Understanding Signal AI: Insights into Real-Time Data Intelligence
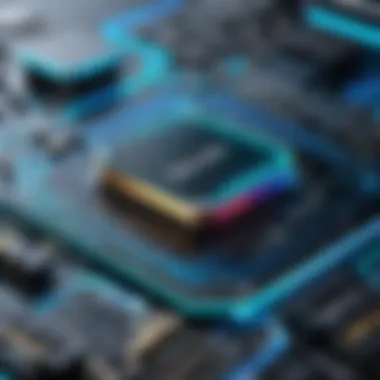
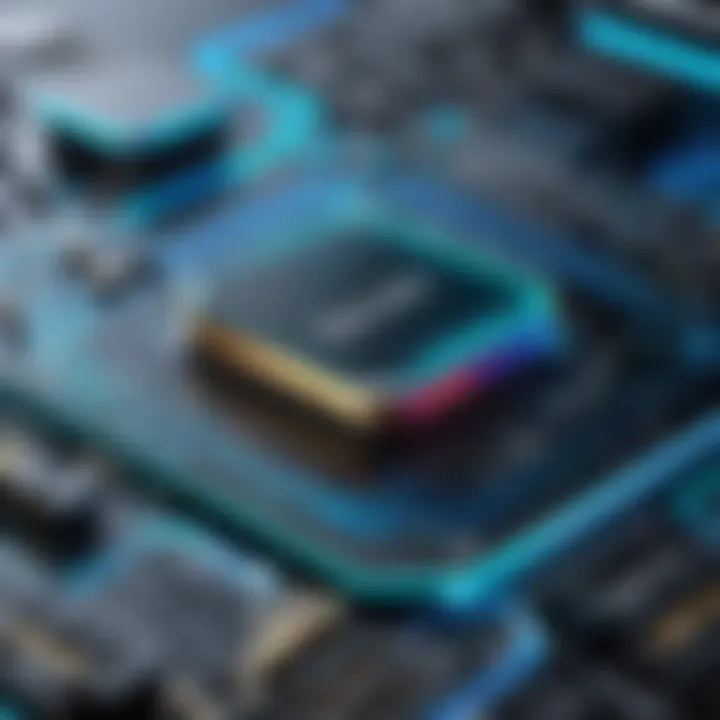
Intro
Signal AI represents a paradigm shift in how organizations utilize artificial intelligence to sift through real-time data signals from diverse sources. The need for rapid decision-making in dynamic business environments has heightened the importance of technology that provides actionable insights. Knowing the basic principles and applications of this technology will enable IT professionals, software developers, and students to unlock its full potential and gain a competitive edge in their respective fields.
Software Overview
Features and functionalities overview
Signal AI has a number of noteworthy features that help address the complexities of data processing and analysis. One of its core functions is the ability to aggregate varied data signals, including news articles, social media activity, and custom data feeds. This amalgamation empowers users to retrieve pertinent information that may influence business strategies. Another critical capability is its real-time analytics feature, which provides instant updates based on emerging trends. Therefore, users are better equipped to respond quickly to shifts in market dynamics.
User interface and navigation
When discussing user experience, Signal AI boasts a clean and intuitive interface. Navigating through the software often involves straightforward operations. Users can efficiently map data sources, configure alerts, and analyze incoming data signals quickly. Whether users are seasoned professionals or newcomers to AI technologies, the user interface seeks to minimize the learning curve while maximizing productivity.
Compatibility and integrations
In the realm of compatibility, Signal AI seamlessly integrates with platforms like Microsoft Power BI and Tableau. These integrations allow users to visualize data directly from within the AI system. Additionally, the platform supports a variety of devices, making it accessible across desktops, tablets, or even smartphones. The interconnectedness of various applications broadens its utility, enabling organizations to derive insights irrespective of their existing tech stacks.
Pros and Cons
Strengths
Signal AI possesses several merits that are immediately recognizable. First, the ability to analyze massive datasets in real time represents a significant advantage. By focusing on relevant signals and omitting noise, the software increases the efficacy of data-driven decisions. Moreover, Signal AI offers custom reporting capabilities tailored to specific user requirements.
Weaknesses
However, Signal AI does come with some limitations. Some users have pointed out that onboarding can take longer than expected due to the broad capabilities of the tool. Furthermore, specific functionalities may be overwhelming for non-technical users.
