Explore Data Science Courses on Udemy: A Complete Guide

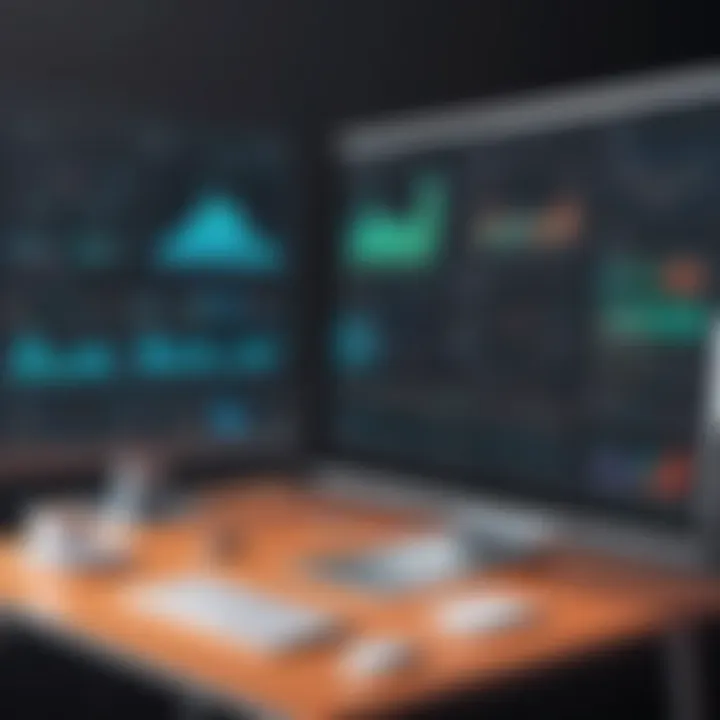
Intro
In today's data-drivn world, the demand for data science skills has skyrocketed. As budding data scientists take their first steps into this expansive field, the question arises: where to start? Udemy, an online learning platform renowned for its extensive array of courses, holds a treasure trove of educational opportunities. From introductory classes in Python and R to specialized training in machine learning and predictive analytics, finding the right course can feel like searching for a needle in a haystack.
This article aims to provide a thorough exploration of the data science courses available on Udemy. We're not just skimming the surface; we'll delve into the nitty-gritty of course content, assess the caliber of instructors, scrutinize learning outcomes, and take a close look at user feedback. All these facets help prospective students make informed choices about their educational endeavors. By assessing various factors including pricing, certification options, and accessibility, the aim is to empower readers with insights necessary for selecting the best course tailored to their needs.
Navigating the world of data science can seem overwhelming. However, this guide will strive to illuminate the path toward achieving your career goals in this rapidly-evolving domain.
Intro to Data Science
Data science, often dubbed the "new oil" of the 21st century, is a dynamic field that combines statistical analysis, data manipulation, and machine learning to extract meaningful insights from vast datasets. It’s not merely about crunching numbers; it’s about turning raw data into actionable intelligence that can inform decisions, drive innovation, and create value. The relevance of the subject matter in today's context cannot be overstated, especially given the surge of data generated daily from various sources, including social media, IoT devices, and business transactions.
Understanding Data Science
At its core, data science is an interdisciplinary field that employs techniques from mathematics, statistics, and computer science. Think of it as a bridge connecting the domain of rigorous analytics with practical business needs. Data scientists don’t just analyze data; they also ask the right questions, identify patterns, and communicate their findings in a way that’s understandable to stakeholders. The process typically includes several stages:
- Data Collection: Gathering information from diverse sources, whether structured or unstructured.
- Data Cleaning: Prepping the data for analysis, which often involves fixing inaccuracies and eliminating irrelevant information.
- Data Exploration: Conducting preliminary investigations to find patterns or anomalies.
- Modeling: Using algorithms to predict outcomes or generate insights.
- Evaluation: Assessing the model's effectiveness and determining its actual utility in real-world applications.
To navigate this landscape, aspiring data scientists must focus on mastering tools like Python, R, SQL, and various data visualization libraries. These tools aid in transforming complex data into understandable formats. This skills framework sets the stage for deeper dives into the specialized courses offered on platforms like Udemy.
Importance of Data Science in Today’s World
In the contemporary business ecosystem, organizations are drowning in data yet starving for insights. This scenario underscores the importance of data science across different sectors. Data-driven decision-making allows companies to enhance efficiency, predict market trends, and ultimately, foster innovation. Consider the following points:
- Competitive Advantage: Businesses leveraging data science can identify opportunities faster than their rivals, enabling them to adapt their strategies accordingly.
