The Evolution of Business Intelligence: Insights and Trends
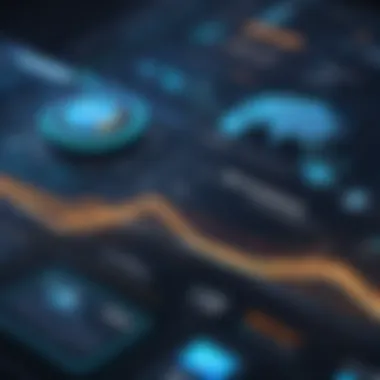
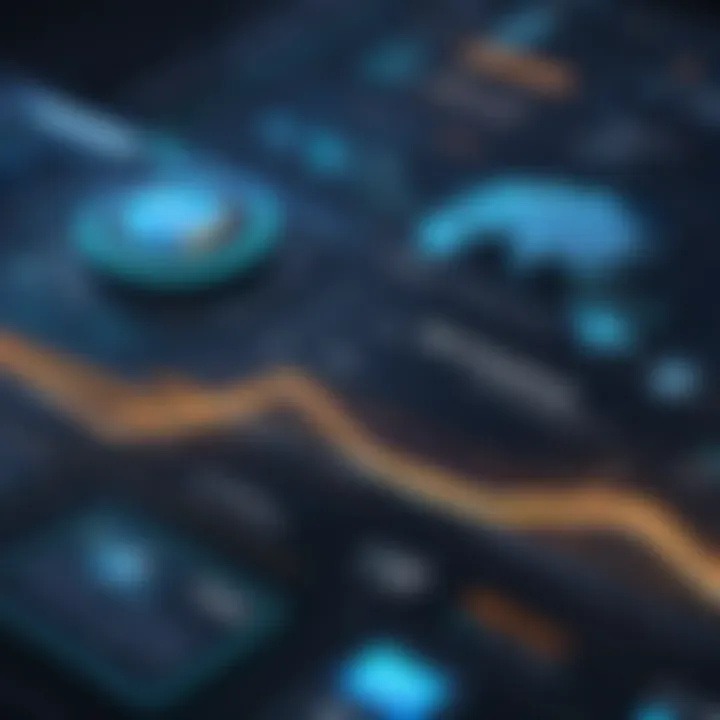
Intro
The landscape of business intelligence has undergone a metamorphosis, evolving from its rudimentary beginnings to the sophisticated systems we have today. Originally, organizations relied on simple reports and spreadsheet analysis to interpret data. But, as the complexities of global markets and consumer needs grew, so did the demand for more nuanced insights.
Business intelligence isn’t just a tech buzzword. It fundamentally shapes how decisions are made within companies. An analysis of its journey reveals significant trends and technological advancements, illustrating why understanding this evolution is crucial for anyone in the field. Recent developments, particularly the emergence of artificial intelligence and machine learning, have further transformed the scope of business intelligence, pushing it into uncharted territories.
In this exploration of business intelligence evolution, we will dissect not only the historical context but also the tools and technologies that have become pivotal in this sector. The role of software has been crucial, functioning as the backbone of data analysis and interpretation. As we navigate through this article, you’ll discover intricate details and insights that could propel organizations towards more effective decision-making and strategic initiatives.
Prologue to Business Intelligence
Business intelligence is more than just a buzzword; it's a fundamental aspect of how organizations navigate the complex waters of today’s market. In this section, we’ll peel back the layers on what exactly business intelligence means and explore its significance in the modern corporate landscape.
Defining Business Intelligence
At its core, business intelligence (BI) encompasses the tools and systems that play a key role in the strategic planning and decision-making processes of an organization. It involves analyzing business data to help companies make informed decisions. BI can include various practices and technologies – from data mining and process analysis to performance benchmarking and predictive analytics.
The definition of business intelligence obtained strength through its application in the real world. Consider this: a retail company utilizing BI might analyze purchasing trends to determine which products are most in-demand in specific demographics, thereby directing its marketing strategies more effectively. Essentially, BI helps organizations transform raw data into actionable insights, which can lead to substantial competitive advantages.
Importance in Modern Business
In today’s fast-paced environment, businesses can't afford to snake around with gut feelings or guesswork. The importance of business intelligence is underscored by its capacity to provide crucial insights that help to inform business strategy.
Here are some key benefits of implementing BI:
- Informed Decision Making: BI enables managers and executives to make data-driven decisions, reducing uncertainty and enhancing clarity.
- Increased Efficiency: Streamlined reporting processes save time and resources, allowing teams to focus on analyzing data rather than just collecting it.
- Identifying Market Trends: Companies can track evolving consumer behavior and trends, allowing them to adapt quickly and responsively.
- Improved Customer Insights: Understanding customer preferences and pain points helps businesses tailor their offerings more effectively.
In modern business, where changes happen swiftly, harnessing the power of comprehensive data through business intelligence means not only surviving but thriving. As organizations look to refine operations and enhance decision-making, the conversation around business intelligence becomes ever more essential.