Comparison with similar software
When contrasting Signal AI to competitors like IBM Watson or Microsoft Azure, its niche specificity stands out. While IBM Watson leans more into comprehensive models incorporating all forms of AI, Signal AI excels at real-time data processing, making it preferable for sectors purely focused on immediate market analysis.
Pricing and Plans
Subscription options
Signal AI typically operates on a subscription-based model, which makes it accessible for diverse budgets. Basic plans provide essential features ideal for small enterprises, while corporate and premium plans unlock advanced software capabilities tailored for large-scale organizations.
Free trial or demo availability
A free trial of Signal AI is often available, permitting prospective users to explore its functionalities ahead of committing to a subscription. This trial period serves as an opportunity for interested users to assess whether platforms align with their specific needs.
Value for money
Considering the advanced features Signal AI offers, many users find that pricing reflects the value delivered through actionable insights. The layer of customization options enhances functionality while justifying the incurred expenses.
Expert Verdict
Final thoughts and recommendations
Given the informative landscape of Signal AI, it becomes clear that this tool stands out in delivering real-time, strategic analysis. Ultimately, whether it fulfills its promise largely depends on user familiarity with leveraging big data.
Target audience suitability
This platform best serves professionals in research and analytics fields, marketing teams, data scientists, and software developers. Its design and capabilities align well with users who require precision in real-time information processing.
Potential for future updates
Speculation regarding future enhancements suggests an increased focus on usability for non-technical users, possibly introducing further automation features. Ongoing adjustments to maintain competitiveness in a rapidly evolving AI landscape will likely influence the next iterations of Signal AI components.
Preamble to Signal AI
The realm of artificial intelligence is rapidly evolving, and Signal AI stands as a notable entity within this landscape. Understanding Signal AI is crucial for professionals, developers, and students engaged in the IT field. This section seeks to illuminate the foundational aspects of Signal AI and highlight its significance as a tool for informed decision-making through data.
Defining Signal AI
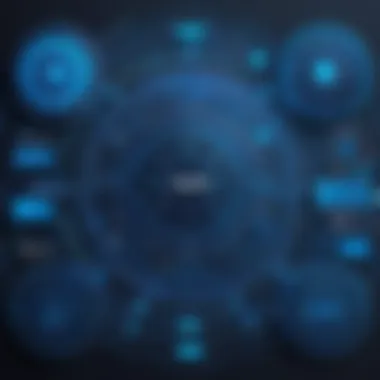
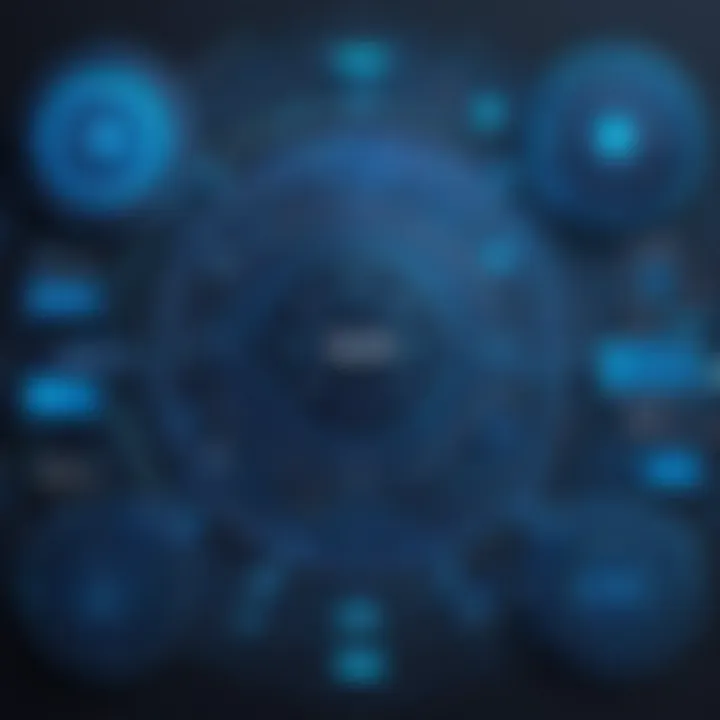
Signal AI can be defined as an advanced artificial intelligence platform that processes and analyzes vast streams of real-time data signals. It utilizes a variety of algorithms to discern patterns and provide actionable insights gleaned from this data. This analysis is not limited to a singular domain; rather, it across sectors such as finance, healthcare, and marketing, enhancing operational efficiency and strategic planning.