- Customer Insights: Through detailed data analyses, organizations can glean insights into customer behavior, leading to personalized service that enhances customer loyalty.
- Operational Efficiency: Data science streamlines processes by identifying bottlenecks and areas for improvement, ultimately reducing costs.
- Innovation: By recognizing patterns from past data, businesses can innovate their products and services, thereby staying ahead in competitive markets.
"Without data, you're just another person with an opinion." – W. Edwards Deming
In summary, understanding data science not only equips individuals with a crucial skill set but also enables them to make significant contributions to their organizations. As future sections will detail prospective courses on Udemy, it’s clear that this knowledge holds immense potential to empower today's learners and professionals in shaping their careers.
Overview of Udemy as an Educational Platform
Exploring Udemy as an educational platform is crucial for understanding the broader landscape of online learning and how it pertains to data science courses. The rise of digital education has reshaped how professionals acquire skills, with Udemy standing out due to its vast course offerings and unique approach. This section will delve into its journey, addressing its target audience and the variety of courses that cater to different learning needs.
History and Development of Udemy
Udemy was founded in 2010, initially as a platform aimed at enabling anyone to create, teach, and learn from online courses. Over the years, it has evolved significantly, embracing new technologies and expanding its course catalog to include over 150,000 courses in various fields. Its mission has been clear: democratizing education for everyone, everywhere. As a result, the platform has attracted a massive global audience, now exceeding 50 million students.
From its humble beginnings, Udemy faced the challenge of establishing a standardized quality of education. To tackle this, they developed systems to vet courses and instructors, ensuring that students receive quality learning experiences. This trajectory shows just how vital Udemy has become in modern education, particularly in tech and data science domains, where rapid advancements demand ongoing learning.
Target Audience and Course Variety
Udemy primarily appeals to a broad spectrum of learners—from beginners eager to start their journey in data science to seasoned professionals aiming to upskill. This adaptability is a key selling point. Whether you're a software developer, an IT aficionado, or a curious student, the range of courses ensures that there’s something for everyone.
Within the data science realm, you can find courses that cover:
- Fundamentals of Data Science: Ideal for people just stepping into the field. These courses often start from the ground up, introducing basic concepts in data analysis and statistics.
- Advanced Techniques: Aimed at those familiar with the basics. Here, courses delve into machine learning, deep learning, and complex data visualization techniques using tools like Python and R.
- Project-Based Courses: These offer a hands-on approach, allowing learners to complete actual projects using real datasets.
Additionally, Udemy’s course variety is quite expansive, showcasing several data science sub-disciplines including data analytics, big data, and natural language processing. The variety keeps learners engaged, enabling them to pursue specific interests or skills relevant to their career goals.
"With a unique blend of practical and theoretical learning paths, Udemy effectively bridges the gap for learners aiming for industry competitiveness and relevance."
In summary, understanding Udemy's historical foundation and its purpose in today's educational landscape provides valuable context for prospective students. With its diverse course offerings, the platform grants access to essential learning opportunities as data science continues to evolve in an ever-changing technological landscape.
Types of Data Science Courses Available on Udemy
When exploring the landscape of data science education on Udemy, it’s crucial to understand the various course types offered. This isn't just about collecting a laundry list of options; it's about comprehending how these different courses can align with your individual learning goals and career aspirations. Each type of course carries unique benefits, covering a spectrum from foundational knowledge to specialized skills, ultimately shaping the paths available to learners in the data science field.
Introductory Data Science Courses
For those just stepping into the vast universe of data science, introductory courses serve as essential launchpads. These offerings often cover the fundamental concepts, techniques, and tools in an approachable manner. Imagine walking through the door into a new world, hand in hand with a knowledgeable guide. These courses typically include:
- Basics of statistical analysis
- Introduction to programming languages like Python or R
- Understanding of data visualization tools
- Foundational data wrangling methods
The beauty of these courses lies in their ability to break complex topics down, making them digestible for beginners. They’re designed to build confidence and provide a strong starting point for individuals who aspire to delve deeper into data science later.
