Statice Synthetic Data: Innovations and Implications
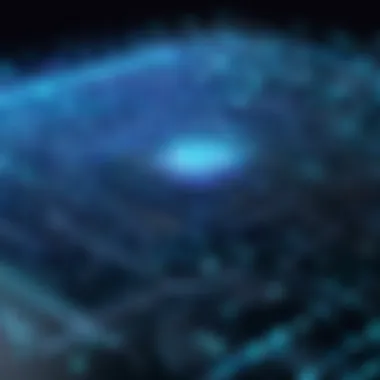
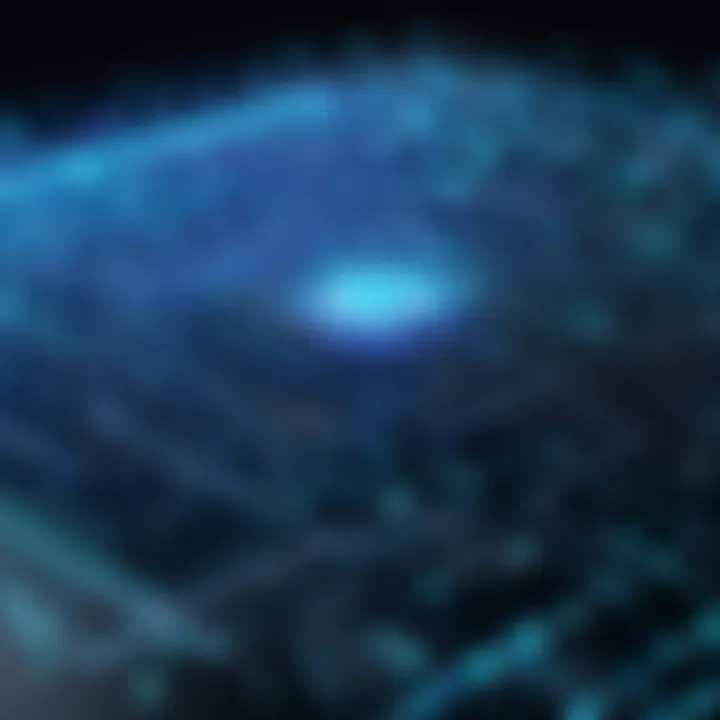
Intro
The emergence of synthetic data has reshaped how organizations approach data handling in modern technology. Statice synthetic data, in particular, has drawn attention for its unique offerings and potential applications across various industries. This article aims to explore the intricate components of Statice synthetic data, alongside its innovative structure and ethical implications. By delving into the features of this tool, its usefulness in current practices, and the significance of cautious data use, we provide insights that are relevant and necessary for todayโs data-driven world.
Software Overview
Statice offers a comprehensive suite of tools specifically tailored for generating synthetic data. This serves various purposes, from enriching datasets to facilitating robust data analyses without compromising privacy.
Features and Functionalities Overview
- Data Generation: Statice allows users to create synthetic datasets that maintain the statistical properties of real data. This is crucial for testing algorithms or training machine learning models without risking sensitive information.
- Flexibility: One of the standout features is the ability to emulate various data types, from tabular data to more complex structures. This adaptability ensures that organizations can utilize the tool across different scenarios.
- Privacy Protection: Statice incorporates mechanisms that safeguard real data from exposure, thus ensuring compliance with data protection regulations.
User Interface and Navigation
The user interface of Statice is designed with usability in mind. The layout is straightforward, facilitating users to navigate seamlessly through various features. With clearly labeled options, users can easily access data generation functionalitiesโfrom uploading base datasets to exporting synthetic versions.
Compatibility and Integrations
Statice is compatible with a range of existing software systems commonly used in data analytics and AI projects. It integrates well with tools such as Python and R, allowing for a smoother workflow when conducting data analyses. Additionally, its cloud-based functionality ensures accessibility from multiple devices, making it a valuable asset for teams working remotely or in diverse environments.
Pros and Cons
Strengths
- High Privacy Standards: Statice prioritizes data privacy, enabling organizations to leverage data safely.
- Scalability: The tool can generate large datasets efficiently, accommodating growing data needs without compromising performance.
- Advanced Customization: Users can tailor the output datasets according to specific requirements, enhancing usability.
Weaknesses
- Learning Curve: New users may experience an initial learning period as they familiarize themselves with advanced features.
- Dependence on Quality of Input Data: The effectiveness of synthetic data generation relies heavily on the quality of the initial datasets provided.
Comparison with Similar Software
Compared to other synthetic data generation tools, such as MOSTLY AI and Hazy, Statice stands out due to its robust privacy measures and flexible data modeling capabilities. While all platforms aim to provide synthetic data, Statice's emphasis on user-friendly interfaces and integration flexibility may give it an edge in certain contexts.