The necessity for real-time data is deeply felt in today’s digital era. A traditional approach to data gathering and analysis, often reflecting static scenarios, can fall short in supporting rapid decision-making. Signal AI empowers organizations to pivot rapidly and insights that can adjust strategy.
Historical Context
The evolution of Signal AI roots back to the convergence of high-speed data technology and sophisticated machine learning. In earlier years, businesses relied mainly on rudimentary data analytics that lacked the nuance of deeper contextual understanding. As companies accumulated vast amounts of data, required advanced tools to extract value became evident.
With the rise of big data in the early 21st century, the demand for smarter analytical frameworks increased. This created fertile ground for innovations leading to Signal AI. The transformation was gradual, reflecting changes in how organizations approached data—moving from retrospective analysis to predictive systems designed to provide insights in real-time.
For those across tech industries, grasping the journey of Signal AI sheds light on its potential and ongoing relevance. By recognizing its historical development, experts can establish a clearer view of its capabilities in meeting contemporary challenges.
The Technological Framework
The technological framework of Signal AI is central to its effectiveness in processing and analyzing vast amounts of data. Understanding this framework allows us to appreciate how the platform operates and the variety of applications it supports. The core components and architecture play a significant role in its ability to provide real-time insights and enhance decision-making across numerous industries.
Core Components of Signal AI
Data Processing Layers
Data processing layers are crucial for Signal AI's functionality. They manage how data is ingested, processed, and stored. One key characteristic of these layers is their scalability. This attribute positions them as a prefered choice since they can efficiently handle progressive data influx from different sources.
A unique feature is their modular structure. This allows different layers to specialize and optimize for various data processing tasks. For instance, ETL (Extract, Transform, Load) processes can be distinctly managed within these layers, giving rise to heightened efficiency and faster results.
However, advancements in computational techniques can make these layers complex and may introduce overhead in terms of management. Hence, sound architectural decisions are critical in the design.
Machine Learning Algorithms
Machine learning algorithms form the backbone of Signal AI's analytical capability. These algorithms process data, extracting meaningful patterns and trends that aid organizations in strategic decision making. A remarkable aspect is their adaptability. They can be trained on various datasets, improving accuracy over time, which makes them vital in analyzing diverse signals.
Additionally, the algorithm's ability to learn in real-time is an excellent feature. While they bring smart decision support features, deploying and tuning these models also have challenges. The risk of overfitting is paramount, which requires vigilance to prevent models from becoming too specialized for historical data.
Real-Time Data Integration
Real-time data integration is foundational for Signal AI. This functionality ensures that the platform engages with data as it arrives, optimizing responsiveness. A core characteristic is its speed; rapid processing capabilities make it possible to analyze vast swaths of data almost instantaneously.
What stands out is the integration of disparate sources seamlessly. By pulling from both structured and unstructured datasets, Signal AI outclasses platforms limited to specific data types. Despite this advantage, real-time integration poses challenges with data consistency and potential latency issues, which need to be accounted for in strategy.
Architecture Overview
An assessment of Signal AI’s architecture reveals how its components cohesively operate. The framework is often layered, promoting maintenance and scalability. At its core, it encompasses integrations with various data storage, processing capacities, and API connections. The result is a resilient platform that end-users find efficient.
Considering the increasing demands in AI, Signal AI is equipped to evolve as new tools emerge, making it a top contribution to advancing operational methods across industries. Overall, this technological framework asserts the foundational principles that enhance the value proposition of Signal AI.
The interplay of diverse technological elements empowers Signal AI to derive actionable insights swiftly, shaping decisions across various sectors.
Data Sources Leveraged by Signal AI
The effectiveness of Signal AI significantly depends on the data it utilizes. Hence, data sources leveraged by Signal AI play a crucial role in shaping the insights and conclusions reached through its analysis. The blending of various data inputs enhances the model’s predictions, providing a more nuanced understanding of ongoing trends and patterns. Understanding these data sources offers clarity about their integral role in facilitating efficient decision-making processes. Additionally, the analysis ensures users grasp the benefits of informed, data-driven strategies in their respective domains.
