Exploring Next-Gen Data Management Tools for Enhanced Efficiency
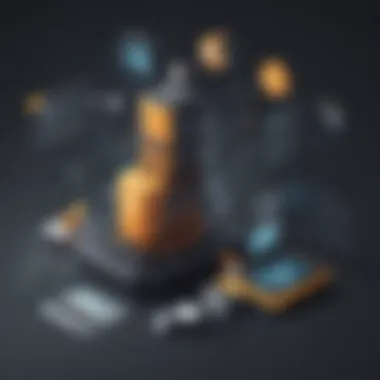
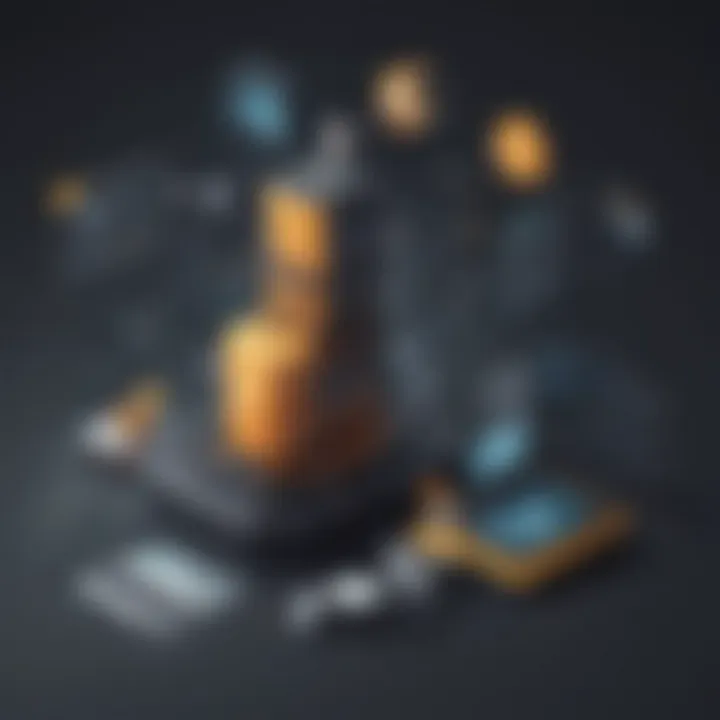
Software Overview
My exploration of alternatives to Hive for efficient data management leads me to analyze the software landscape. In this technological terrain, there exist various tools offering solutions that cater to a myriad of data-related needs. Understanding these options encompass delving into their scalability, performance, usability, and integration capabilities to make informed decisions regarding the appropriate data management solution.
Features and Functionalities Overview
When delving into these Hive alternatives, it is imperative to comprehend the main features that distinguish one from another. These features serve as the backbone of the software, dictating its usability in real-world scenarios.
User Interface and Navigation
A crucial aspect to consider while assessing these alternatives is the ease of use offered by their user interfaces. The way users interact with these tools significantly impacts their overall experience and efficiency in handling data management tasks.
Compatibility and Integrations
Furthermore, exploring compatibility with various devices and other software systems aids in understanding how seamlessly these alternatives can be integrated into existing infrastructures. This compatibility plays a pivotal role in ensuring a smooth transition and operation.
Pros and Cons
Scrutinizing the strengths and weaknesses of each software option is indispensable for a comprehensive evaluation. This in-depth analysis provides a balanced perspective on the advantages and limitations of utilizing these alternatives.
Strengths
Highlighting the advantages and benefits inherent in each software option sheds light on the unique selling propositions that set them apart in the competitive data management market.
Weaknesses
Conversely, addressing the shortcomings and limitations of these alternatives offers a realistic appraisal of the challenges users might encounter in their data management endeavors.
Comparison with Similar Software
Comparing these alternatives to other relevant software products delineates the competitive landscape, aiding users in making well-informed choices based on distinct features and functionalities.
Pricing and Plans
Understanding the pricing models and subscription options available for each software alternative is crucial for aligning financial considerations with organizational needs. Evaluating the value proposition and affordability of these plans is essential for making a cost-effective decision.
