Unveiling the Intricacies of the Bi Tech Stack: A Comprehensive Examination
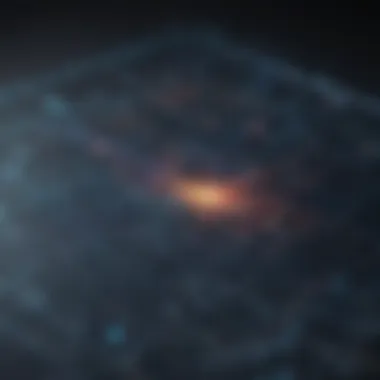
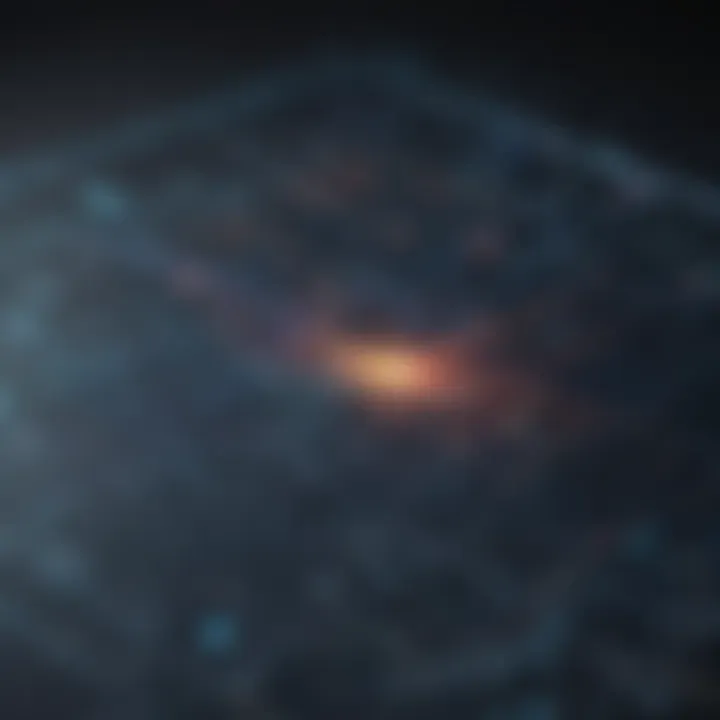
Software Overview
When exploring the Bi Tech Stack, one must first grasp the core concept of this intricate system. The Bi Tech Stack encompasses a wide array of components and functionalities that work in unison to elevate data management and analytical capabilities. From robust data storage mechanisms to cutting-edge analytics tools, every aspect of the Bi Tech Stack serves a specific purpose in enhancing business intelligence and decision-making processes. Understanding the main features of each software element within the Bi Tech Stack is crucial to unraveling its inner workings.
Features and Functionalities Overview
Each software component within the Bi Tech Stack offers a unique set of features and functionalities that contribute to its overall efficiency. For example, the data management software streamlines the storage and organization of vast datasets, ensuring quick access and retrieval when needed. On the other hand, analytics tools within the stack provide advanced data processing capabilities, enabling users to derive valuable insights from complex datasets. By comprehensively exploring these features, users can harness the full potential of the Bi Tech Stack for their analytical needs.
User Interface and Navigation
A noteworthy aspect of the Bi Tech Stack is the user interface and navigation experience it delivers to users. The ease of use and intuitive design of the software components greatly impact the overall user experience. An intuitive user interface ensures that users can seamlessly navigate through different modules, access essential features, and perform analytical tasks with precision. By emphasizing user-friendly design principles, the Bi Tech Stack enhances user productivity and efficiency in handling vast amounts of data.
Compatibility and Integrations
When exploring the Bi Tech Stack, compatibility and integrations play a pivotal role in ensuring seamless data flow and connectivity across various systems. The ability of the software components to integrate with other devices or software systems is essential for creating a cohesive ecosystem for data analysis. Furthermore, compatibility with different devices ensures that users can access and analyze data regardless of their preferred platform or operating system. By examining the compatibility and integrations of each software element, users can maximize the interoperability of the Bi Tech Stack.