Public Data Repositories
Public data repositories are major assets for Signal AI. These sources encompass a wide range of information freely accessible to anyone. For example, repositories like government databases and academic research institutions publish significant datasets annually, which are transparent and open to the public. They contribute vital information on demographics, economic activity, weather data, and more.
The advantages of utilizing public data repositories include:
- Cost-effectiveness: The data is often free, reducing operational costs compared to paid data.
- Diversity of perspectives: Accessing varied sources lends a more comprehensive viewpoint on trends.
- Reliability: Many public datasets are maintained by reputable organizations, ensuring their credibility.
However, the use of public data carries some caveats. It's crucial to assess the accuracy and relevance of datasets, as well as their possible biases often intertwined with the methods of their collection. Misinterpretations tied to data quality might adversely affect analysis and results.
Private Databases
In contrast to public data, private databases offer proprietary information that can be pivotal in driving Signal AI's effectiveness in specific industries. These databases are typically accessible through paid subscriptions or require partnership agreements. High-value private data elements can involve customer transaction records, commercial data, and market research reports from leading firms.
Using private databases provides numerous benefits including:
- Tailored insights: They often contain granular information specific to individual organizations, thus allowing market-targeted strategies.
- Access to real-time data: Businesses can gain access to promptly updated datasets, ensuring market responsiveness.
- Strategic differentiation: Proprietary insights can give firms competitive advantages over those only relying on public data.
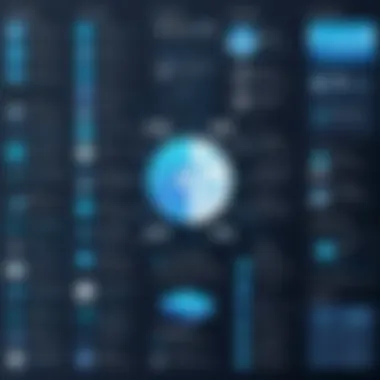
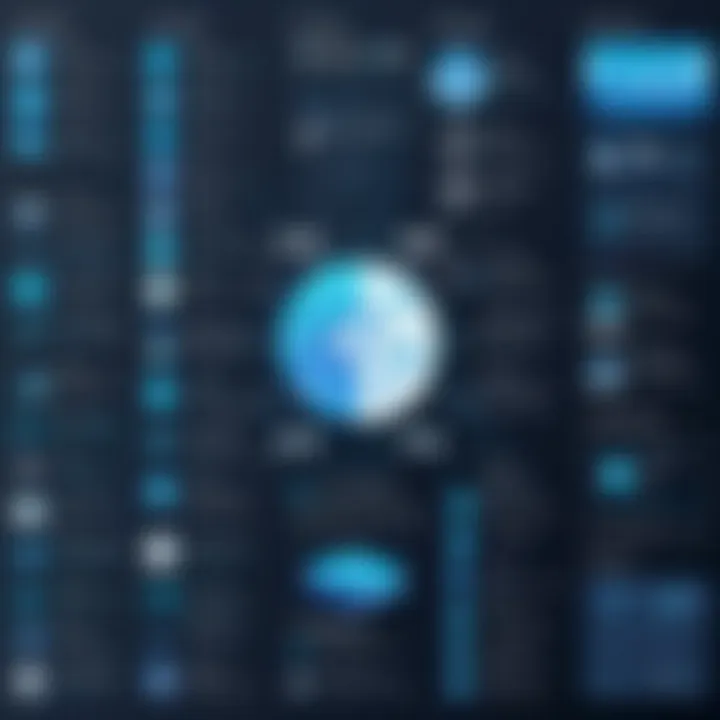
Nevertheless, leveraging private databases can surface challenges. Cost could arise when affording data, which might limit accessibility to smaller enterprises. Additionally, the integration of distinct private data into existing systems invites complex operational adjustments.
It’s essential to maintain an effective balance between public and private data sources.
By using a robust combination of both types, Signal AI maximizes the precision of analytics while capturing multiple dimensions of data-driven insights suitable for resolving current business needs.
Applications Across Industries
Understanding how Signal AI applies across various industries is critical in grasping its impact on contemporary business processes. The platform’s ability to analyze real-time data signals enhances functionality in diverse sectors, driving innovations that lead to better decision-making, more efficient operations, and improved service delivery.