Pricing and Plans
Subscription Options
Statice provides a variety of subscription plans designed for different needs. Organizations can choose from smaller plans for startups to enterprise-level solutions for larger corporations, providing both functionality and value.
Free Trial or Demo Availability
A demo version is available, allowing potential users to experience key functionalities before making a commitment. This transparency enables informed decision-making.
Value for Money
While pricing varies, many users find the cost justified by the features and capabilities offered. Compared to potential compliance risks associated with real data, using Statice may prove more cost-effective long-term.
Expert Verdict
Final Thoughts and Recommendations
Statice synthetic data presents a powerful option for organizations looking to enhance their data privacy while still leveraging datasets for analytics and AI. Its commitment to user needs and data protection is commendable.
Target Audience Suitability
This software is particularly suitable for software developers, data scientists, and IT professionals who require reliable synthetic data for development and modeling tasks. Educational institutions can also benefit from its capabilities, making it a valuable tool for research.
Potential for Future Updates
Looking forward, Statice may further enhance its offerings by expanding integration capabilities and increasing the types of data it can generate. As technology and data needs evolve, continuous improvement will be essential to remain competitive in this field.
With the growth of data usage, innovative tools like Statice synthetic data are crucial in ensuring data privacy while enabling analytics.
Understanding Synthetic Data
In todayโs digital world, the role of data has become pivotal in driving innovation and informing decision-making across diverse sectors. Understanding synthetic data is crucial as it represents an evolving method for data generation that addresses many limitations of traditional data collection processes. Synthetic data enables organizations to simulate real-world scenarios without compromising sensitive information. This capability is particularly significant in fields where data privacy is a priority, such as healthcare and finance.
Artificial intelligence and machine learning rely heavily on high-quality data to improve their algorithms. However, acquiring this data can present issues, including costs and ethical considerations. Synthetic data offers solutions by providing viable alternatives that enhance data utility without the associated risks. This article explores these significant aspects of synthetic data and how it can reshape the future of technology and analytics.
Definition and Characteristics
Synthetic data refers to information that is artificially generated rather than obtained from real-world events. This type of data maintains the necessary attributes of real data, thereby enabling its use in various applications while avoiding privacy issues. Characteristics of synthetic data include:
- Realism: It mimics the patterns and structure of actual data.
- Variability: Generation processes can yield diverse datasets that reflect numerous scenarios.
- Scalability: Organizations can produce vast amounts of data quickly, suitable for big data applications.
Each characteristic addresses specific challenges faced by industries today, enhancing overall data strategy.
The Role of Algorithms in Data Synthesis
Algorithms are foundational to creating effective synthetic data. They underpin processes that allow for the modeling and generation of data that resembles real-world datasets. Key roles of algorithms in this context include:
- Data Generation: Utilizing models such as Generative Adversarial Networks (GANs), algorithms create new data points similar to existing datasets.
- Data Quality Assurance: Advanced algorithms ensure that generated data meets quality standards necessary for analytical rigor.
- Adaptive Learning: Algorithms continuously refine their processes based on feedback, allowing them to generate increasingly accurate data.
This adaptability is essential for applications such as simulating user behavior or predicting market trends, where data accuracy directly impacts results.
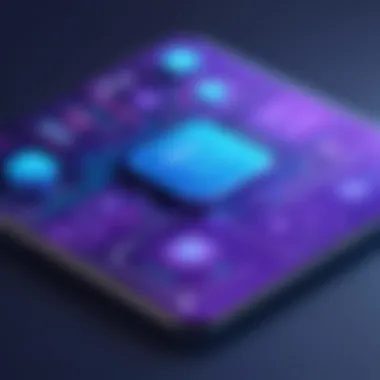
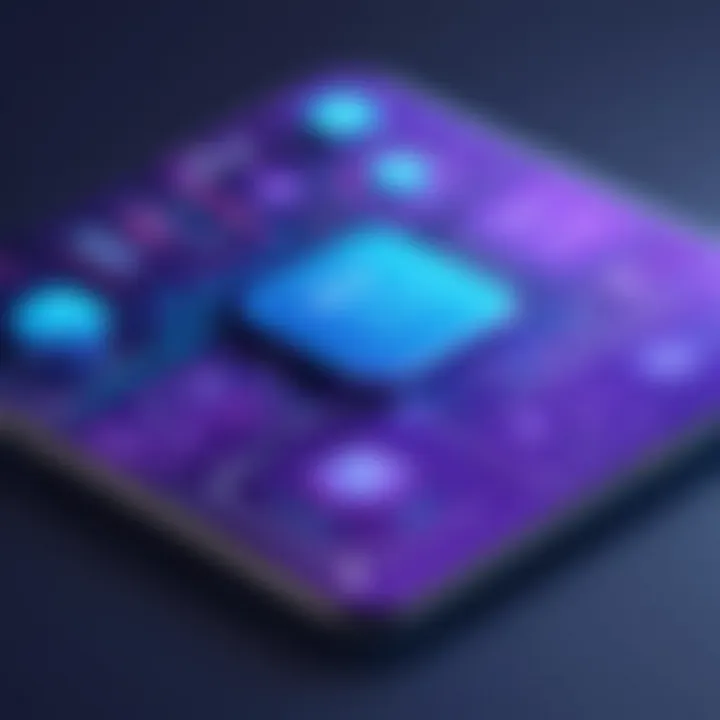
Types of Synthetic Data
Understanding the different types of synthetic data helps clarify its applications and advantages. There are three primary classifications:
Structured Data
Structured data refers to highly organized information that fits into predefined models, such as databases. This data is easy to analyze, making it a beneficial choice for applications needing quick insights or operational support. A critical characteristic of structured data is its format, which typically includes rows and columns, similar to spreadsheets. Its primary advantage is simplicity when querying and processing, allowing for rapid analysis in scenarios like business intelligence.
