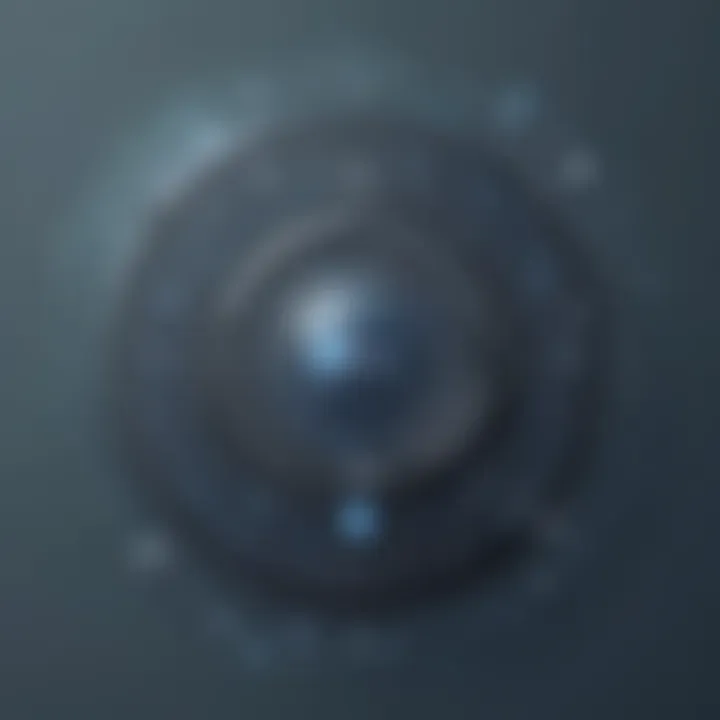
Automating data workflows for efficiency
Automating data workflows for efficiency revolutionizes data management operations by reducing manual interventions and streamlining processes. Automation accelerates data processing, ensuring timely data delivery and enhancing operational productivity. The automated workflow enables data teams to focus on strategic tasks, promoting innovation and agility in data management practices.
Key characteristics
Automation enhances data processing speed and accuracy, minimizing the risk of errors and bottlenecks in data workflows. The seamless integration of automated workflows ensures consistent data quality and integrity throughout the processing chain. Automated data workflows streamline repetitive tasks, optimizing resource utilization and improving operational performance.
Advantages
Automating data workflows for efficiency improves operational efficiency and reduces processing times, enabling organizations to respond rapidly to data demands. The elimination of manual interventions minimizes the potential for human errors, enhancing overall data quality and reliability. Automated workflows promote standardized processes, improving collaboration and ensuring data consistency.
Orchestrating data management tasks across hybrid infrastructure
Orchestrating data management tasks across hybrid infrastructure centralizes data operations, facilitating seamless coordination and data exchange between on-premise and cloud resources. Orchestration optimizes resource allocation, ensuring efficient data processing and scalability in hybrid environments. The centralized management of data tasks enhances operational visibility and control, mitigating risks and enhancing data governance.
Key characteristics
Orchestration simplifies complex data workflows by coordinating diverse data tasks across hybrid infrastructure seamlessly. The centralized orchestration framework enhances agility in adapting to changing data requirements and environments. Orchestrating data management tasks streamlines data operations, improving data accessibility and reliability.
Advantages
Orchestrating data management tasks across hybrid infrastructure enhances operational efficiency and data governance by centralizing control and visibility. The coordinated approach to data tasks minimizes inconsistencies and redundancies, optimizing resource utilization and improving data processing speed. Orchestration promotes synergy between on-premise and cloud resources, enabling seamless data integration and management.
Data Privacy and Compliance
Ensuring adherence to data protection regulations
Ensuring adherence to data protection regulations is paramount in hybrid data strategies to safeguard sensitive information and uphold regulatory compliance. Data protection measures mitigate security risks and ensure the confidentiality of critical data assets. Adhering to data protection regulations promotes trust among stakeholders and enhances organizational credibility in data management practices.
Key characteristics
Adherence to data protection regulations involves implementing secure data storage and transmission protocols to prevent unauthorized access and data breaches. Compliance with regulatory requirements ensures the lawful processing and protection of personal and sensitive data, maintaining data integrity and confidentiality. Data protection measures encompass encryption, access controls, and auditing mechanisms to enforce data security best practices.
Advantages
Ensuring adherence to data protection regulations enhances data security and mitigates the risk of data breaches, safeguarding business-critical information. Compliance with data protection laws instills consumer trust and confidence, fostering positive relationships with stakeholders. Adhering to regulatory mandates protects organizations from legal implications and financial penalties associated with non-compliance, reinforcing data governance and risk management frameworks.
Integrating privacy measures in hybrid data strategies
Integrating privacy measures in hybrid data strategies embeds data privacy principles into data management frameworks, ensuring comprehensive protection of sensitive information. Privacy measures promote transparency in data handling practices and empower individuals to exercise control over their personal data. By integrating privacy measures, organizations uphold ethical data practices, fostering trust and accountability in data management processes.
Key characteristics
Privacy measures include data anonymization, consent management, and privacy impact assessments to evaluate and mitigate privacy risks in data processing activities. By incorporating privacy-enhancing technologies, organizations enhance data protection and regulatory compliance, respecting individual privacy rights and preferences. Integrating privacy measures fosters a privacy-centric culture within the organization, prioritizing data ethics and responsible data handling.
Advantages
Integrating privacy measures in hybrid data strategies enhances data transparency and accountability, fostering trust and compliance with data privacy regulations. By prioritizing data privacy, organizations differentiate themselves as ethical data stewards, building credibility and loyalty among customers. Privacy measures strengthen data governance frameworks and risk management practices, ensuring alignment with evolving privacy laws and regulations in an increasingly data-driven ecosystem.