Financial Services
In the financial sector, Signal AI provides a powerful tool for risk assessment and management. The platform processes vast amounts of market data, allowing financial institutions to predict trends and assess the potential impact of global events in real time. With its analytical capabilities, Signal AI enhances fraud detection significantly. The machine learning algorithms discern patterns indicative of suspicious activity. Furthermore, by utilizing signals from various data sources, banks and investment firms can optimize their portfolios by making data-driven investment decisions. This results in streamlined operations and increased profitability.
- Fraud Detection: Signal AI analyzes transaction data for signs of fraud. This immediate insight aids in protecting consumers and financial institutions from potential losses.
- Market Predictions: Real-time analysis of market trends can lead to more informed investment strategies, allowing firms to position themselves favorably.
Signal AI not only reduces risks through accurate insights but also increases compliance by constantly monitoring transactions that may raise regulatory concerns.
Healthcare Innovations
Healthcare is another field where Signal AI's capabilities shine. The platform aggregates and analyzes patient data, enhancing clinical decision-making by providing practitioners with accurate assessments of patient conditions in real-time. Furthermore, it streamlines administrative processes such as scheduling and billing.
An example includes operational enhancements in hospitals where Signal AI predicts patient inflow based on previous data, optimizing resource allocation to ensure effective patient care.
- Patient Monitoring: Predictive analytics help in early detection of complications, ultimately improving patient outcomes.
- Efficient Resource Management: Hospitals can better manage staffing and equipment, leading to cost savings and better patient care.
The integration of Signal AI into health informatics holds promise for revolutionary advancements in treatment protocols and patient care quality.
Marketing and Consumer Insights
In marketing, Signal AI offers companies tools to analyze consumer behavior effectively. Being able to comprehend signals from various consumer interactions provides businesses the ability to tailor their strategies uniquely for different audiences. This approach enhances customer engagement and satisfaction.
The continuous data integration allows firms to respond swiftly to market changes, recalibrating their strategies based on real-time feedback.
- Personalized Marketing: By interpreting consumer signals, businesses can create highly targeted advertisements that resonate with specific demographics.
- Competitor Analysis: Signal AI enables businesses to understand competitor behaviors in real time, allowing for agile strategic pivoting.
Through these applications, Signal AI creates opportunities for businesses to increase their market share and meet consumer needs efficiently.
Benefits of Using Signal AI
Signal AI presents various advantages for businesses and professionals across multiple sectors. Understanding these benefits is crucial for making informed decisions about integration and utilization of this technology. The following sections analyze how it can enhance decision-making processes and boost efficiency and productivity, inviting further exploration of its impact.
Enhanced Decision-Making Processes
One of the core advantages of Signal AI is its capacity to improve decision-making. It achieves this by providing real-time insights gathered from vast signals ranging over countless data sources. These insights are not only quick but also contextually richer. In uncertain and rapidly evolving markets, timely access to relevant data can distinguish a successful strategy from a failing one.
Signal AI applies advanced algorithms to sift through noise in data to find the signals that matter. This technology plays a critical role in understanding market dynamics, competitive movements, and shifts in consumer preferences. For professionals in IT and data analysis, the ability to make sense of complex datasets quickly translates to more significant advantages in strategic planning.
Consider the following points:
- Data-Driven Decisions: Improved confidence in choices made.
- Time-Saving: Faster information retrieval.
- Market Adaptability: Easier adjustments in response to real-time data.
“In the world of business, real-time data can convert uncertainty into predictability.”
For organizations focusing on sustainable growth, the tools offered by Signal AI propose a path where intuition amplified by factual data can lead to better outcomes.
Increased Efficiency and Productivity
Another prominent benefit of using Signal AI is the substantial increase in operational efficiency. By automating data processing and analysis, businesses can manage time and resources more effectively. Signal AI streamlines workflows, allowing professionals to focus on high-level strategy rather than spending excess time on rudimentary data tasks.
Automation does not merely reduce labor input; it also increases accuracy. Errors that often occur with manual processes decline significantly. This reliability translates into smoother transactions, optimal resource allocation, and ultimately enhances productivity.
Key efficiency factors include:
- Automation of Routine Tasks: Frees up resources for complex research.
- Better Resource Management: Ensures optimal deployment of personnel.