Unstructured Data
Unstructured data lacks a standard format and is more challenging to analyze. Examples include text, images, and videos. This type of data is gaining importance due to its vast availability and ability to hold significant insights. The key characteristic of unstructured data is its variability in form, which offers richer context for analysis. However, harnessing its potential requires sophisticated algorithms to derive meaningful patterns, making it both a challenging and rewarding area for synthetic data applications.
Semi-structured Data
Semi-structured data combines elements of both structured and unstructured data. It may follow a loose structure, such as JSON or XML formats, which allows for some level of organization while retaining flexibility. This type of data is beneficial because it can encapsulate complex relationships within datasets, providing richer information without the constraints of fully structured formats. Its unique feature is its adaptability, allowing integration across systems that require varying levels of data structure, particularly in scenarios involving APIs or data lakes.
Understanding these types lays the groundwork for effectively leveraging synthetic data in various applications.
Intro to Statice
The topic of Statice is crucial for understanding the advancements in synthetic data. Statice specializes in generating high-quality synthetic data that preserves the statistical properties of real datasets. This synthesis of data can significantly enhance privacy while providing avenues for analysis. It is important to know how Statice aligns with modern data requirements and challenges. By diving into its core elements, we unveil vital aspects of its impact on various sectors.
Company Overview
Statice, founded to tackle data privacy concerns, has positioned itself as a leader in synthetic data generation. The company offers innovative solutions aimed at providing businesses with the means to access data without compromising privacy. By utilizing advanced algorithms, Statice ensures that the data generated remains useful for analytics without risking exposure to sensitive information. This dual focus on utility and security makes Statice an appealing choice for organizations seeking compliant data practices.
Core Technology and Innovations
Generative Models
Generative models are at the heart of Staticeโs technology. These models excel at producing data that mimics the underlying structure of real datasets. A key characteristic of generative models is their ability to learn from existing data and create new datasets that reflect similar trends and patterns. This is beneficial in scenarios requiring data for machine learning training or testing frameworks.
One unique feature of generative models is their adaptability to various data types, whether they be structured or unstructured. This means organizations can generate useful datasets regardless of the initial data's nature. However, a noteworthy disadvantage is the potential for low fidelity in certain domains if the original data is not sufficiently rich or diverse.
Machine Learning Techniques
Machine learning techniques employed by Statice are designed to optimize the data generation process. These techniques include advanced algorithms, statistical processes, and artificial intelligence elements that contribute to high-quality data synthesis. The key characteristic of these techniques lies in their capability to improve the accuracy of generated data, ensuring that it remains representative of real-world scenarios.
A distinct advantage of using these techniques is the speed at which data can be generated, allowing for rapid prototyping in diverse applications, such as software development and testing. A drawback to consider, however, is the potential complexity of these methods, which may require specialized knowledge to implement effectively.
Key Features of Statice Synthetic Data
Statice synthetic data offers a myriad of features that enhance its utility across various fields. Understanding these features is crucial for professionals and students in IT-related fields who seek to leverage synthetic data effectively. The sophistication of these features can greatly facilitate the processes of data generation, privacy regulation, and analytical rigor in software development.
High-Fidelity Data Generation
High-fidelity data generation remains a cornerstone of Statice's approach. This feature ensures that the synthetic data mirrors real-world data closely, thus making it applicable in scenarios where accuracy is paramount. High fidelity is achieved through advanced algorithms that analyze patterns in actual datasets. As a result, the synthetic data generated retains critical statistical properties which are vital for tasks such as machine learning model training.
The implications of high-fidelity data extend beyond mere accuracy. When developers use synthetic data that accurately reflects real-world complexities, the risk of model bias is significantly reduced. This is especially important in sectors that demand precision, like healthcare and finance. High-fidelity synthetic data allows for comprehensive testing and validation in these contexts without compromising sensitive information.