Introduction to Bi Tech Stack
In the vast landscape of technology, the Bi Tech Stack stands out as a fundamental pillar that drives critical decision-making processes and business intelligence. The intricate web of components within the Bi Tech Stack plays a pivotal role in transforming raw data into actionable insights. By focusing on data management and analytics tools, this article aims to shed light on how the Bi Tech Stack significantly influences the realm of technology. Exploring each element in detail will provide a profound understanding of how businesses leverage the Bi Tech Stack to optimize their operations and strategic initiatives.
Overview of Bi Tech Stack
Definition and Purpose of Bi Tech Stack
The Definition and Purpose of the Bi Tech Stack represent the core foundation of this comprehensive analysis. This essential aspect encapsulates the essence of leveraging technology for enhancing business intelligence and decision-making processes. Providing clarity on the objectives and functions of the Bi Tech Stack is vital for understanding its significance in driving organizational success. By delving into this key characteristic, readers can grasp the transformative power of the Bi Tech Stack and how it aligns with contemporary business demands.
Evolution of Bi Tech Stack
The Evolution of the Bi Tech Stack sheds light on the historical progression and advancements within this sophisticated technological framework. Understanding how the Bi Tech Stack has evolved over time offers valuable insights into the constant innovations and adaptations in the field of business intelligence. By highlighting the distinctive features and contributions that have shaped its development, readers gain a comprehensive view of why the Bi Tech Stack remains a preferred choice for organizations seeking actionable insights and data-driven strategies.
Key Components
Data Warehouse
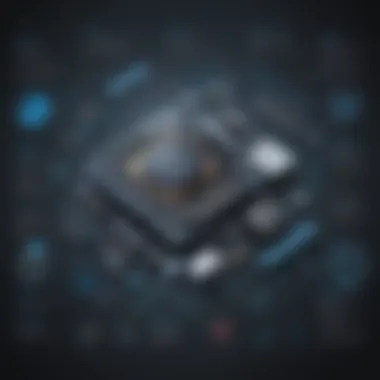
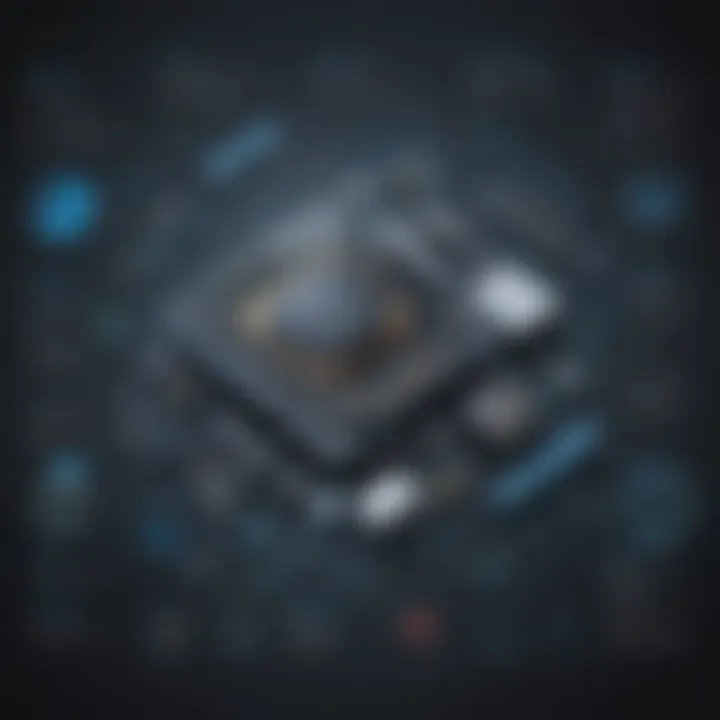
At the core of the Bi Tech Stack lies the Data Warehouse, a crucial component that enables the storage and management of vast amounts of data. The role of the Data Warehouse in centralizing information for analytical purposes is unparalleled, making it a popular choice for organizations aiming to streamline their data processing workflows. The unique features of the Data Warehouse, such as scalability and performance optimization, enhance its appeal in this article by providing efficient data storage solutions with both advantages and disadvantages for consideration.