Historical Context of Business Intelligence
Understanding the historical context of business intelligence is crucial for grasping how modern practice came about. It sheds light on the evolution of data management, the methodologies that shaped this field, and the subsequent impact on decision-making processes. By examining this backdrop, professionals can appreciate how far we’ve come and the challenges that have been faced along the way.
Early Data Management Practices
In the early days, data management was more of a rudimentary affair than what we see today. Businesses relied heavily on paper records and spreadsheets. Decision-making was often based on intuition or qualitative assessments rather than on quantifiable metrics.
For instance, a small retailer might keep track of inventory with pen and paper, making it difficult to view trends or forecast demand accurately. The limitations were staggering; choices were often made in the dark.
As businesses grew, so did the need for a more systematic approach to data. This led to the rise of databases. Relational databases became prominent in the 1970s, enabling companies to store information with better organization and retrieval methods. Instead of a chaotic mix of papers, data could be stored in structured formats. Despite their benefits, these early systems were still too complex for many organizations to navigate effectively.
The Birth of Business Intelligence
The concept of business intelligence began to take form in the 1980s as a response to these growing complexities in data management. The term itself was coined by Howard Dresner, an analyst at Gartner, who noted that this new focus was about using data to guide decisions effectively. BI aimed to integrate various data sources, providing a more holistic view of business performance.
In the 1990s, tools such as SAP Business Warehouse and Oracle BI began to emerge. These platforms offered organizations more sophisticated analytics capabilities. Businesses could not only store data but also generate insights from it, fueling competition.
"Business Intelligence is about transforming raw data into actionable insights."
As companies recognized the power of BI, investments in this area surged. Insights derived from data analytics started informing market strategies, operational efficiencies, and customer engagement initiatives. Organizations began to see the value in leveraging data adequately, marking a significant turning point in businesses’ operational strategies.
Today, business intelligence continues to grow and adapt, shaped by the historical practices that laid the groundwork. Understanding these origins provides essential context, especially for those working in software development and IT, as they grapple with the complexities and potential of modern BI tools. This historical journey illustrates not just an evolution of technology, but a transformation in how businesses view data itself.
Technological Advancements
The landscape of business intelligence (BI) has been greatly shaped by technological advancements, which have transformed raw data into actionable insights. These developments have not only streamlined data processes but also opened new avenues for analysis, thereby enhancing decision-making capabilities for organizations.
Data Warehousing Development
Data warehousing plays a cornerstone role in the realm of business intelligence. In essence, a data warehouse is a centralized repository that stores data from multiple sources, allowing businesses to perform complex queries and analysis without affecting the operational systems. This capability is fundamental for decision-makers who rely on accurate, timely information.
The transition from traditional databases to data warehouses marked a significant turning point. Earlier, data was often scattered across different systems. Consolidating that data into a single source of truth made it much easier for users to access and analyze. Today’s data warehouses leverage cloud technology, which brings several notable advantages:
- Scalability: Companies can scale their data storage without making hefty investments in physical infrastructure.
- Performance: Advanced data processing engines like Snowflake and Amazon Redshift allow for quick execution of complex queries.
- Integration: Data from operational systems, social media, and even IoT devices can be combined seamlessly, enriching the datasets available for analysis.
A pertinent example is how major retailers use data warehousing. They combine point-of-sale data with customer feedback from social media platforms to refine their inventory management. This integrated view provides them crucial insights into customer preferences, helping fine-tune marketing strategies and stock levels.
Emergence of Analytical Tools
The rise of analytical tools has further propelled the evolution of business intelligence. Tools like Tableau, Power BI, and QlikView empower users to visualize data in ways that were once unimaginable. These platforms allow even those without data expertise to generate reports and derive insights. Analytics has shifted from a backend function to a front-line capability, empowering employees across various functions to make data-driven decisions.
Moreover, the advent of self-service analytics is a game changer. Professionals don’t need to rely solely on the IT department to generate reports. With intuitive interfaces, users can create dashboards and manipulate data independently.