Specialized Data Science Tracks
Once you have a handle on the basics, specialized tracks can elevate your expertise to a whole new level. These courses target specific domains within data science, such as:
- Machine Learning
- Deep Learning
- Natural Language Processing (NLP)
- Data Engineering
Specialized tracks mean diving deep into particular skills or methodologies that are critical to succeeding in today's competitive job market. They cater to learners who wish to niche down and gain proficiency that can set them apart from their peers. It’s like picking a specialty dish in a restaurant; you see the menu and decide to focus on honing the flavors of your chosen meal.
Project-Based Learning Options
Project-based courses introduce a hands-on approach to learning, allowing students to apply the concepts they’ve learned in real-world scenarios. Instead of simply watching lectures and memorizing information, learners work on practical projects, gaining experience that is invaluable when pursuing employment. These often include:
- Real datasets for analysis
- Completing projects that companies might undertake
- Capstone projects that synthesize knowledge gained throughout the course
Engaging with projects not only helps solidify your understanding but also gives you tangible deliverables to showcase in your portfolio. This experience is crucial as employers often look for candidates who can demonstrate practical application of their knowledge.
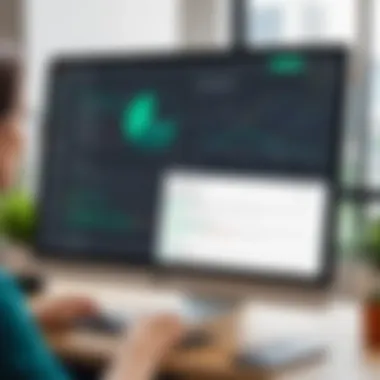
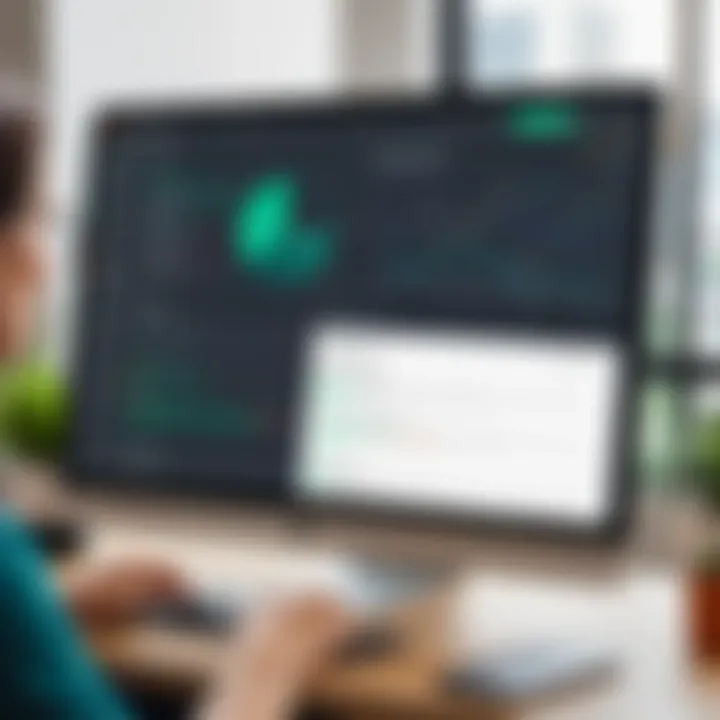
Evaluating Course Content
Evaluating the course content is crucial when considering any educational program, and it's especially important for data science courses on Udemy. Given the vast offerings available, discerning which course meets your objectives can be a daunting task. Proper evaluation involves looking into several factors that collectively shape the learning experience. By thoroughly assessing these aspects, prospective students can ensure they invest their time and resources into a course that delivers value and relevance to their career aspirations.
Curriculum Structure
The curriculum structure often serves as the backbone of any online course. A well-organized curriculum can set a clear path for learners, guiding them progressively through complex topics.
When looking at data science courses, consider:
- Logical Progression: Does the course follow a clear sequence? Beginning with fundamental concepts before advancing to complex subjects is essential.
- Module Breakdown: Examine how topics are divided into modules or sections. A balanced distribution allows learners to digest information without feeling overwhelmed.
- Duration and Depth: Each module should have an appropriate length that appropriately covers the subject matter. Too brief could leave gaps in knowledge, while overly long may lead to fatigue.
This structured approach allows students to absorb material systematically, enhancing retention and understanding.
Tools and Technologies Covered
A distinguishing feature of any data science course is the tools and technologies it introduces. Data science stands at the crossroads of programming, analytics, and big data. Thus, familiarity with relevant software and languages is paramount for practical application in real-world scenarios.