Customizability and Flexibility
Customizability and flexibility are significant advantages of Statice synthetic data. Users can specify various parameters to tailor the data to their project's particular needs. For example, a user can create datasets that align with specific demographic attributes or supply chain variables. This kind of control is crucial for businesses that require targeted insights from their data.
Moreover, the platform's flexibility allows users to adapt datasets as needed throughout the development process. This is particularly beneficial in agile environments where requirements frequently change. By being able to adjust data characteristics dynamically, developers can streamline workflows and ensure their models remain relevant as project needs evolve.
Scalability for Various Applications
Scalability is a defining feature of Statice synthetic data, which positions it well to serve a range of industries. As organizations grow, the volume of data they work with often expands exponentially. Statice addresses this challenge by offering solutions that can be scaled up or down based on the demand.
For instance, in sectors like retail and finance, where data needs fluctuate seasonally, scalable solutions ensure that companies can generate the amount of data required without incurring excessive costs or time delays. This scalability is crucial as it supports the continuous development of technologies like AI and automated systems. Additionally, organizations find themselves capable of conducting larger experiments or tests without being constrained by the limits of traditional data collection methods.
The advantages of using Statice synthetic data are manifold, with its key features addressing important aspects of modern data challenges. High-fidelity generation, customizability, and scalability are integral to ensuring that developers and businesses can realize the potential of their data-driven initiatives.
Applications of Statice Synthetic Data
The application of Statice synthetic data is crucial in todayโs data-driven world. Organizations across various sectors are increasingly recognizing the importance of synthetic data for enhancing operational capabilities. Synthetic data offers solutions for data privacy, making it an invaluable asset for tech and analytics. By simulating real-world scenarios, Statice enables organizations to test systems, train models, and much more without the constraints associated with real data. This section will delve deeper into specific applications, highlighting the benefits and considerations.
Data Privacy and Security Testing
Statice synthetic data plays a vital role in data privacy and security testing. With regulations like GDPR and CCPA putting pressure on organizations to protect personal data, synthetic data offers a safe alternative. It mimics real user data but without any identifiable information, allowing security teams to test their systems rigorously.
- Key Benefits:
- Risk Mitigation: By using synthetic data, organizations minimize the risk of violating data privacy laws during testing.
- Comprehensive Testing: Security measures can be tested against realistic scenarios without the consequence of exposing actual data.
This is especially useful in situations requiring large datasets for penetration testing, ensuring robust defenses against potential data breaches.
Training Machine Learning Models
Machine learning models require vast amounts of data to learn effectively. Statice synthetic data provides an avenue for creating datasets that are tailored for specific training requirements. This not only fills gaps in data availability but also enhances the model's ability to generalize well.
- Advantages:
- Diversity of Data: Synthetic data can introduce variation that may not be present in the existing datasets, improving model robustness.
- Cost-Effectiveness: Acquiring real datasets can be expensive and time-consuming, while synthetic data generation can accelerate the process and reduce costs.
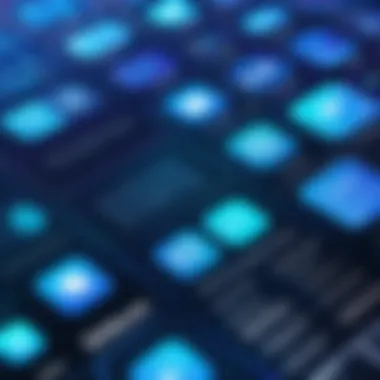
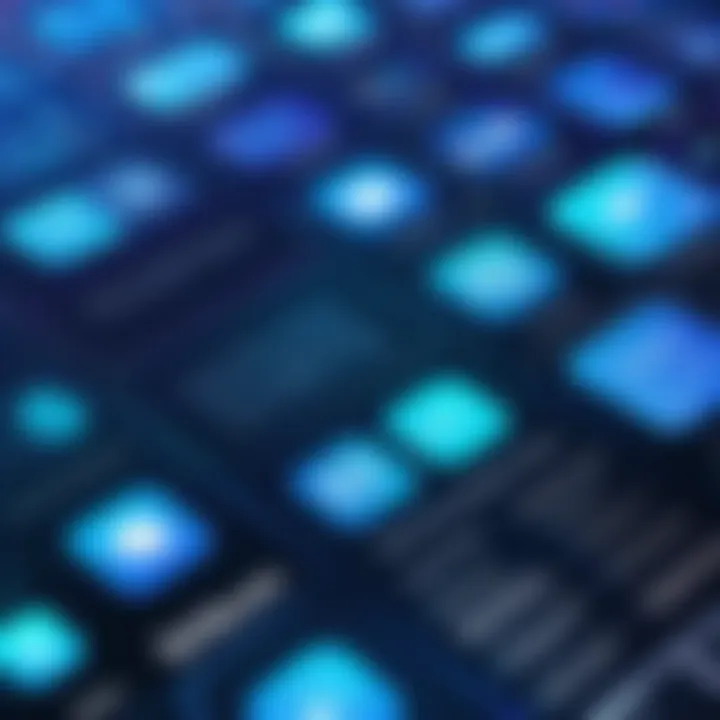
Using Statice in machine learning accelerates development cycles and can lead to innovative solutions based on synthetic training sets.