Key benefits of these tools include:
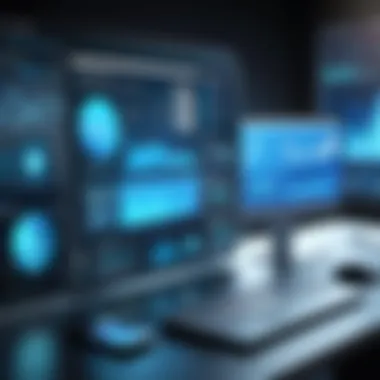
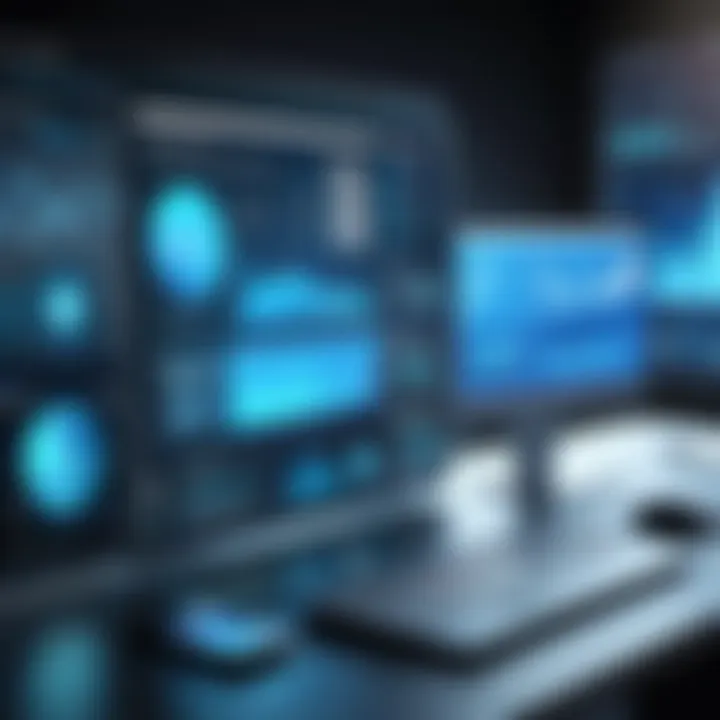
- User Friendliness: Drag-and-drop features make the experience accessible.
- Collaboration: Users can share insights easily, fostering a data-driven culture within organizations.
- Real-Time Insights: With the integration of live data feeds, businesses can respond quickly to market changes.
A striking illustration of this tool's impact is found in healthcare systems. By using tools like QlikView, institutions can visualize patient data, improve treatment plans, manage resources efficiently, and thus significantly enhance patient outcomes.
"The integration of analytical tools into business processes dramatically changes how companies understand their markets and internal operations."
In summary, technological advancements in data warehousing and analytical tools have fundamentally altered how businesses interact with data. These changes have ushered in a new era of insights and strategies that empower organizations to make informed decisions swiftly and effectively.
Trends Influencing Business Intelligence
As we navigate through the dynamic landscape of modern business intelligence (BI), it’s crucial to recognize the currents shaping its trajectory. This section delves into two significant trends influencing BI: the pervasive nature of big data and the advent of cloud computing. Understanding these phenomena is not just academic; it’s about positioning organizations for success in a data-driven world.
The Impact of Big Data
Big data is the widespread collection of data that is generated at an unprecedented scale and speed. The deluge of information presents both challenges and opportunities for businesses. Utilizing big data effectively can drive significant value. Here are several key aspects worth considering:
- Volume and Variety: Companies now collect data from myriad sources, ranging from customer interactions to IoT devices. This wealth of information, when processed correctly, can unveil insights that were previously unimaginable.
- Real-Time Analytics: With the right tools, businesses can analyze data as it streams in. This means decisions can be made swiftly, often in seconds or minutes, enabling companies to respond to market changes nimbly.
- Customer Insights: Analyzing large datasets allows firms to understand customer preferences and behavior at a granular level. Marketers can tailor campaigns, boosting engagement and conversion rates significantly.
Reflecting on the impact of big data is essential; many organizations are stuck in the past, relying on outdated methods. Adapting to big data isn’t merely an advantage; it’s a necessity.
"In the data-driven age, those who effectively harness the power of big data not only survive, they thrive."
Cloud Computing and BI
Cloud computing has emerged as a game-changer in the realm of business intelligence. By shifting resources to the cloud, organizations can enhance their BI capabilities dramatically, helping them stay agile and competitive. Here are several key benefits of integrating cloud computing into BI:
- Scalability: Cloud platforms allow businesses to scale their BI solutions effortlessly. Whether it’s the need for more data storage or increased processing power, the cloud can accommodate.
- Accessibility: With cloud BI tools, users can access information from anywhere, making it easier for teams to collaborate regardless of geographical barriers. This flexibility promotes a culture of data sharing and teamwork.
- Cost Efficiency: Traditional BI systems often require hefty upfront investments. Cloud solutions typically operate on a subscription model, which can significantly lower costs for organizations, especially small- to medium-sized enterprises.
Integrating cloud computing with BI opens the door to innovative solutions. The potential for collaboration, data integration, and real-time access transforms how decisions are made in modern business environments.
Artificial Intelligence and Machine Learning
In the landscape of business intelligence, the integration of artificial intelligence (AI) and machine learning (ML) marks a significant leap forward. These technologies transform how organizations gather, analyze, and utilize data. By incorporating predictive algorithms and automating processes, businesses can not only enhance operational efficiency but also make decisions that are informed by deep insights rather than gut feelings.
AI Integration in Business Intelligence
Bringing AI into the fold of business intelligence is more than just slapping a fancy label on data analysis. It’s about creating systems that learn from their data interactions and improve over time. This integration allows companies to sift through mountains of information and draw actionable insights without manual intervention. A prime example could be how retail organizations use AI to forecast inventory needs. By analyzing purchase patterns and external factors like seasonality, they can predict what products will be hot sellers before the first leaf falls.