Here are key aspects to keep in mind:
- Programming Languages: Commonly taught languages include Python and R. It's valuable to know which languages are emphasized, as familiarity with them can open doors to various job roles.
- Data Analysis Tools: Look for courses that incorporate tools like Pandas, NumPy, or even Excel for data manipulation. Understanding these tools can significantly enhance analytical skills.
- Visualization Software: Courses that introduce visualization tools such as Tableau or Matplotlib are beneficial. Data visualization is crucial for presenting insights effectively.
Gaining hands-on experience with these technologies ultimately prepares students for industry challenges and boosts their employability.
Hands-On Exercises and Assignments
No course is complete without hands-on exercises; practical engagement is the magic ingredient that transforms theory into practice. In data science, where concepts often intersect, applying knowledge is vital.
Look for:
- Real-World Projects: Projects that solve actual problems can solidify learning. They allow students to synthesize their skills in a comprehensive manner, facilitating deeper understanding.
- Interactive Quizzes: Regular quizzes keep students accountable. They not only reinforce learning but also provide immediate feedback on their grasp of the concepts.
- Peer Reviews: Courses that encourage collaboration through peer reviews can enhance the learning experience. They promote critical thinking and expose students to diverse problem-solving approaches.
"Engagement through practical exercises can vastly enhance your learning journey, making it more relatable and impactful."
With these hands-on elements, learners are more likely to develop a robust skill set that stands out in a competitive job market.
Instructor Qualifications
In the domain of data science, the role of the instructor cannot be overstated. Having a qualified instructor can significantly impact the learning experience, shaping the students’ comprehension and practical skills. In a field that is constantly evolving, students must learn not just theoretical concepts but also how to apply them to real-world problems. This underscores the importance of instructor qualifications when choosing a course.
Identifying Experienced Instructors
When searching for a data science course on Udemy, it is crucial to identify instructors who bring real-world experience to the table. An instructor with a strong professional background is more likely to provide insights that go beyond textbook knowledge:
- Relevant Work Experience: Instructors with hands-on experience in data science roles can share strategies that have proved effective in their previous jobs.
- Industry Recognitions: Look for instructors who have been recognized in data science competitions like Kaggle or have contributed to significant projects in the field. Their credentials can lend credibility to their teaching.
- Educational Background: While degrees aren’t everything, instructors with advanced degrees in data science, computer science, or related fields typically have a solid understanding of the concepts necessary for teaching.
By thoroughly vetting instructor backgrounds, students can ensure they're learning from professionals who have walked the walk, not just talked the talk.
Instructor Ratings and Reviews
One important aspect of choosing a course is the feedback provided by past students. Ratings and reviews can offer a window into an instructor's teaching style and overall effectiveness. Here’s how to analyze them:
- Aggregate Ratings: Pay attention to the overall course ratings. Generally, courses with ratings over 4.5 stars can be a good bet, signifying a level of satisfaction among previous learners.
- Comments and Feedback: Look for specific comments about the instructor. If multiple reviews mention how engaging or informative the instructor is, this can indicate a positive learning experience.
- Response to Feedback: Some instructors engage with students through Q&A sections. This responsiveness can enhance learning, as it shows an instructor’s commitment to their students.
"A good instructor can turn complex data science theories into practical knowledge that one can easily comprehend and apply."
In summary, instructor qualifications play a pivotal role in determining the quality of a data science course. An experienced and well-rated instructor can provide invaluable insights, enhance the learning process, and ultimately equip students with skills that are vital in their professional journeys.
User Experience and Feedback
When it comes to online learning, especially in a field as complex as data science, the user experience can make all the difference. It encapsulates how learners interact with the platform, course content, and overall educational framework. Quality user experience is paramount as it fosters engagement, retention, and successful completion. In this section, we’ll explore two critical facets of user experience and feedback: student testimonials and completion rates.
Student Testimonials
Student testimonials are like gold in the world of online courses. They offer potential learners real-life insights into the experience of prior students. Positive testimonials can serve as a glowing endorsement for a course, giving newcomers confidence in its value. Here’s why diving into testimonials is essential:
- Authenticity: Real stories from past students bring an element of honesty. For example, a student might share how a specific course helped them land a job as a data scientist at a well-known tech company. Such narratives can be more persuasive than any marketing material.