Enhancing Software Development Processes
In software development, the use of Statice synthetic data can significantly streamline processes. Development teams often need data for testing and validating new features without exposing sensitive information. Synthetic data is that bridge, allowing teams to carry out comprehensive tests while adhering to data security standards.
- Benefits:
- Faster Deployment: With readily available synthetic data, teams can speed up their testing phase, leading to quicker releases.
- Realistic Testing Environments: Developers can create environments that closely resemble production settings, leading to better quality assurance.
Statice aids in achieving a continuous integration and delivery model that is essential for modern software development.
Simulations in Various Industries
Statice synthetic data is instrumental in running simulations across different industries. Letโs take a closer look at three key sectors: healthcare, finance, and retail.
Healthcare
In healthcare, synthetic data assists in simulating patient data for research and analysis. This is critical in environments where real patient data cannot be shared due to confidentiality concerns.
- Characteristic: The ability to generate large datasets that mimic diverse populations enhances studies and models.
- Benefits: Researchers can conduct trials without ethical dilemmas, paving the way for better healthcare solutions.
However, the unique feature of having to ensure the data meets compliance standards adds a layer of complexity.
Finance
The finance sector benefits from synthetic data by using it to model financial scenarios and market trends. Financial institutions can analyze risks without using actual customer information.
- Characteristic: This allows for creating various risk profiles, enhancing their predictive analytics capabilities.
- Advantages: It ensures compliance with financial regulations while improving decision-making processes.
Counterbalancing this is the need to maintain accuracy, as errors in synthetic data could lead to poor financial predictions.
Retail
Retailers use Statice synthetic data to analyze customer behaviors and purchasing patterns. This helps in formulating effective marketing strategies and inventory management.
- Characteristic: Synthetic datasets can reflect changing trends without risking actual customer data leaks.
- Benefits: Retailers gain insights that can optimize product offerings and enhance customer experiences.
The disadvantage could be the reliance on synthetic data that may not fully capture nuances of real customer interactions.
The applications of Statice synthetic data are numerous and varied. They reflect a growing trend towards more intelligent data management practices that prioritize privacy and security while fostering innovation.
Benefits of Using Statice Synthetic Data
The use of Statice synthetic data represents a significant advancement in data generation techniques. Employing synthetic data provides distinct advantages that traditional methods cannot match. This section will explore how Statice synthetic data offers cost reductions, improved compliance with data privacy regulations, and faster development cycles.
Cost Reduction in Data Acquisition
One of the most immediate benefits of using Statice synthetic data is the reduction in costs associated with data acquisition. Traditionally, acquiring high-quality datasets often requires significant financial resources and time, particularly in industries where data is sensitive. By generating synthetic data, organizations can bypass many of these costs. It allows them to create the necessary data sets internally without relying on external sources that come with hefty price tags. Moreover, this reduces the dependency on real user data, which can be both expensive to gather and fraught with legal complications.
Synthetic data generation significantly lowers the need for expensive data acquisition processes.
Improved Data Privacy Compliance
Another crucial advantage of Statice synthetic data lies in its ability to enhance data privacy compliance. Many organizations operate under strict data regulations, such as the GDPR (General Data Protection Regulation) or CCPA (California Consumer Privacy Act). These regulations impose heavy penalties for data breaches or misuse. Synthetic data, generated to mimic real datasets without containing personally identifiable information, allows organizations to conduct testing and analysis while remaining compliant with these laws. It minimizes the risk associated with directly handling sensitive information, which is a significant concern for many companies.
Speeding Up Development Cycles
Using Statice synthetic data also contributes to accelerating development cycles. When organizations rely on real datasets, the data collection process can be lengthy and complicated. By switching to synthetic data, the time taken to gather and prepare data for machine learning models and software development is reduced drastically. This leads to shorter feedback loops and faster iteration cycles. Consequently, teams can focus on refining algorithms and improving their systems rather than on data management issues. Overall, this improves operational efficiency across various projects.
In summary, the benefits of using Statice synthetic data are profound. It promotes cost reductions in data acquisition, enhances compliance with privacy laws, and speeds up development processes. Incorporating synthetic data is not merely a trend but a vital strategy for organizations looking to improve their data handling practices.
For more on data privacy, you can refer to Wikipedia on Data Privacy.