Moreover, AI tools can process unstructured data—like customer feedback on social media or product reviews—much faster than traditional methods. This capability can give a business a pulse on public sentiment and inform strategies in real-time. For instance, if a software company notices a spike in negative feedback about a feature during a major update, it can pivot quickly to resolve issues before they escalate.
Machine Learning Applications
Machine learning applications offer multiple avenues for enhancing business intelligence strategies. Companies harness ML for tasks such as customer segmentation, fraud detection, and demand forecasting. One noteworthy application is customer segmentation. Here, machine learning algorithms analyze customer data to identify distinct segments based on behavior and preferences. This allows marketers to tailor their approaches for different groups, enhancing engagement and increasing conversion rates.
But it does not stop there. Fraud detection is another area where machine learning shines. Financial institutions deploy these algorithms to monitor transactions in real-time, identifying anomalous behaviors that could indicate fraud. When the system spots unusual patterns, alerts can be sent to relevant personnel immediately, making it possible to prevent losses before they occur.
Here's a simple outline of how machine learning can be applied within business intelligence:
- Customer Behavior Analysis: Understand and predict customer actions by analyzing past purchasing data.
- Churn Prediction: Identify customers likely to leave, allowing businesses to target retention efforts.
- Sales Forecasting: Use historical data to predict future sales trends and performance.
"Incorporating machine learning into business intelligence isn’t just about having the latest tech; it’s about making smarter decisions that pave the way for success."
Adopting these technologies is not without challenges, though. Organizations must ensure they have quality data and appropriate frameworks in place to derive meaningful insights. Missteps in implementation can lead to incorrect conclusions, which could steer a business in the wrong direction.
All in all, the combination of AI and machine learning in business intelligence provides powerful tools that enable companies to navigate the complexities of modern data environments effectively. They help in driving strategic initiatives and foster a culture of data-informed decision-making.
Challenges in Business Intelligence Evolution
In the realm of business intelligence (BI), challenges are as common as a rainy day in April. As organizations strive to tap into the vast reservoirs of data available to them, several key difficulties come into play. These hurdles can hinder the potential benefits offered by BI tools and approaches, necessitating a closer look at what these challenges entail and how businesses can navigate through them. Understanding these obstacles can be cardinal in shaping strategies that not only address current issues but also pave the way for a robust BI implementation.
Data Security Concerns
When it comes to data security, the stakes are high. In a world where breaches seem to be a frequent headline grabber, the importance of safeguarding sensitive information cannot be overstated. Companies must handle data with care, ensuring that they have adequate protections in place. It's vital for organizations not just to comply with regulations, such as GDPR or HIPAA, but also to foster trust among their stakeholders.
- Potential Risks: With increasing digital transformation efforts, vulnerabilities can arise. Cyber attacks, insider threats, and even accidental data leakage form a triad of risks, and organizations must be prepared to counter these threats.
- Protective Measures: Implementing encryption, access controls, and regular audits can help mitigate these risks. Often, employees are the weakest link in the security chain, so ongoing training on security protocols is essential.
The challenge doesn't stop at merely implementing measures; it extends to maintaining them. Businesses need to stay ahead of emerging threats, necessitating a shift towards proactive rather than reactive security strategies. The bottom line here is clear: if organizations fail to prioritize data security, the ramifications can be severe, leading to financial loss and tarnished reputations.
Quality of Data Management
"Garbage in, garbage out" – a saying that carries weight in business intelligence. The quality of data directly affects the insights that can be drawn from it. Poor data management struggles can result in incorrect conclusions, which can lead to misguided strategies. High-quality data management is like a sturdy foundation for a skyscraper; it bears the weight of decisions and strategies built on top of it.
- The Essence of Quality Data: Quality encompasses accuracy, completeness, consistency, and timeliness. A dataset may be expansive, but if the information is dubious or outdated, it becomes a minefield for decision-makers.
- Strategies for Improvement: To boost data quality, organizations could establish clear data governance policies. Regular data cleaning processes can aid in identifying and correcting inconsistencies, ensuring that the data remains reliable. Collaborative efforts across departments can also enhance data collection and management strategies.