Subscription Options
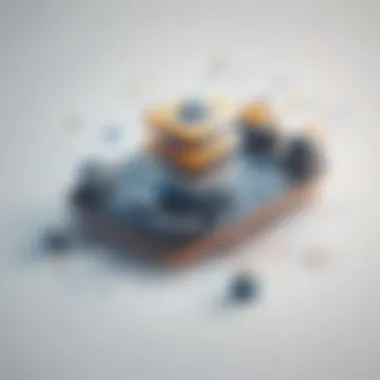
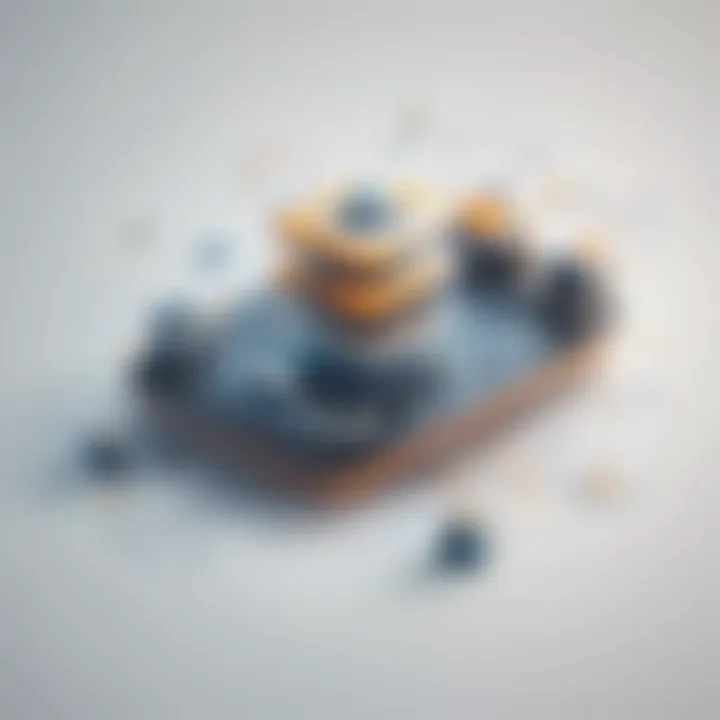
Detailing the various pricing plans unlocks insights into the scalability of these alternatives based on organizational requirements and budget constraints.
Free Trial or Demo Availability
Mentioning the availability of free trials or demo versions allows users to test the waters and experience firsthand the capabilities of these software options before committing.
Value for Money
Assessing whether the pricing aligns with the features offered illuminates the cost-benefit ratio, enabling users to make informed decisions regarding the investment in these alternatives.
Expert Verdict
Providing a final verdict encapsulates the essence of the analysis conducted on these Hive alternatives. Offering recommendations tailored to specific target audiences ensures that users can leverage the right tool for their data management needs.
Final Thoughts and Recommendations
Summarizing the key takeaways and insights, the expert verdict aims to guide users in embracing the software option that best aligns with their operational requirements.
Target Audience Suitability
Suggesting which audience segments would derive the most benefits from utilizing these alternatives tailors the recommendations to specific user groups, enhancing the decision-making process.
Potential for Future Updates
Speculating on possible improvements or updates to these software options predicts the trajectory of innovation and enhancement, preparing users for evolving data management challenges.
In the realm of data management solutions, exploring alternatives to Hive is imperative for organizations seeking enhanced efficiency and performance. While Hive has been a prevalent tool, newer technologies offer different approaches and capabilities to meet evolving data needs. This section serves as a pivotal point in the article, setting the foundation for a comprehensive analysis of alternative platforms. By dissecting the key components of Hive and elucidating its strengths and limitations, readers gain a clear understanding of why exploring alternatives is not only beneficial but necessary for modern data-centric environments.