- Diversity of Experience: Different learners have unique backgrounds and learning styles. Testimonials reveal how a course meets varied needs, be it for beginners or advanced learners. A comment like "This course helped me transform my career from marketing to data analytics" tells prospective students that the course has broad applications.
- Benchmarking Expectations: Reading what others found beneficial—or lacking—helps new students set their expectations. A consistent remark about a course being intensive yet rewarding can shape a learner’s approach.
"I was skeptical at first, but this course exceeded my expectations. The hands-on projects were invaluable!"
— Past Student
Completion Rates and Satisfaction Levels
Completion rates are a direct measure of how many students finish a course, and satisfaction levels indicate how happy they are with their learning journey. These metrics are crucial when weighing your options.
- Course Retention: High completion rates often signal that a course is engaging and well-structured. If a particular data science course boasts a completion rate of over 80%, you can bet there’s something worth sticking around for. Conversely, a low completion rate might raise red flags. You should inquire into course updates or instructor engagement.
- Student Surveys: Many platforms gather student feedback through surveys. Reviews mentioning course organization, clarity, and instructor responsiveness are key indicators. For instance, students saying that "The instructor was always available for questions" attests to an engaging learning environment.
- Satisfaction Metrics: Most students expect a solid ROI from their investments. Beyond just fun and engaging content, they look for actionable knowledge. Satisfaction levels can often be measured in follow-up surveys, where high marks indicate strong course value.
Pricing and Payment Options
Understanding the pricing and payment options of online courses can make or break a student’s educational journey. This section digs into how Udemy manages its pricing strategy, which is particularly relevant for those eyeing data science courses. With various factors like course duration, instructor reputation, and demand influencing costs, prospective students have to navigate this terrain wisely.
Understanding Udemy’s Pricing Model
Udemy operates on a dynamic pricing model, offering a mix of free and paid courses. For many data science enthusiasts, this is a double-edged sword. Courses priced at modest rates can be very accessible but may also prompt questions about the quality of the content.
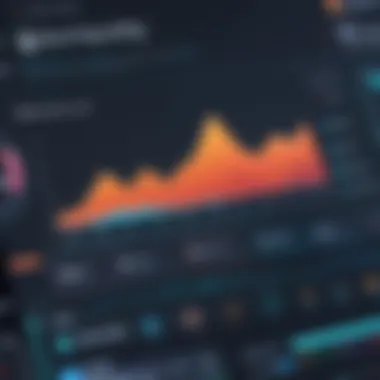
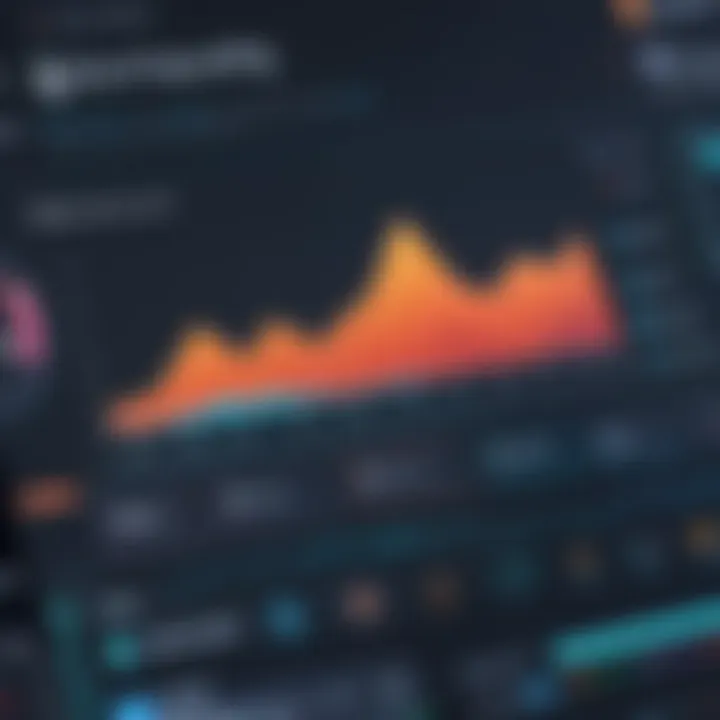
Generally speaking, most data science courses hover around the $10 to $200 mark. It’s essential to note that the pricing can fluctuate due to frequent sales and promotional events. Some courses are often marked down steeply during these sales, and this makes it possible for students to grab quality courses without breaking the bank.
- Regular pricing vs. sale prices can vary significantly—sometimes up to 90% off.