Data Integration Tools
Data Integration Tools play a pivotal role in consolidating data from various sources and formats, ensuring seamless data flow within the Bi Tech Stack. By discussing the key characteristics of Data Integration Tools, including ETL processes and data quality management, readers can appreciate their significance in ensuring data accuracy and consistency. The advantages and limitations of these tools are crucial for organizations looking to optimize their data integration processes and maximize the value extracted from disparate data sets.
Data Visualization Software
Data Visualization Software serves as a visual gateway to the insights derived from complex datasets, empowering users to explore data analytics through interactive dashboards and reporting features. The importance of visual representation in data analysis cannot be overstated, making Data Visualization Software an indispensable component of the Bi Tech Stack. By outlining the unique features and functionalities of these tools, readers can understand their role in facilitating data-driven decision-making and enhancing overall business intelligence efforts.
Importance of Bi Tech Stack
Enhanced Decision-Making
Enhanced Decision-Making stands as a cornerstone of the Bi Tech Stack, offering organizations a data-driven approach to strategic planning and operational execution. The key characteristic of Enhanced Decision-Making lies in its ability to leverage real-time insights and predictive analytics to foster informed decision-making processes. By exploring the unique features of Enhanced Decision-Making, readers can grasp its impact on accelerating organizational growth and adapting to dynamic market conditions, providing a blend of advantages and disadvantages for critical evaluation.
Improved Data Quality
Improved Data Quality underpins the foundation of the Bi Tech Stack, emphasizing the importance of accurate and reliable data for driving actionable insights. The key characteristic of Improved Data Quality lies in its proactive measures to enhance data integrity and precision, mitigating the risks associated with erroneous data interpretation. By articulating the unique features and benefits of focusing on data quality within the Bi Tech Stack, readers can appreciate its role in optimizing decision-making processes and fortifying the organization's data-driven culture.
Core Elements of Bi Tech Stack
As we venture into the realm of the Bi Tech Stack, understanding its core elements becomes pivotal for a comprehensive analysis in this article. The Bi Tech Stack comprises essential components like Data Warehouse, Data Integration Tools, and Data Visualization Software, each playing a unique role in the realm of business intelligence. Exploring these core elements sheds light on how raw data is managed, processed, and transformed into actionable insights for informed decision-making processes. By dissecting the intricacies of these components, readers can grasp the significance of the Bi Tech Stack in enhancing organizational efficiency and strategic planning. Considerations about scalability, compatibility, and performance optimization are crucial aspects that shape the efficacy of core elements within the Bi Tech Stack.
Data Warehouse
Role of Data Warehouse in Bi Stack
The role of a Data Warehouse in the Bi Stack ecosystem is fundamental, serving as a centralized repository for storing and managing vast amounts of structured data. Its contribution lies in facilitating data integration from multiple sources, enabling organizations to consolidate diverse datasets for analytical purposes. The key characteristic of a Data Warehouse is its ability to support complex queries and analytics, fostering a data-driven culture within enterprises. Its advantageous nature stems from providing a unified view of data for faster decision-making and strategic insights. While offering enhanced data accessibility, the Data Warehouse also necessitates robust data governance and maintenance practices to ensure data quality and consistency.
Types of Data Stored
Data Warehouses store various types of data, including historical, aggregated, and transactional data, crucial for analytical processes within the Bi Tech Stack framework. The diversity of data types allows organizations to explore trends, patterns, and anomalies, driving valuable business outcomes. Its unique feature lies in schema-on-read architecture, enabling on-the-fly data structuring for dynamic business needs. This flexibility offers advantages in adaptability and scalability but may pose challenges in performance optimization and data processing efficiency, requiring strategic data modeling and indexing for optimal performance.