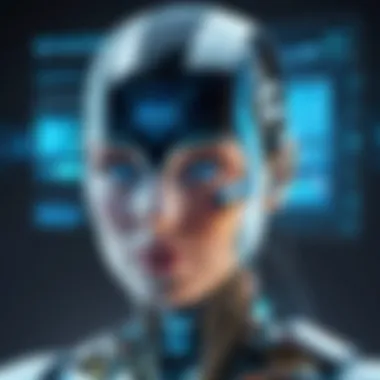
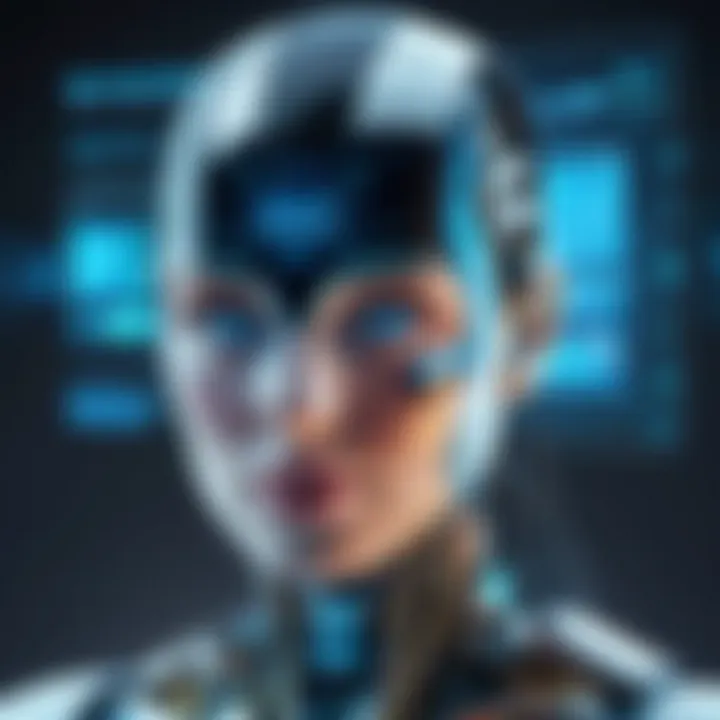
In a scenario fraught with misinformation, businesses can easily find themselves at a crossroads, unsure of which path to take. The challenge lies in not just collecting data but managing it in a way that elevates its intrinsic value. If approached correctly, a robust data management framework can lead to more accurate analytics and better decision-making, transforming potential challenges into a competitive edge.
"Effective data security and quality management aren’t just optional; they’re required for a successful business intelligence strategy."
By confronting these challenges head-on, organizations position themselves not just to survive but thrive in a data-driven world. Ensuring data security and quality management are paramount for businesses looking to gain traction in an increasingly competitive landscape.
Future Directions of Business Intelligence
As organizations adapt to the rapidly changing landscape, the future directions of business intelligence (BI) emerge not just as predictions, but as a roadmap for the survival and growth in the competitive marketplace. Understanding how BI will evolve is crucial for decision-makers and tech enthusiasts alike. In this section, we will explore significant trends like predictive analytics and augmented intelligence, shedding light on their transformative potential.
Predictive Analytics and its Role
Predictive analytics has emerged as a prominent tool in the toolkit of modern business intelligence. At its core, predictive analytics employs historical data patterns to forecast future outcomes. This capability is invaluable for firms aiming to stay a step ahead, helping them make informed decisions based on projected trends.
- Enhanced Decision-Making: Organizations can navigate risk better by using predictive models to analyze customer behavior, market shifts, and supply chain variables.
- Resource Optimization: By anticipating future demands, businesses can optimize their resource allocation, ensuring that they are not over or under-investing in crucial areas.
- Customer Insights: Understanding potential customer preferences allows businesses to tailor offerings, enhancing customer satisfaction and loyalty.
Think of it like having a crystal ball; predictive analytics lets companies glance into the future. Imagine a retail company using past purchasing trends to decide on stock levels for an upcoming season or a healthcare provider predicting patient inflow based on various demographics. The implications are wide-reaching.
Augmented Intelligence Trends
While artificial intelligence (AI) often takes the spotlight, augmented intelligence is a rising star in the business intelligence arena. This concept refers to the collaboration between human intelligence and AI, creating a synergy that enhances decision-making processes rather than replacing human insight.
- Empowering Human Analysts: Augmented intelligence tools do not solely depend on algorithms; they assist human analysts with insights that would be difficult to ascertain through sheer data analysis alone.
- Data-Assisted Strategies: The incorporation of augmented intelligence into BI means that organizations can craft strategies informed by data but still rooted in human creativity and intuition.
- Continuous Learning: These tools adapt as they learn from human interactions, becoming increasingly efficient at predicting outcomes and offering actionable insights over time.
To sum it up, augmented intelligence allows businesses to fully harness their data by combining human creativity with AI’s computational strength. It’s like having a seasoned partner who takes care of the numbers while you focus on innovative strategies.
"The future of business intelligence is not just about the data we collect, but the wisdom we ignite from it."
The Role of Business Intelligence in Decision Making
In today’s fast-paced business world, the significance of business intelligence (BI) in decision-making cannot be overstated. It acts as the scaffolding that supports organizations in navigating complex data landscapes and harnessing insights that propel growth. Effective decision-making relies heavily on accurate data, timely analysis, and clear visualization of information. Consequently, BI tools have become invaluable assets for decision-makers, offering both clarity and a competitive edge.
Enhancing Strategic Planning
Business intelligence fortifies strategic planning by offering data-driven insights that align with organizational goals. When companies weave BI into their strategic processes, they gain access to real-time data which supports informed deliberation on key initiatives. This is especially crucial in areas like market analysis, resource allocation, and forecasting future trends.