Challenges in Synthetic Data Generation
The generation of synthetic data presents various challenges that deserve careful consideration. Understanding these challenges is critical for software developers, IT professionals, and students engaged with data-driven technologies. Addressing these issues not only enhances the quality of generated data but also ensures that applications utilizing this data behave as expected under real-world scenarios. Two primary challenges occur in this sphere: data quality concerns and bias and fairness issues.
Data Quality Concerns
One of the foremost challenges in synthetic data generation is ensuring data quality. Quality refers to how well the synthetic data reflects real-world scenarios. High-quality synthetic data should retain the statistical properties of the original data set, such as distribution, correlations, and variability. If the synthetic data lacks these essential characteristics, it can lead to misleading insights and poor decision-making in applications like machine learning and software testing.
Furthermore, the data quality can be affected by numerous factors. For instance, the algorithms used for data synthesis play a crucial role. If the underlying generative models are flawed or inadequately trained, the resulting synthetic data may exhibit inaccuracies or inconsistencies. Developers must keep this in mind when selecting or designing the tools they use for data synthesis. Regular evaluations and validation processes are essential to maintain the integrity of synthetic datasets, ensuring they meet the required standards for their intended applications.
Bias and Fairness Issues
Another significant challenge in synthetic data generation concerns bias and fairness. Bias can manifest in synthetic datasets if the original data includes any discriminatory or unjust patterns. For example, if the source data used for synthesis reflects societal inequalities, the synthetic data may perpetuate these unfair biases. This can have serious implications in fields such as healthcare and finance, where biased data outputs could lead to unfair treatment of certain groups.
Developers must focus on identifying and mitigating biases in the data generation process. Implementing fairness assessments during the data generation phase can help detect these issues early. Employing algorithms designed to promote equitable representation in the generated data is also critical. By recognizing and addressing these challenges, organizations can work towards ensuring their synthetic data is not only useful but also ethical and just.
"The goal is not just to generate data that mimics reality, but to ensure the generated data promotes fairness and equality."
Ethical Considerations in Using Synthetic Data
Understanding the ethical implications of using synthetic data is essential in todayโs data-driven landscape. Statice synthetic data offers significant innovations but also raises several ethical questions. These concerns revolve around how data is utilized and managed. With the utilization of synthetic data, it becomes crucial to maintain transparency and ensure responsible use.
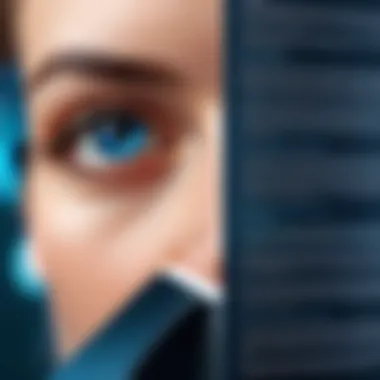
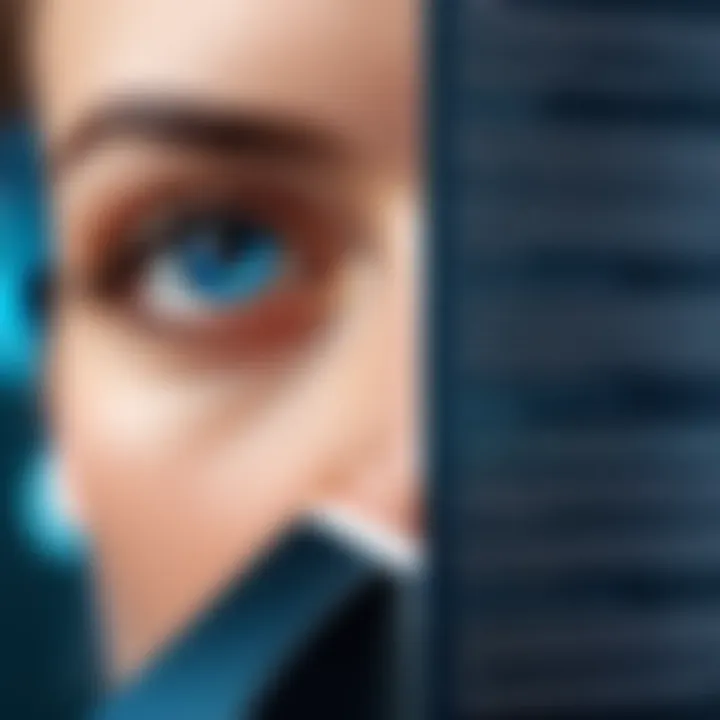
Ethical considerations in using synthetic data involve several elements that must be addressed. First, there is the question of consent. Even though synthetic data is not tied to real individuals, it can still be derived from real datasets that often contain personal information. Therefore, it is necessary to ensure that appropriate measures are in place to safeguard individual privacy and comply with regulations. Failure to do so can lead to reputational damage and legal implications for organizations.