To begin this exploration, it is crucial to highlight the significance of understanding Hive and its role in the data management landscape. With a focus on elucidating the architecture, functionalities, and use cases of Hive, readers can grasp the fundamental principles that underpin this technology. Moreover, delving into the key features of Hive such as data warehousing, query optimization, and scalability sheds light on its capabilities and limitations. By unpacking these aspects, readers are better equipped to discern the areas where alternative tools may offer distinct advantages.
Furthermore, examining the challenges associated with Hive unveils potential pain points that organizations may encounter when relying solely on this platform. Scalability issues, performance concerns, and complexities in query optimization can hinder operational efficiency and limit the scope of data management capabilities. This critical analysis sets the stage for exploring alternative solutions that address these challenges head-on, propelling organizations towards more streamlined and agile data management processes.
The subsequent sections will venture into alternative tools such as Apache Spark, Presto, and Databricks, offering in-depth insights into their scalability, performance, ease of use, and integration capabilities. By conducting a comparative analysis, readers will gain a holistic perspective on how these alternatives stack up against Hive in various aspects. This thorough examination is pivotal in helping readers make informed decisions when selecting the most suitable data management solution for their specific requirements.
Understanding Hive
In the realm of data management, understanding Hive is crucial for professionals striving for efficient and effective data handling. Hive, as a data warehouse infrastructure built on top of Hadoop, provides a SQL-like interface to manage and query large datasets. By comprehending Hive's architecture and functionality, users can harness its power to streamline data processing tasks, enhance data analysis capabilities, and optimize resource utilization. Understanding Hive also involves grasping its role in the broader ecosystem of big data technologies, its compatibility with various data formats, and its capability to handle complex querying requirements efficiently. This section will delve deep into the significance of mastering Hive for professionals in IT-related fields, software developers, and students seeking in-depth knowledge of data management solutions beyond conventional databases and processing methods.
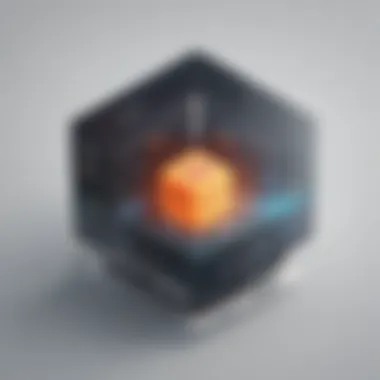
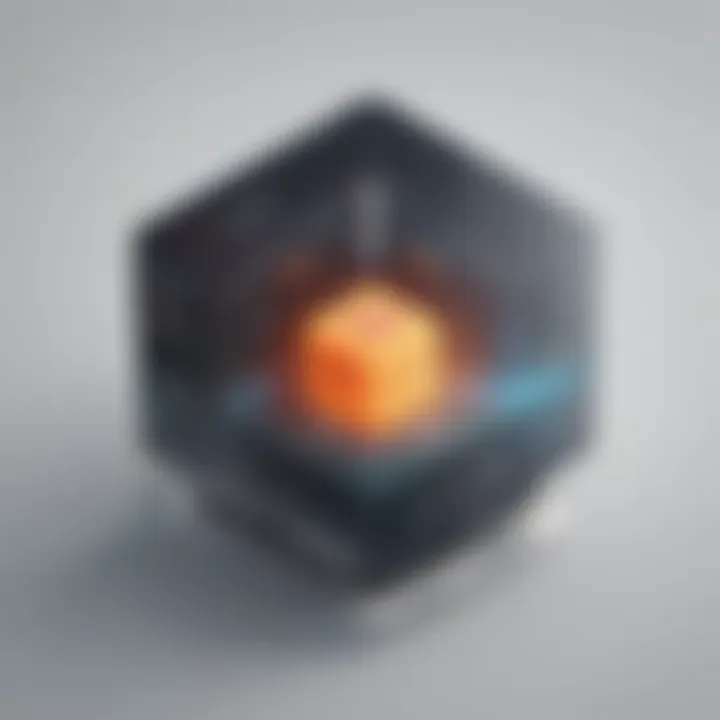
Overview of Hive
To gain a comprehensive perspective on Hive, delving into its overview is essential. Hive operates by translating SQL queries into MapReduce jobs, allowing users to interact with data stored in Hadoop Distributed File System (HDFS) using familiar SQL syntax. This abstraction layer simplifies data access and manipulation, making it easier for users to work with vast amounts of structured and semi-structured data effectively. Understanding Hive's architecture, components such as Metastore, Hive Query Language (HQL), and execution engine, facilitates a holistic grasp of its inner workings and functionalities. By exploring Hive's overview, readers can appreciate its role in facilitating data processing pipelines, supporting data analytics workflows, and enabling complex data transformations with ease.