- Market Analysis: Understanding market dynamics is critical. With BI, companies can analyze consumer behaviors and market trends. For instance, a retail chain can utilize sales data and social media analytics to anticipate product demand, ensuring inventories are aligned with customer needs.
- Resource Allocation: BI tools enable organizations to allocate resources more effectively. Real-time insights help identify which departments are performing well and where bottlenecks occur. For instance, if analytics show that a particular marketing campaign is underperforming, resources can be reallocated quickly to projects with better potential returns.
- Forecasting: Leveraging past data to predict future trends is where BI shines. It equips decision-makers with critical information about potential market changes, demographics shifts, or economic conditions. With this predictive power, strategic planning becomes not just reactive, but proactively anchored in data.
"In a data-driven world, organizations that fail to leverage business intelligence miss opportunities to excel."
Real-time Reporting and Insights
One of the standout features of business intelligence is its capacity for real-time reporting. This characteristic distinguishes BI from traditional reporting methods which often lag behind. Decision-makers benefit immensely from having immediate access to relevant data, allowing them to act promptly rather than relying on historical data that might be outdated.
- Time-sensitive Decisions: In dynamic markets, timing is everything. Real-time insights help businesses react to changing conditions instantly. For example, a financial services firm assessing market volatility can make timely trades based on the latest data, minimizing losses or capitalizing on profit opportunities.
- Performance Monitoring: Continuous monitoring of key performance indicators (KPIs) allows organizations to stay on top of their performance metrics. For instance, an airline can use BI tools to track on-time performance and customer satisfaction scores. Should issues arise, management can take immediate corrective measures, thus maintaining operational efficiency.
- Collaboration and Transparency: BI encourages a culture of collaboration. When reports and dashboards are accessible to all stakeholders, it fosters transparency in decision-making. Cross-departmental teams that utilize shared insights will derive comprehensive understanding, leading to more cohesive strategies and execution.
Through strategic planning enhancements and real-time reporting, business intelligence molds the decision-making landscape. Organizations that embrace these practices not only thrive in their operational efficacy but also cultivate a culture of informed choices, leading to sustained success.
Best Practices for Implementation
When organizations decide to adopt business intelligence (BI) solutions, it's akin to setting sail on uncharted waters. Navigating through the complex sea of data requires an efficient implementation strategy. Having best practices in place ensures that the implementation process is smooth, and it significantly boosts the chances of realization of the desired outcomes. S likely consider the particular needs of the organization while planning and execution. Here are some pivotal aspects to bear in mind:
- Alignment with Business Goals: The initial step in any BI implementation should be to ensure that the strategy is aligned with the core business objectives. This involves understanding what the organization wants to accomplish using BI, whether it's enhancing customer satisfaction or improving operational efficiency. Without this alignment, the BI initiative may end up being just another costly endeavor.
- Stakeholder Engagement: Getting buy-in from key stakeholders throughout the organization is crucial. This means regularly involving individuals who will be using the BI tools, such as data analysts and executives. Their insights can provide valuable feedback and help tailor the BI setup to meet their expectations.
- Data Quality & Governance: Implementing BI without addressing data quality is like building a house on a shaky foundation. Organizations must ensure that the data they feed into the BI system is clean, accurate, and governed properly. Establishing data governance protocols can help mitigate issues that arise from poor data quality down the line.
- Iterative Approach: Rather than attempting to implement everything at once, an iterative approach allows for gradual integration. Starting with a pilot program can enable organizations to refine their processes based on real feedback before rolling out on a larger scale.
- Investing in Training: Proper training is the bedrock of successful BI adoption. It’s not enough to introduce new tools; users must know how to leverage these tools effectively. A well-structured training program will empower users and make them comfortable.
"In the world of business intelligence, it's not just about having the right tools; it's about ensuring people know how to use those tools effectively."
- Choosing the Right Technology Stack: It's critical to select the right BI tools that are not only compatible with existing systems but also flexible enough to grow with the organization. Evaluating options based on scalability, usability, and community support can help avoid future headaches.
These best practices create a robust framework to guide teams through the choppy waters of BI implementation. With these principles in mind, any organization can ensure that it is well-equipped to face the challenges and harness the full potential of business intelligence.
Choosing the Right Tools
Choosing the right tools for business intelligence is a cornerstone of any successful implementation. Organizations have to sift through a plethora of options before settling on the tools that best fit their unique requirements.
A few things to keep at the forefront are:
- User-Friendliness: If the tools are complex and hard to navigate, very few users will leverage them effectively. The best BI tools come with intuitive interfaces that simplify user interaction, allowing teams to focus more on analysis than on technical hiccups.
- Integration Capabilities: Effective BI systems are designed to play well with other software environments. The ability to integrate seamlessly with existing CRM systems, databases, or ERP tools can drive efficiency and ensure that data flows freely.
- Cost-Effectiveness: This doesn’t merely mean finding the cheapest option. Organizations need to evaluate the total cost of ownership which often includes licensing fees, maintenance costs, and potential costs related to training and support.
- Scalability: As businesses grow, their data needs will evolve. Tools that effectively scale are crucial for ensuring that BI capabilities remain robust as the organization expands.