Data Integration Tools
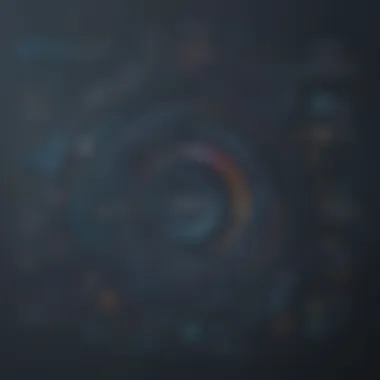
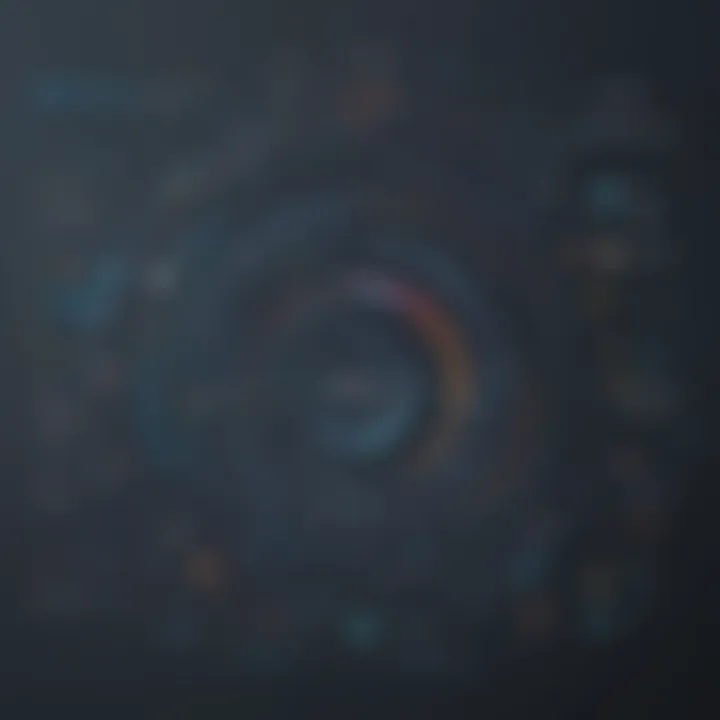
ETL Processes
ETL processes, Extract, Transform, Load, exemplify the backbone of seamless data integration within the Bi Tech Stack environment. These processes play a vital role in extracting data from disparate sources, transforming it into a consistent format, and loading it into the Data Warehouse for analysis. Their key characteristic lies in automating data workflows, ensuring data consistency and integrity across various operational systems. The uniqueness of ETL processes lies in their ability to handle large volumes of data efficiently, enhancing data processing speed and accuracy. While advantageous in streamlining data pipelines, ETL processes require meticulous data mapping and transformation rules to mitigate errors and ensure data quality standards.
Data Quality Management
Data Quality Management is a critical aspect of the Bi Tech Stack, focusing on maintaining accurate, consistent, and reliable data for analytical purposes. Its key characteristic lies in establishing data quality frameworks, defining data validation rules, and implementing data cleansing strategies to improve data accuracy and completeness. The uniqueness of Data Quality Management lies in its ability to identify and rectify data anomalies, ensuring data reliability and trustworthiness for decision-making processes. While advantageous in enhancing data integrity, data quality management necessitates continuous monitoring, governance, and stakeholder collaboration to uphold data quality standards and regulatory compliance.
Data Visualization Software
Interactive Dashboards
Interactive Dashboards are indispensable tools in the Bi Tech Stack, enabling users to visualize and explore data insights through dynamic and user-friendly interfaces. Their key characteristic lies in providing interactive data visualization features, allowing users to drill down into specific metrics, filter data dynamically, and extract actionable insights in real-time. The uniqueness of Interactive Dashboards lies in their ability to promote data-driven decision-making by presenting complex data relationships in an intuitive and engaging manner. While advantageous in enhancing data comprehension, Interactive Dashboards require user training and interface customization to align with user preferences and analytical requirements.