- Each course has a set base price, but instructors have the flexibility to adjust it accordingly.
- To make the most out of Udemy, keep an eye on course pages and notifications for updates on price drops or flash sales.
Moreover, Udemy provides a 30-day money-back guarantee on all paid courses. This safety net is especially comforting for those who are unsure about the course content or the teaching style. Using this option means you can try a course risk-free, giving you the chance to fully assess its value before committing long-term.
Discounts and Promotions
One of the standout features of Udemy’s pricing strategy is the abundance of discounts and promotions available throughout the year. This is where savvy students can truly benefit, especially those on a budget.
Udemy runs various promotional events, such as:
- Seasonal sales during holidays, Black Friday, and New Year.
- Regular flash sales, often promoted through email newsletters or social media.
- Special deals for new users or specific courses that are trending.
Udemy makes it a point to encourage lifelong learning, often using aggressive promotional strategies that can lighten the financial load on students.
Keeping track of these promotions is worth the effort. Many seasoned learners have developed a habit of waiting for the right moment to enroll, allowing them to benefit from substantial discounts. However, not every course goes on sale frequently. Thus, if a course catches your eye, it's worth considering enrolling promptly if the price feels right.
In summary, understanding Udemy’s pricing model and remaining vigilant for discounts can significantly impact your educational expenses. Properly navigating these elements can enable learners to gain valuable skills in data science without the always-heavy financial burden.
Certification and Career Prospects
In the realm of data science, obtaining certification acts as a beacon, guiding students and professionals alike toward enhanced career opportunities. As the field continues to expand, the role of certifications becomes increasingly pivotal for many looking to break into or advance within the industry. The journey through data science can be daunting; thus, certifications not only validate one’s skills but also serve as a powerful tool in distinguishing oneself from a crowded candidate pool.
Udemy Certificates of Completion
Udemy offers a wealth of courses, each culminating in a certificate of completion. This certificate, while not as esteemed as a degree from a traditional university, holds value in specific contexts. Here’s why:
- Validation of Knowledge: Completing a course signifies a commitment to learning. It shows potential employers that the individual has put in the time and effort to acquire new skills.
- Skill Development: Each certificate reflects competencies gained throughout the course. Be it Python programming, machine learning, or data visualization, the specifics mentioned help illuminate a learner’s area of expertise.
- Portfolio Building: For job seekers, presenting certificates can bolster a portfolio. It showcases a proactive approach to self-improvement and can be the key to landing that interview.
"A chart without clear title and labels is like a book without a cover, you know? It's informative but also confusing!"
While Udemy certificates are great, they typically aren’t recognized by all employers. Thus, one should always verify how specific companies view these certifications before relying on them for their job applications.
How Certification Impacts Job Opportunities
The crux of pursuing certification in data science is to enhance job opportunities. The impact can be profound, particularly in a market buzzing with competition. Here’s how it plays out:
- Increased Employability: Certifications can open doors. Hiring managers often prefer candidates with demonstrable knowledge and skills, and certifications provide tangible proof of one’s capabilities.
- Higher Earning Potential: In many cases, certified individuals command higher salaries compared to their non-certified peers. This can significantly enhance one’s overall career trajectory.
- Expanding Network: Enrolling in courses often provides entry into exclusive groups or forums. Networking with fellow students and instructors can lead to valuable connections in the industry.
- Career Transitions: For those looking to pivot into a new role within data science, certifications can facilitate smoother transitions. It communicates an investment in developing the necessary skills, thereby reducing the perceived risk for employers.
To maximize the benefits, prospective job seekers should align their certifications with specific career goals. Certifying in trending areas, like artificial intelligence or big data analytics, can offer a competitive edge against others in the job market.
In summary, while certifications provide a stepping stone into the data science world, they are not a silver bullet. A balanced approach, integrating practical experience with certification, will yield the best results in one’s career journey.
Accessibility and Learning Environment
In the realm of online education, particularly with the rise of data science courses on platforms like Udemy, accessibility and learning environment play a pivotal role. It's not just about having a course and a curriculum; how students engage with the material can significantly impact their overall learning outcomes. Accessibility ensures that anyone who is eager to learn can do so without unnecessary barriers. This includes accommodating various learning styles and ensuring that resources are available for individuals with disabilities.