In addition, there are considerations around data accuracy and bias. The process of generating synthetic data must ensure that it accurately represents the underlying distributions of the original data. This concern is especially important when the synthetic data is used to train machine learning models. If not managed correctly, bias can propagate, leading to unfavorable outcomes in automated decision-making systems. Thus, organizations using Statice synthetic data should have frameworks in place to regularly assess and mitigate bias in the generated datasets.
Moreover, organizations need to think about the broader social consequences of using synthetic data. Its impact extends to how businesses interact with customers and make decisions based on data insights. Ethical frameworks become vital in ensuring that the usage of synthetic data enhances, rather than detracts from, societal well-being.
Ethical Frameworks for Data Usage
Establishing ethical frameworks for data usage can serve as a guideline for organizations. A well-structured framework helps in navigating the complexities involved. Key aspects of these frameworks should include:
- Transparent Practices: Organizations should disclose how and why synthetic data is generated and used. Transparency builds trust and accountability.
- Responsible Data Stewardship: Emphasizing data care, firms should prioritize the responsible management of datasets to prevent misuse.
- Regular Auditing: Continuous monitoring of data processes will help address any emerging ethical issues proactively.
- Stakeholder Engagement: Involving stakeholders in discussions regarding data usage can provide diverse perspectives, enriching the ethical discourse.
By implementing these principles, organizations can better align their practices with responsible data usage.
Regulatory Impacts on Synthetic Data Generation
Regulatory frameworks significantly impact the generation and utilization of synthetic data. As data privacy and protection laws evolve, organizations must adapt their approaches accordingly. Regulations such as the General Data Protection Regulation (GDPR) in Europe profoundly affect how synthetic data is developed and employed.
The key principles of regulations that affect synthetic data generation include:
- Data Minimization: Organizations need to ensure that only the necessary data is collected and processed. This principle encourages the use of synthetic data when real data is not needed.
- Purpose Limitation: Data should be used only for the purposes for which it was collected. Misuse can lead to legal consequences.
- Accountability: Organizations must demonstrate compliance with regulations. This may include documentation and regular assessments of synthetic data processes.
The rise of regulations highlights the importance of ethical considerations. Companies that proactively adhere to these frameworks not only avoid legal ramifications but also enhance their reputation.
"Ethical engagement is not a regulatory burden; itโs an opportunity for organizations to build lasting trust with their customers."
Future Prospects of Statice Synthetic Data
The future of Statice synthetic data holds considerable promise, as it can significantly affect how data is generated and utilized across various domains. As organizations increasingly deal with data privacy challenges, the demand for innovative solutions, such as Statice synthetic data, rises. This will lead to progress in data utilization while maintaining strict privacy standards. Moreover, the evolution of technology, especially in artificial intelligence and machine learning, will enhance the potential applications of synthetic data, driving further advancements in the industry.
Emerging Trends in Data Synthesis
The field of data synthesis continues to evolve rapidly. Key trends indicate an increased focus on improving data quality, enhancing algorithms for better fidelity, and integrating synthetic data with real-world datasets. As the tools and techniques for data generation mature, businesses will require more accurate synthetic datasets to make informed decisions. Synthetic data is becoming a valid alternative to traditional data sources, mainly due to its agility and security benefits.
Potential Industry Transformations
Data Analytics
In data analytics, Statice synthetic data offers a pivotal advantage. It enables analysts to conduct experiments without compromising sensitive information. The crucial characteristic of synthetic data in this realm is its ability to provide high-quality datasets that mimic real data patterns while ensuring that no personal information is disclosed. This feature makes synthetic data a popular choice for organizations looking to derive insights without the ethical implications tied to actual data. However, users need to be cautious about relying solely on synthetic data, as it might not capture every nuance of the real-world data.
Artificial Intelligence
With artificial intelligence, synthetic data gives a robust platform for training models. It allows developers to create diverse and extensive datasets essential for building accurate AI systems. A notable feature of using synthetic data for AI is its potential for rapid scalability; one can generate large amounts of data tailored to specific needs. Nonetheless, it is vital to validate that the generated data reflects the complexities of real-world scenarios, to avoid bias in AI models. This validation process is crucial for the reliability of any AI system's outcomes.
Automated Systems
Automated systems can leverage Statice synthetic data for improved performance in various scenarios. By utilizing simulated data, these systems can be tested and optimized before real-world deployment. The key aspect of automated systems is their efficiency in managing data ingestion and processing without human intervention, making them beneficial in fast-paced industries. However, an important consideration is the quality and reliability of the generated data; if it does not reflect actual conditions well, it may lead to flawed conclusions or system failures.
"The evolution of synthetic data methods presents an opportunity for numerous sectors to embrace innovations while prioritizing data protection and ethical practices."