Reporting Features
Reporting Features in Data Visualization Software play a vital role in communicating analytical findings and business performance metrics effectively within organizations. Their key characteristic lies in generating customizable reports, dashboards, and visualizations, catering to diverse stakeholder needs and preferences. The uniqueness of Reporting Features lies in providing automated report scheduling, real-time data updates, and ad-hoc report generation capabilities, facilitating timely decision-making and performance monitoring. While advantageous in streamlining reporting processes, Reporting Features require data visualization best practices, template customization, and integration with other BI tools for comprehensive data reporting and analysis.
Integration and Implementation Strategies
The Integration and Implementation Strategies section underlines the pivotal role of efficient integration practices and deployment methods in maximizing the potential of the Bi Tech Stack. In this detailed analysis, we delve into the intricate web of data governance policies and the collaborative dynamics between IT and business teams, shedding light on their contributions to the seamless functioning of the Bi Tech Stack. By highlighting the significance of effective integration practices, we aim to provide software developers, IT professionals, and students with a profound insight into establishing robust data management frameworks for enhanced decision-making processes and improved data quality. Through a meticulous examination of deployment methods, including cloud-based deployment and on-premise installation, we aim to dissect the unique features, advantages, and disadvantages of each approach, equipping readers with the knowledge to navigate and implement Bi Tech Stack solutions with precision and foresight.
Effective Integration Practices
- Data Governance Policies: The crux of effective integration practices lies in the formulation and enforcement of stringent data governance policies. These policies serve as the foundation of data management, dictating the guidelines for data collection, storage, and usage within an organization. By implementing comprehensive data governance policies, businesses can ensure data integrity, compliance with regulatory standards, and enhanced data security. The key characteristic of data governance policies is their ability to establish a standardized framework for data handling, mitigating risks associated with data misuse or unauthorized access. While data governance policies offer significant advantages in ensuring data reliability and regulatory compliance, they may introduce complexities in data workflows and require substantial resources for implementation and maintenance within the Bi Tech Stack ecosystem.
- Collaboration between IT and Business Teams: Effective integration practices are further bolstered by fostering seamless collaboration between IT and business teams. The synergy between these two vital pillars of an organization facilitates the alignment of technology solutions with business objectives, ensuring that Bi Tech Stack implementations are congruent with organizational goals. The key characteristic of collaboration between IT and business teams lies in its potential to bridge the communication gap, promote mutual understanding, and streamline decision-making processes related to data management and analytics. While this collaborative approach enhances the relevance and usability of BI solutions, it may encounter challenges related to conflicting priorities, differing perspectives, and resource allocation disparities, necessitating effective stakeholder engagement and change management strategies.
Deployment Methods
- Cloud-Based Deployment: Cloud-based deployment emerges as a prominent choice for organizations seeking flexible and scalable infrastructure solutions for their Bi Tech Stack implementations. The key characteristic of cloud-based deployment lies in its agility, enabling organizations to leverage cloud resources on a pay-as-you-go model, scalability, and elasticity that accommodate fluctuating data processing demands. By opting for cloud-based deployment, businesses can benefit from reduced infrastructure costs, simplified maintenance, and global accessibility to BI tools and data. However, cloud-based deployment may introduce concerns regarding data security, compliance with data sovereignty regulations, and dependency on third-party cloud service providers, requiring organizations to conduct thorough risk assessments and implement robust security measures to safeguard sensitive information.