Ultimately, the right tool should not just meet immediate needs but serve as a fruitful investment that yields value over the long haul, ensuring sustainable growth and adaptability to future changes.
Training and Support for Users
When it comes down to actually using business intelligence tools, the importance of training and support cannot be overstated. It’s akin to teaching someone to ride a bike; without sufficient practice and guidance, even the smartest innovations can become useless.
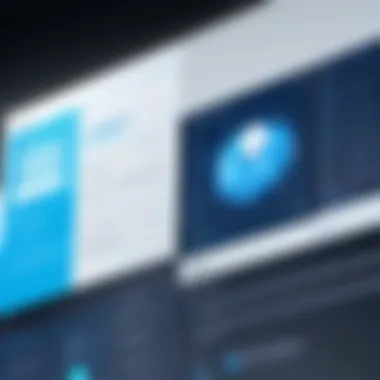
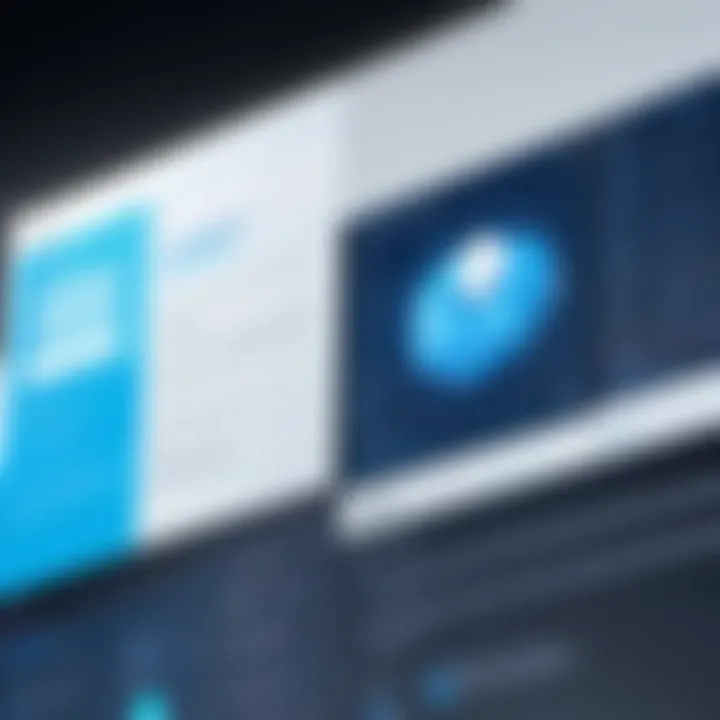
To maximize the potential of BI, organizations must:
- Offer Comprehensive Training: A one-size-fits-all training session simply won’t cut it. Tailoring training programs to various skill levels and user groups ensures that everyone—from novice to expert—can gain confidence in using BI tools.
- Establish a Support System: After training, ongoing support is essential. Whether through creating user communities, appointing help desks, or online resources, providing a support system fosters an environment where users can continually share insights and ask questions.
- Encourage Knowledge Sharing: Daily challenges and solutions can often reveal best practices or new ways of utilizing BI tools. Encouraging users to share their experiences makes the learning process ongoing and dynamic rather than static.
Overall, investing in training and support is not an additional expense but a strategic move to ensure that your organization unleashes the full power of business intelligence tools, driving effective decision-making and fostering a culture of data-driven insights.
Case Studies in Business Intelligence
Case studies in business intelligence (BI) are crucial to understanding its practical impact and application within various organizations. Through these real-world examples, businesses can mirror the strategies and outcomes displayed by others, learning not only from successful implementations but also from instances where things didn’t quite go as planned. A comprehensive examination of these cases reveals the multifaceted advantages of BI, offering insights that can lead to smarter decisions in the future.
Successful BI Implementations
Organizations that have successfully harnessed BI tools have often reported remarkable improvements in their operational efficiency and decision-making processes. One notable case is that of Netflix, which utilizes data analytics to anticipate viewer preferences and optimize content recommendations. This proactive approach not only enhances user experience but also significantly boosts retention rates.
Another striking example is Amazon, which employs predictive analytics to tailor marketing efforts, manage inventory effectively, and optimize logistics. The integration of business intelligence in such firms has contributed to their ability to adapt rapidly to market trends and customer needs while maintaining a competitive edge.
Key elements of successful BI implementations generally include:
- Clear Objectives: Establishing what the organization hopes to achieve with BI.
- Data Governance: Ensuring data quality and compliance throughout the organization.
- User Training: Preparing staff to effectively utilize BI tools.
- Iterative Approach: Emphasizing continuous improvement.
Lessons Learned from Failures
While many companies have navigated the BI landscape successfully, others have faced significant challenges. One well-known example is J.C. Penney, which underwent a major BI initiative aimed at redefining its pricing strategy. However, the company’s failure to properly analyze customer data led to an alienation of its core customer base, resulting in plummeting sales. This misstep underscores the importance of aligning BI strategies with customer expectations.