The integration of Statice synthetic data into various industries will likely continue to grow, as evidenced by increased investment and interest in this technology. As organizations recognize the benefits of synthetic data, the landscape for data generation and analytics will transform, creating a new standard for how data is produced and used.
Comparative Analysis with Other Data Generation Methods
The exploration of how synthetic data, especially from Statice, compares with traditional data generation methods is crucial. By understanding these differences, professionals can make informed decisions about data sourcing based on their specific needs. Traditional data collection often involves significant effort and resources, while synthetic data offers a quicker, more flexible alternative. This discussion will provide insights into both paradigms, highlighting the strengths and weaknesses of each.
Traditional Data Collection Methods
Traditional data collection methods encompass various techniques such as surveys, interviews, and observational studies. These methods can be time-consuming and costly, often requiring extensive planning. Each method's reliability hinges on several factors, including sample size, response rate, and data collection protocols. Some common characteristics of traditional methods include:
- Resource Intensive: Significant financial and human resources are needed to collect data.
- Time-Consuming: The process of gathering and validating data can take long time.
- Potential Bias: Human error and bias can influence results, affecting the overall quality of data.
While traditional methods have their place in academia and various industries, they often struggle to keep pace with the rapid developments in technology and data needs, making synthetic data a valuable alternative.
Statistical vs. Synthetic Data Approaches
The difference between statistical and synthetic data is essential to grasp for a proper understanding of their applications. Statistical data generation relies heavily on real-world observations and can often come from traditional data collection methods. In contrast, synthetic data is generated through algorithms and models that create a dataset resembling real data without the privacy concerns associated with it.
Some noted differences include:
- Data Authenticity: Statistical data usually reflects real-world conditions, while synthetic data is a constructed version meant to preserve characteristics without revealing real identities.
- Speed of Generation: Synthetic data can be produced rapidly, enabling faster testing, training, and analysis compared to the slower traditional methods.
- Cost Efficiency: Generating synthetic data can drastically reduce costs, allowing for a more scalable approach to data availability.
In summary, both traditional data and synthetic data have roles in various fields. Understanding their distinctions and applications can lead to more effective data use in technology and analytics.
Summary and Ending
In this final section, we consolidate the insights presented throughout the article on Statice synthetic data. Understanding these key points is crucial for software developers, IT professionals, and students who seek to leverage synthetic data in their work. The article highlights the transformative role of synthetic data in technology, particularly regarding innovations brought forth by Statice. Several factors underpin the significance of summarizing these discussions:
- Clarity of Key Concepts: This summary serves to clarify essential concepts. It ensures the readers can identify the core ideas about synthetic data and its applications.
- Highlighting Innovations: Statice has introduced advancements in data generation that merit attention. Understanding these innovations enables professionals to engage effectively with emerging technologies.
- Recognizing Benefits and Challenges: A recap brings forth both benefits and challenges associated with synthetic data usage. Being aware of these elements aids decision-making and informs responsible data practices.
Conclusively, the implications of synthetic data reach beyond mere technological enhancements. Its influence on privacy, data security, and ethical considerations play a vital role in its adoption across industries.
Recap of Key Points
- Definition of Synthetic Data: Provides a foundation for understanding what synthetic data entails. It mimics real data while preserving patterns and characteristics.
- Statice's Innovations: Innovative generative models have pushed boundaries in data synthesis, enabling high-fidelity data generation. This emphasizes the importance of advanced algorithms in creating synthetic datasets.
- Applications: Statice synthetic data finds diverse applications from data privacy testing and machine learning to simulations in healthcare and finance.
- Benefits: Key benefits include reduced costs in data acquisition, improved data privacy compliance, and expedited development cycles.
- Ethical and Regulatory Aspects: Ethical concerns surrounding bias and fairness, alongside regulatory impacts, form critical considerations for stakeholders.
Final Thoughts on the Future of Synthetic Data
Looking ahead, the future of synthetic data is promising yet challenging. Emerging trends suggest a growing integration of synthetic data in various fields. As industries evolve, the following points stand out:
- Increased Adoption in Analytics: Companies will likely enhance their data analytics capabilities using synthetic datasets. Traditional data sourcing methods may become less favored as synthetic alternatives prove effective.
- Advancements in AI and Machine Learning: Continuous improvements in machine learning models can lead to even more realistic synthetic datasets, pushing the boundaries of what is possible in areas like automated systems and predictive analytics.
- Greater Emphasis on Ethical Standards: As synthetic data becomes more prevalent, adherence to ethical standards and regulatory compliance will be critical. Industries must navigate these complexities carefully to avoid potential pitfalls.
Ultimately, the evolution of synthetic data technologies, particularly those advanced by Statice, indicates a significant shift in how organizations approach data management and utilization. Stakeholders must remain informed and adaptable as they explore this rapidly evolving landscape.