Platform User Interface
A well-designed platform user interface (UI) can be the difference between a smooth learning experience and a frustrating one. Udemy's user interface is crafted with the user in mind. It allows for easy navigation through courses, making it simple to find lessons, access resources, or revisit past lectures. Particularly for a discipline as intricate as data science, where topics such as machine learning or data processing can be dense, having a clean UI can facilitate focused learning rather than tech troubles.
Notably, Udemy offers features like progress tracking through the course, which can motivate learners to finish what they've started. A visual representation of completed and outstanding sections adds a layer of gamification that appeals to most students' psychology.
Device Compatibility
In this day and age, flexibility is key. The fact that Udemy courses are accessible on multiple devices—their website is compatible with desktops and their app is available on both Android and iOS—gives users the freedom to learn on the go. Some may prefer the comfort of the sofa with a laptop, while others might catch up during a commute on their phones.
This compatibility means you can view lectures, complete assignments, and interact with fellow students at your convenience, which is especially handy in a busy tech-driven life. It encourages more people to enroll since one isn't tethered to a specific setting to gain knowledge. Students can learn at their own pace, making it easier to fit education into busy schedules.
"The digital shift in education has made learning more accessible, allowing individuals to engage from virtually anywhere."
In summary, ensuring that a learning platform like Udemy provides a user-friendly interface complemented by multi-device support promotes a truly accessible educational experience. It's about removing hurdles that could prevent eager minds from soaking in knowledge and equipping them for their future endeavors.
Comparative Analysis with Other Platforms
When discussing the landscape of online education in data science, comparing Udemy to other platforms can provide valuable insights. As the field of data science continues to grow, it becomes increasingly important for learners to evaluate where they can best invest their time and resources. With a multitude of options available, understanding the strengths and weaknesses of each platform can help students make informed decisions about their educational journey and career development.
Udacity and Coursera
Udacity and Coursera are two prominent platforms that often find themselves in the same conversation as Udemy. They have their own unique selling points, shaped by their course offerings and methodologies.
Udacity specializes in what it calls "Nanodegree programs," which are designed in collaboration with leading tech companies. This focus allows students to gain skills that are in direct demand in the job market. The hands-on projects associated with these programs are a significant aspect, encouraging practical learning. However, it’s worth noting that these courses often come with a higher price tag compared to most Udemy offerings.
On the other hand, Coursera partners with renowned universities and institutions to deliver courses that carry academic weight. Their certifications can add credibility to a learner's resume. Like Udacity, many courses on Coursera offer project-based components, but the emphasis can be more theoretical. This balance between theory and practice appeals to many but might not cater to those who are looking for hands-on experience only.
Pros and Cons of Each Platform
Udemy
- Pros:
- Cons:
- Extensive variety of courses covering a broad range of data science topics.
- More affordable than many other platforms, frequently offering discounts.
- Flexibility to learn at one's own pace without stringent deadlines.
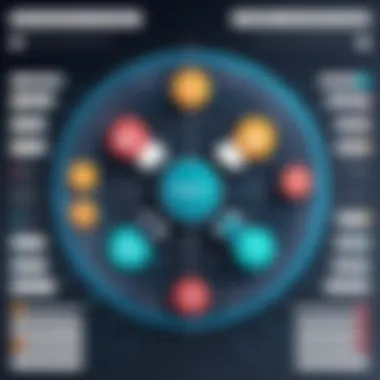
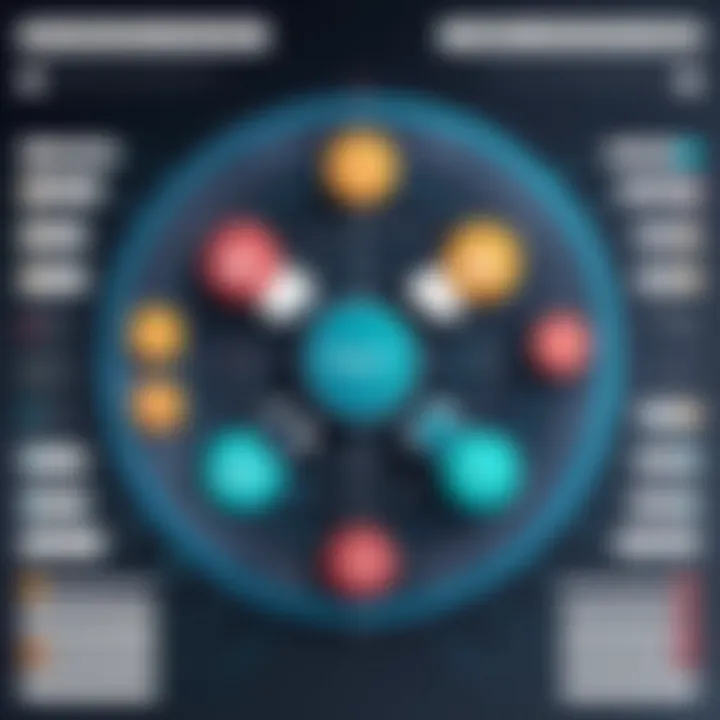
- The quality of courses can vary significantly since anyone can create a course.