Key Features of Hive
Analyzing the key features of Hive unveils its capabilities that set it apart as a robust data management tool. Hive offers schema flexibility, allowing users to define schemas on read, offering versatility in handling diverse data types. Its query optimization techniques enhance performance by optimizing data processing workflows and minimizing query execution times. Hive's scalability enables seamless horizontal scaling to accommodate growing data volumes, making it ideal for organizations dealing with expanding datasets. Furthermore, Hive's integration with existing Hadoop ecosystem tools enhances interoperability, enabling seamless data exchange and processing across various platforms. By exploring Hive's key features, readers can identify its strengths in data management, understand its potential drawbacks, and make informed decisions when evaluating alternative data processing solutions.
Challenges with Hive
Scalability Issues
Scalability issues within the context of Hive pose significant obstacles to managing large volumes of data effectively. As organizations grapple with ever-expanding datasets, the ability of a data management tool to scale seamlessly becomes paramount. In the case of Hive, its architecture and design may struggle to cope with the increasing demands of growing data sets, leading to performance bottlenecks and inefficiencies. This section delves into the intricacies of scalability issues in Hive, outlining the implications for data processing capability and infrastructure support. By dissecting these challenges, readers gain insights into the practical limitations that can hinder efficient data management within a scalable framework.
Performance Concerns
Performance concerns are central to the discussion of Hive alternatives, as data processing speed and resource utilization play a pivotal role in decision-making. Within the context of Hive, performance issues may arise due to various factors, including suboptimal query execution speed and inefficient resource utilization. Addressing these concerns is vital for organizations seeking enhanced efficiency and productivity in their data management workflows. By dissecting the nuances of performance issues in Hive, we shed light on the critical aspects of query execution speed and resource utilization. This detailed analysis equips readers with a comprehensive understanding of the performance bottlenecks that Hive may encounter, prompting consideration of alternative tools that offer improved performance capabilities.
Complexity in Query Optimization
Complexity in query optimization adds another layer of intricacy to the challenges posed by Hive in the realm of data management. Query optimization plays a pivotal role in ensuring efficient query processing and timely results retrieval, factors that significantly impact overall system performance. Within the domain of Hive, navigating the complexities of query optimization can be daunting, potentially impeding the seamless execution of queries and leading to subpar performance outcomes. This section dives deep into the maze of query optimization complexities within Hive, highlighting the implications for user interface and query language flexibility. By unraveling these complexities, readers gain a nuanced understanding of the challenges associated with query optimization in Hive, setting the stage for exploring alternative tools that offer streamlined query processing capabilities.
Exploring Alternatives
In the realm of data management solutions, the importance of exploring alternatives beyond Hive cannot be overstated. This section delves into alternative tools that offer unique benefits and address specific considerations in the realm of efficient data management. By examining Apache Spark, Presto, and Databricks, we gain insights into diverse options that cater to a variety of data processing needs, scalability requirements, and performance expectations.
Apache Spark
Apache Spark emerges as a compelling alternative to Hive, leveraging its distributed computing framework to enhance data processing capabilities and deliver high performance. With its in-memory processing capabilities and support for various programming languages, Apache Spark stands out for its ability to handle complex analytics tasks with speed and efficiency. Its seamless integration with other big data tools and frameworks further amplifies its appeal, making it a versatile choice for organizations seeking a robust data management solution.