- Integration Across Platforms: Harmonizes varied workflows.
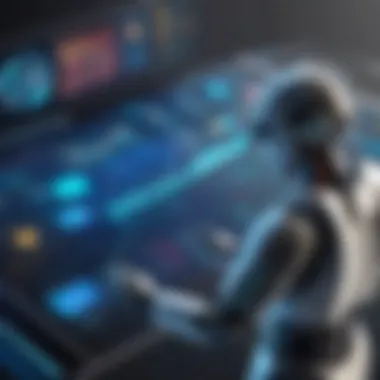
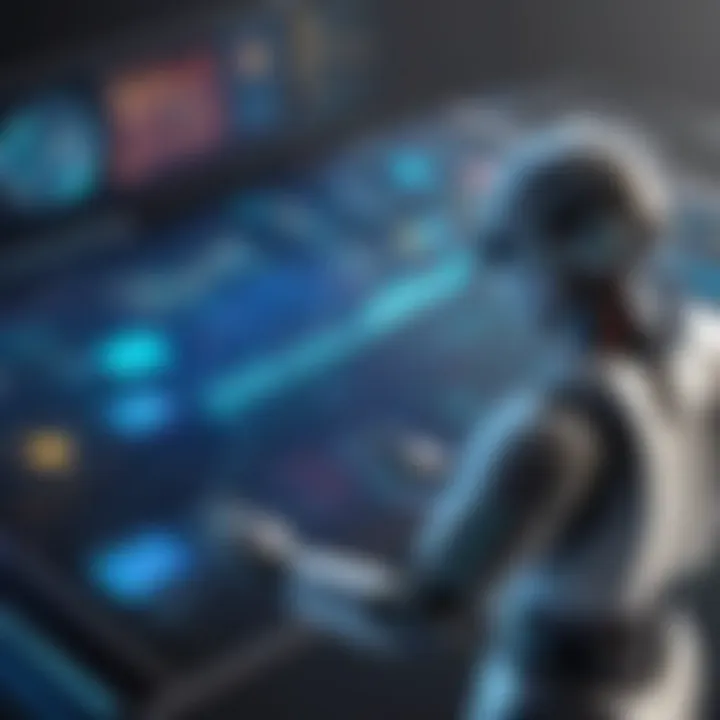
Additionally, productivity doesn’t only improve within operations; it extends to customer interactions. Companies utilizing Signal AI are better equipped to engage customers through enhanced understanding of preferences and behavior.
Both decision-making and productivity benefits bolster the case for adopting Signal AI. As industries continue to evolve, maintaining competitiveness becomes leveraged on how strategically data can be utilized. Professionals in software development and IT applications should regard these benefits as essential considerations when exploring the vast landscape of artificial intelligence solutions.
Challenges and Limitations
The evolution of Signal AI, while significant, does bring forth challenges and limitations that must be understood by end-users and stakeholders. This section delves into data privacy concerns and integration difficulties. These elements play crucial roles in assessing the value and implementation feasibility of Signal AI across various sectors.
Data Privacy Concerns
Data privacy is a major consideration when implementing Signal AI. The platform’s operational reliance on real-time data from diverse sources can raise significant issues regarding how sensitive information is handled. Compliance with regulations, such as the General Data Protection Regulation (GDPR), is paramount. Any data analyzed or processed must align with standards meant to protect user rights and confidentiality.
Signal AI utilizes large volumes of data, which often includes Personally Identifiable Information (PII). Its algorithms might inadvertently expose user data if not adequately monitored. Companies must ensure stringent data governance policies are established before deploying such systems. The repercussions of failing to uphold privacy can be detrimental, bringing legal ramifications and damaging reputations.
Here are several factors in play regarding data privacy:
- Regulations Compliance: Organizations must stay always updated with legal expectations on data management.
- User Trust: Maintaining user trust is critical for business continuity, established through transparent data practices.
- Informative Consent: Users should always be informed about data usage, fostering an ethical approach to data management.
In sum, making strides in AI technologies must not come at the expense of users' privacy. High-profile cases of data breaches underline the need for vigilance and protection.
Integration Difficulties
Integrating Signal AI into existing systems poses its own unique set of challenges. Companies often run large-scale legacy systems, which may not be compatible with the advanced infrastructure Signal AI requires. Transition challenges can arise during the adoption phases if systems cannot easily communicate or operate together.