- On-Premises Installation: On-premise installation retains its significance for organizations with stringent data privacy regulations, legacy systems dependencies, or specific security requirements necessitating localized data storage and processing. The key characteristic of on-premise installation lies in granting organizations complete control over their hardware, software, and data management processes, ensuring data sovereignty, compliance, and customization according to organizational needs. By opting for on-premise installation, organizations can address data residency concerns, maintain higher levels of data security, and adhere to industry-specific compliance standards. However, on-premise installations may entail higher upfront costs, longer deployment timelines, and increased maintenance overhead, necessitating a comprehensive evaluation of cost-benefit considerations and long-term IT infrastructure strategies.
Challenges and Solutions
In this comprehensive analysis of the Bi Tech Stack, focusing on the Challenges and Solutions aspect is crucial. Addressing challenges that arise in implementing and maintaining a Bi Tech Stack is vital to ensure its efficiency and effectiveness. By delving into the specific elements of challenges and solutions, readers can grasp the complexities involved in optimizing a Bi Tech Stack for business intelligence and decision-making processes. Understanding the considerations when mitigating challenges and implementing solutions is key to maximizing the benefits of a Bi Tech Stack.
Data Security Concerns
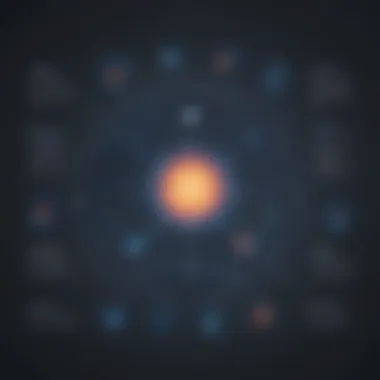
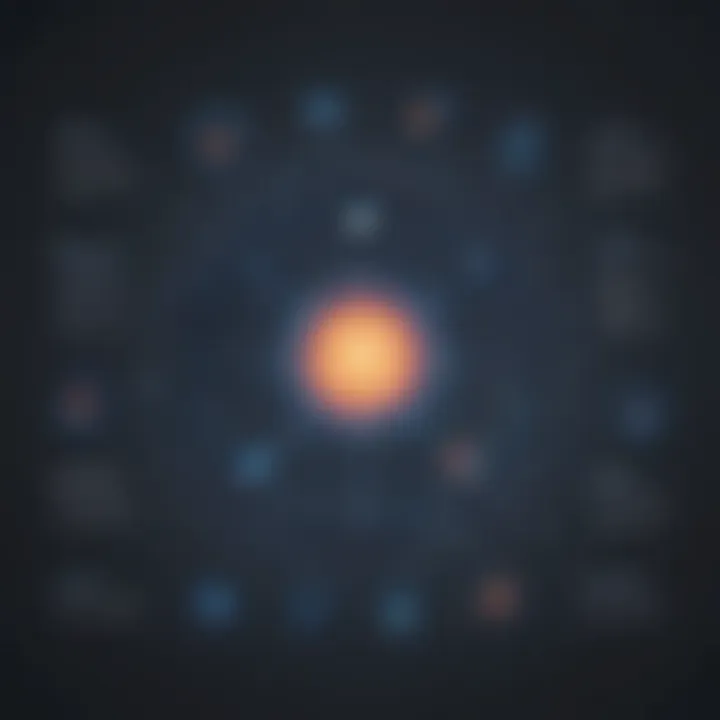
Encryption Protocols
Encryption protocols within the Bi Tech Stack play a pivotal role in safeguarding sensitive data and ensuring secure communication channels. The unique feature of encryption protocols lies in their ability to encode data, making it unreadable to unauthorized users. By utilizing advanced encryption algorithms, such as AES or RSA, organizations can fortify their data against cyber threats and breaches effectively. The advantage of encryption protocols in this context is the ability to maintain data integrity and confidentiality, contributing to overall data security within the Bi Tech Stack.
Access Control Measures
Access control measures are essential components of data security within the Bi Tech Stack, dictating who can access specific information and under what conditions. The key characteristic of access control measures is their ability to restrict unauthorized access and govern user permissions effectively. By implementing role-based access control (RBAC) or attribute-based access control (ABAC), organizations can enforce granular control over data accessibility, reducing the risk of data leaks and unauthorized disclosures. While access control measures enhance security protocols, they may also introduce complexities in managing user permissions and access levels within the Bi Tech Stack.