Presto
Presto offers a unique approach to data processing, focusing on interactive queries and real-time analytics. Known for its query execution speed and high performance, Presto enables users to access and analyze data rapidly, making it ideal for ad-hoc analysis and exploratory data tasks. Its compatibility with multiple data sources and SQL query support enhance its usability, while Presto's distributed SQL query engine ensures efficient parallel processing for improved resource utilization.
Databricks
Databricks introduces a unified analytics platform that combines data engineering and data science capabilities, offering a comprehensive solution for data processing and analysis. By providing a collaborative environment for data teams, Databricks streamlines the process of building and deploying data applications. With features such as automated cluster management and interactive notebooks, Databricks simplifies data workflows and accelerates insights generation, making it a valuable alternative for organizations aiming to optimize their data management practices.
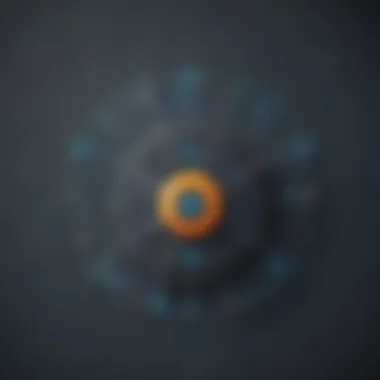
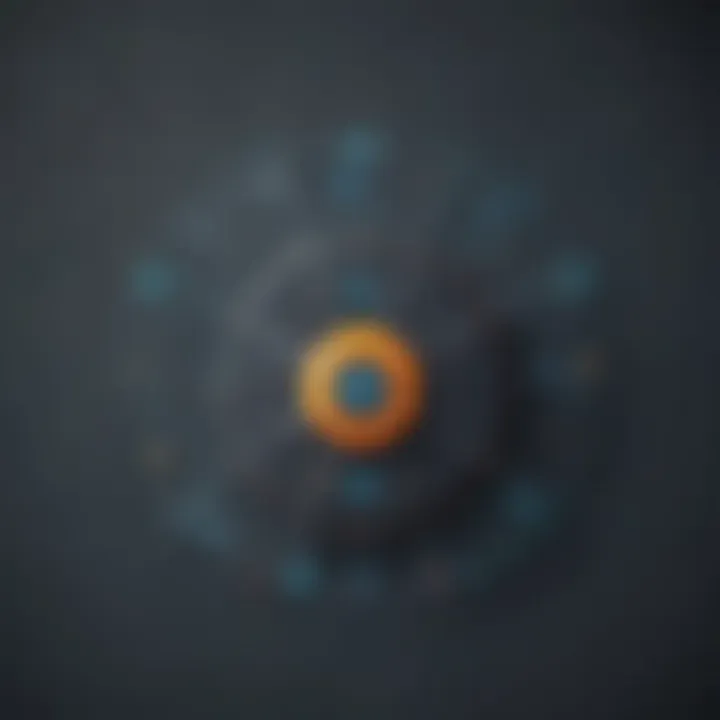
Comparative Analysis
In the realm of data management tools, a Comparative Analysis plays a pivotal role in evaluating alternative solutions to Hive. By dissecting key elements such as scalability, performance, ease of use, and integration capabilities, this analysis equips decision-makers in IT-related fields with invaluable insights. It enables a detailed examination of each tool's strengths and weaknesses, fostering informed decision-making processes. Furthermore, Comparative Analysis serves as a compass for navigating the complex landscape of data management platforms, offering professionals a clear roadmap towards selecting the most suitable solution.
Scalability
Data Processing Capability
Data Processing Capability stands as a crucial facet within the realm of scalable data management solutions. Its adeptness in handling vast volumes of data swiftly and efficiently is instrumental in achieving optimal performance. The key characteristic of Data Processing Capability lies in its ability to streamline complex data processing tasks, thereby enhancing overall productivity. This attribute makes Data Processing Capability a popular choice in modern data management, promising enhanced operational efficiency and streamlined workflows. Despite its advantages, challenges may arise in maintaining synchronization across diverse datasets and ensuring consistent processing speeds in dynamic environments.