Another cautionary tale is that from Target, which faced criticism for its approach to data privacy and customer tracking. Although it used BI to personalize marketing campaigns, an oversight in customer segmentation led to a backlash when customers felt their privacy was invaded.
The lessons learned from these failures include:
- Understand Your Audience: The necessity of aligning BI strategies with customer needs and preferences.
- Robust Data Analysis: Ensuring thorough analysis to minimize risks associated with misinterpretation of data.
- Ethical Considerations: The critical nature of ethical data usage practices to maintain trust and credibility.
"Even in failure, there lies a wealth of information that can drive future successes."
In summary, examining case studies in business intelligence provides invaluable insights into both effective practices and pitfalls to avoid. These real-world examples highlight the evolving nature of BI and underscore the importance of thoughtful strategy formulation and implementation in driving organizational success.
The Competitive Advantage of BI
In today’s fast-paced business environment, organizations are increasingly realizing that having a robust business intelligence (BI) strategy can set them apart from their competitors. The capacity to analyze data effectively gives firms an edge, enabling them to make informed decisions swiftly. Let’s delve into key aspects that showcase the competitive advantage of BI.
Driving Business Success
With the explosion of data available, companies are tasked with not only collecting this data but also converting it into actionable insights. BI tools empower organizations to visualize their data in an intelligible manner. This aspect is crucial for driving business success. For instance, a retail company can analyze customer purchasing patterns and determine which products are gaining traction during a particular season. This alignment allows businesses to offer promotions tailored to consumers’ preferences, thereby increasing sales.
Furthermore, BI solutions can enhance operational efficiencies. By identifying bottlenecks in processes through data analytics, companies can streamline operations. For example, a manufacturing firm using BI analytics might discover that a specific machine is causing delays. Addressing this issue not only saves time but also cuts down costs, leading to enhanced profitability.
"Without data, you're just another person with an opinion." – W. Edwards Deming
This quote underscores the importance of data-driven decision-making in driving business success. Adopting BI enables companies to go beyond basic reporting, diving deep into predictive analytics and forecasting.
Market Responsiveness and Adaptation
The business world is dynamic, subject to rapid changes due to consumer behavior shifts, technological innovations, or regulatory updates. Here, the agility provided by BI tools is invaluable. Companies that can quickly adapt to market changes are the ones that thrive.
For instance, during the recent pandemic, many businesses struggled to adjust to sudden changes in consumer behavior. Companies equipped with effective BI tools managed to pivot quickly, tracking real-time data about consumer trends. They could analyze which products were in demand, reconfigure their supply chains, and adjust marketing strategies accordingly.
Moreover, incorporating BI fosters a culture of continuous improvement. When data is at the heart of decision-making, it encourages teams to test various approaches and learn from their outcomes. This iterative process ensures that organizations not only keep pace with their competitors but can lead in innovation and strategy.
Summary and End
In the realm of business intelligence (BI), the culmination of advancements, challenges, and future possibilities serves as a vital reference for organizations and professionals alike. This summary encapsulates the essential themes discussed throughout the article while anchoring the relevance of BI in today’s data-driven landscape.
The journey of BI reflects a shift from rudimentary data collection methods to sophisticated analytical frameworks embracing artificial intelligence and machine learning. This evolution is not merely a technical development; it signifies a cultural shift within organizations, urging them to harness data as a strategic asset for decision-making.
Recapping the Evolution Journey of BI
The evolution of business intelligence isn't a linear path but rather a complex tapestry woven from various innovations and uses. Early on, companies relied heavily on manual processes, which were prone to error and inefficiency. Over the decades, the introduction of data warehousing and analytical tools transformed how businesses approached data assimilation.
"Data is the new oil; it’s valuable, but if unrefined, it cannot really be used."
Key milestones in this journey include:
- Early Data Management Practices: From using ledgers and spreadsheets to implement systematic data management systems.
- Emergence of Analytical Tools: The introduction of tools like Tableau and Microsoft Power BI revolutionized reporting, enabling better visualization and interpretation of data.
- AI and Machine Learning Integration: Organizations began utilizing predictive modeling, optimizing outcomes in ways that were previously unimaginable.
In hindsight, the evolution of BI can be seen as a continuous pursuit to extract actionable insights from data, pushing businesses towards a more informed and strategic frontier.
Future Outlook for Business Intelligence
Looking ahead, the future of business intelligence seems bright and vibrant with potential. Innovations in predictive analytics and augmented intelligence are setting the stage for greater engagement with data. Here’s what we might expect:
- Enhanced Predictive Analytics: This technology will likely empower organizations to anticipate market trends, identifying opportunities and threats with greater precision.
- Integration of Cloud Computing: Cloud technologies will continue to facilitate real-time data accessibility and collaboration across teams.
- Augmented Intelligence Trends: This approach emphasizes human-machine collaboration, where tools enhance human capabilities rather than replace them. In this context, the development of user-friendly interfaces may emerge as a critical focus in future BI platforms.