Scalability Issues
Vertical Scaling vs. Horizontal Scaling
The juxtaposition of vertical scaling and horizontal scaling presents inherent scalability challenges within the Bi Tech Stack infrastructure. Vertical scaling involves increasing the computational power of existing resources vertically, while horizontal scaling focuses on distributing data and workload across multiple resources horizontally. The key characteristic of vertical scaling lies in its ability to augment individual resource capacity, ensuring higher performance for specific tasks. In contrast, horizontal scaling offers enhanced redundancy and reliability by distributing workloads across diverse resources. Determining the most suitable scaling strategy depends on the organizational needs, workload demands, and future growth projections within the Bi Tech Stack environment.
Resource Allocation Strategies
Resource allocation strategies are pivotal in optimizing the performance and efficiency of the Bi Tech Stack, encompassing techniques to allocate resources effectively based on workload demands and system requirements. The key characteristic of resource allocation strategies is their ability to maximize resource utilization while minimizing bottlenecks and performance disparities. By employing dynamic resource allocation algorithms or auto-scaling mechanisms, organizations can adapt resource allocation in real-time to meet fluctuating demands within the Bi Tech Stack. While resource allocation strategies enhance system performance, improper allocation may lead to resource wastage or underutilization, necessitating continuous monitoring and optimization for sustained efficiency.
Future Trends in Bi Tech Stack
In the ever-evolving landscape of technology, staying abreast of the latest trends is vital for businesses to maintain their competitive edge. The Future Trends in Bi Tech Stack section aims to provide a glimpse into the horizon of business intelligence, focusing on upcoming advancements that are set to redefine data analysis and decision-making processes. This segment dissects potential technological innovations and their implications, offering readers a strategic foresight into the future of BI.
AI Integration
Machine Learning Algorithms:
Machine Learning Algorithms are the backbone of AI integration in the Bi Tech Stack. These algorithms enable systems to learn from data, identify patterns, and make decisions with minimal human intervention. Their ability to adapt and improve over time based on new data makes them instrumental in unlocking valuable insights from complex datasets. Despite their complexity, Machine Learning Algorithms offer unparalleled accuracy and efficiency in predictive modeling and data analysis, revolutionizing the way businesses derive actionable intelligence from raw information.
Predictive Analytics Capabilities:
Predictive Analytics Capabilities empower organizations to forecast future outcomes based on historical data and trends. By leveraging advanced statistical algorithms and machine learning techniques, predictive analytics transforms data into actionable insights, guiding strategic decision-making processes. The key strength of Predictive Analytics lies in its capacity to identify patterns and trends that may not be immediately apparent, thus aiding businesses in proactively addressing challenges and optimizing opportunities. While it empowers organizations with foresight, predictive analytics also requires robust data quality and model validation to ensure the accuracy and reliability of predictions.
IoT Integration
Data Collection from IoT Devices:
The proliferation of IoT devices has led to a data explosion, generating vast amounts of real-time information that can provide valuable insights for business operations. Data Collection from IoT Devices involves harnessing data from interconnected devices, sensors, and machines to derive actionable intelligence. The key advantage of IoT data lies in its timeliness and relevance, offering businesses the opportunity to make informed decisions based on up-to-the-minute information. However, managing and analyzing the sheer volume and variety of IoT data poses challenges in terms of data processing, storage, and security.
Real-Time Analytics:
Real-Time Analytics enables organizations to analyze data instantaneously, facilitating quick decision-making and response to changing conditions. By processing data in real-time, businesses can gain immediate insights into operational performance, customer behavior, and market trends. The ability to act swiftly based on real-time data gives organizations a competitive advantage in dynamic and fast-paced environments. However, real-time analytics necessitate robust infrastructure and efficient algorithms to handle continuous streams of data while ensuring accuracy and reliability in decision support.