Infrastructure Support
Infrastructure Support plays a crucial role in bolstering the scalability of data management solutions. By providing robust support infrastructure, organizations can ensure seamless operation and reliable performance of their chosen platform. The key characteristic of Infrastructure Support lies in its ability to provision resources effectively, catering to varying workload demands with agility. This makes it a beneficial choice for organizations seeking scalable and adaptable data management solutions. However, challenges may stem from resource allocation complexities and the need for efficient resource utilization strategies to manage peak workloads effectively.
Performance
Query Execution Speed
The aspect of Query Execution Speed holds profound importance in the realm of data management platforms. Its capability to execute queries swiftly and deliver results in real-time is essential for meeting critical business requirements. The key characteristic of Query Execution Speed lies in its efficiency in processing complex queries with minimal latency, thereby ensuring timely insights and actionable outcomes. This feature makes Query Execution Speed a preferred choice for organizations relying on prompt data analysis and decision-making processes. Nevertheless, challenges may arise in maintaining consistent query performance across varying datasets and query complexities.
Resource Utilization
Resource Utilization stands as a critical aspect in driving optimal performance within data management solutions. Its ability to allocate and utilize resources efficiently is crucial for maximizing operational efficiency and minimizing wastage. The key characteristic of Resource Utilization lies in its capacity to optimize resource allocation based on workload requirements, thereby enabling cost-effective operations. This attribute makes Resource Utilization a popular choice for organizations aiming to streamline resource management and enhance overall performance. However, challenges may surface in balancing resource allocation across competing tasks and ensuring equitable resource distribution across diverse workloads.
Integration Capabilities
The essence of Integration Capabilities lies in the capacity of a data management tool to harmonize with existing systems, applications, and databases. By possessing robust Integration Capabilities, a data management solution can facilitate the smooth flow of data across various platforms, enabling efficient data exchange and synchronization.
An integral aspect to consider about Integration Capabilities is the extent to which a tool supports diverse data formats, protocols, and APIs. This flexibility is vital for enabling easy data transfers and interactions between different systems, enhancing overall operational efficiency in data processing workflows.
Moreover, the benefits of strong Integration Capabilities extend to enhancing collaboration and integration among teams working on distinct projects or tasks. Seamless integration fosters a conducive environment for sharing data insights, leveraging collaborative efforts, and driving innovation across the organization.
Considering Integration Capabilities in data management solutions is not just about connectivity but also about streamlining processes and minimizing complexities. A tool with robust Integration Capabilities can automate data workflows, reduce manual intervention, and ensure data consistency and accuracy throughout the analytical pipeline.
For professionals in IT-related fields or software developers assessing Hive alternatives, a comprehensive evaluation of Integration Capabilities is essential to determine the tool's adaptability to their existing infrastructure, data sources, and integration requirements.
Conclusion
In this intricate exploration of options beyond Hive for efficient data management, the Conclusion stands as a crucial segment encapsulating the essence of our discourse. By delving into the detailed analysis of various alternatives such as Apache Spark, Presto, and Databricks, we have unraveled a landscape teeming with possibilities and considerations. Each alternative presents unique strengths and nuances that cater to distinct data management needs, ensuring a tailored approach for diverse scenarios.
The Importance of Conclusion lies in its ability to synthesize the plethora of information disseminated throughout this guide. It serves as a compass, guiding readers to navigate through the complexities and intricacies of selecting the optimal data management solution. By encapsulating the salient features, benefits, and drawbacks of each alternative, the Conclusion empowers software developers, IT professionals, and students with the acumen needed to make informed decisions that align with their organizational goals and technological requirements.
Furthermore, the Conclusion elicits a deeper reflection on the evolving landscape of data management tools, signaling a shift towards more versatile, scalable, and performance-driven solutions. As organizations grapple with ever-expanding data sets and complex analytics requirements, the insights gleaned from this exploration become invaluable, shaping the way businesses harness the power of data for strategic decision-making and operational efficiencies.