Facilitating such integration involves several critical considerations:
- Compatibility: Assessing whether existing systems can adopt new technologies without disrupting their normal functions is essential.
- Staff Training: Employees may require robust training programs to effectively work with new AI tools. Insufficient knowledge can lead to poor implementation.
- Resource Allocation: Beyond hardware.software needs, maintaining services and support for both AI and pre-existing systems adds additional operational costs.
Furthermore, security vulnerabilities may surface during the integration unknowns unless those are actively mapped and thoroughly addressed.
Future Potential of Signal AI
The future potential of Signal AI holds significant value, especially as data-driven decision-making becomes crucial across various industries. The platform's ability to harness real-time signals can create competitive advantages for businesses. Along with its integration into existing workflows, it may lead to enhanced operational efficiencies and informed strategic choices.
Emerging Trends in AI Technologies
The landscape of artificial intelligence continues to evolve. Key trends impacting Signal AI include:
- Natural Language Processing: AI's ability to understand and interpret human language improves its interaction capabilities. Enhanced NLP can allow Signal AI to analyze customer feedback and sentiment effectively.
- Automated Machine Learning (AutoML): Automation in machine learning streamlines the model training process, reducing barriers for non-experts. Signal AI can benefit from AutoML by rapidly adapting to new developments without extensive human intervention.
- Advancements in Data Analytics Techniques: The integration of sophisticated analytical methods, such as deep learning and reinforcement learning, will enrich Signal AI's data processing capabilities.
The rise of cloud computing is also relevant. It promotes scalability and flexibility. As businesses continue to adopt cloud solutions, Signal AI can easily expand its tools and services, ensuring accessibility to a wider range of users.
Expanding Application Use Cases
Signal AI's versatility opens it to various application scenarios. Some emerging use cases include:
- Real-Time Compliance Monitoring: In heavily regulated fields like finance, real-time analysis helps companies stay ahead of compliance requirements and minimize risks.
- Predictive Healthcare Analytics: In healthcare, Signal AI can integrate diverse health data sources, aiding in predictive analysis for better patient outcomes.
- Customizable Marketing Strategies: Signal AI allows marketers to tailor campaigns by analyzing customer behavior patterns and preferences.
As new industries adopt AI technologies, the potential use cases for Signal AI will inevitably expand. Combined with the aforementioned AI trends, such growth will likely result in innovations that significantly enhance its functionalities.
The future progression of Signal AI is pivotal for businesses aiming to leverage valuable insights from expansive datasets for effective decision-making.
Epilogue
Understanding Signal AI is not just an intellectual exercise. The technology shapes how modern professionals, especially in tech fields, approach data interpretation and decision-making. The implications of integrating real-time analysis into various sectors signify significant trends in progress, but also issues needing consideration.
Summarizing Key Points
To encapsulate our discussion, the key takeaways of Signal AI involve:
- Real-time Data Processing: Analyzing data signals instantly from numerous streams provides timely insights.
- AI Technologies: Sophisticated machine learning algorithms enhance data evaluation capabilities.
- Cross-Industry Impact: Industries like finance, healthcare, and marketing have begun to leverage this technology effectively.
- Benefits & Challenges: While Signal AI brings efficiency, data privacy and integration difficulties also present hurdles.
- Future Potential: Emerging trends indicate that Signal AI may redefine problem-solving strategies in versatile contexts.
Summing these points elucidates the overarching narrative of exploiting AI for enhanced decision-making processes.
Implications for Future Research
The prospects for further exploration into Signal AI yield significant implications. Research could focus on:
- Ethical AI Development: As data privacy concerns evolve, developing ethical standards in AI applications becomes crucial. Research in policies and regulations can effectively mitigate risks associated with misuse of real-time data.
- Integration Innovation: Future studies can also benefit from examining new integrations with existing systems. A clearer understanding of friction points leads to improved solutions and tools.
- Advanced Algorithms: Continual advances in machine learning necessitate ongoing investigation into algorithm performance under various conditions. Better algorithms will optimize data utilization.
As research progresses, the careful navigation of these implications structures a pathway for more efficient and ethical AI deployment.
Always remember, optimizing real-time information is a competitive advantage, paramount for industry leaders.