- Lesser emphasis on certification recognition in the job market when compared to university-backed platforms.
Udacity
- Pros:
- Cons:
- Strong industry ties ensure relevant content that meets current job market demands.
- High-quality, project-based learning provides real-world scenarios.
- Higher pricing compared to other platforms may deter budget-conscious learners.
- Limited course variety compared to other platforms, focusing only on careers in tech.
Coursera
- Pros:
- Cons:
- Courses are authored by reputable educational institutions, adding credibility.
- Wide range of subjects and specialization options available.
- More structured learning paths can be restrictive for some learners.
- Costs of acquiring full certificates can add up quickly, making it less affordable for casual learners.
Deciding where to study data science ultimately hinges on a learner’s goals, budget, and learning preferences. Each platform has its own merit, thus understanding the comparative aspects is key to making an informed choice > "Only by discerning these differences can students pinpoint the path that aligns with their career aspirations.
For more information on these platforms and their offerings, visit Udacity, Coursera, and Wikipedia on Online Education.
Future Trends in Data Science Education
In the rapidly evolving field of data science, staying ahead of the curve often means being aware of the latest trends in education. As more professionals pivot toward data-driven roles, the demand for innovative educational methods has surged. This is especially crucial when considering how these trends can shape learning experiences on platforms like Udemy. Embracing these trends is not just a necessity but also a strategic advantage for aspiring data scientists. The importance lies in understanding how to utilize these trends to enhance one’s skills, making them more appealing in a competitive market.
Emerging Technologies in Data Science Training
The integration of emerging technologies into data science education can't be overlooked. Technologies such as augmented reality (AR) and virtual reality (VR) are now beginning to find their way into training modules. Imagine donning a VR headset and diving into a virtual lab to visualize complex data sets. This immersive experience allows for better retention of information and a deeper understanding of intricate machine learning concepts.
Furthermore, advancements in online collaborative tools have transformed how learners interact. Many courses on Udemy now leverage platforms like Slack or Zoom to not only deliver lectures but also foster a community among students. This collaboration mirrors real-world working environments, better preparing learners for actual data science roles. Always remember to keep an eye on these technological movements; they can significantly enhance the learning experience and provide an edge over those who might shy away from such advancements.
- Benefits of Emerging Technologies in Education:
- Interactive Learning: Enhances engagement through active participation.
- Real-World Simulations: Allows learners to practice in realistic settings.
- Collaborative Learning: Encourages peer interaction, replicating workplace dynamics.
The Role of Artificial Intelligence in Course Development
Artificial intelligence is reshaping course development in a multitude of ways. Udemy, like many educational platforms, is beginning to implement AI-driven analytics to tailor learning experiences to individual student needs. These technologies can assess a student’s strengths and weaknesses, automatically suggesting courses or learning materials based on performance history. Imagine receiving personalized feedback that adapts each week, honing in on skills that require more attention.
Moreover, AI is streamlining the creation of courses, helping educators focus on content rather than logistics. For instance, tools powered by AI can assist instructors in curating the curriculum. This not only saves time but ensures that courses remain relevant and cutting-edge. The integration of AI goes further, enabling adaptive learning environments where content is delivered dynamically based on student engagement levels.
"The future of education will rely heavily on not just what we learn, but how we adapt and engage with that knowledge."
In summary, understanding the future trends in data science education is vital. Emerging technologies and AI are not just buzzwords; they are practical tools that can lead to more effective learning experiences. Students and professionals in the IT field should stay informed about these dynamics, ensuring their skills remain relevant to industry demands. As you navigate your educational journey, consider how these trends might influence your learning path.
Challenges in Online Learning
Navigating through the world of online education can be a bit like trying to find a needle in a haystack, especially with platforms like Udemy offering a myriad of data science courses. While the flexibility and accessibility of online learning are its selling points, there are hurdles that learners often encounter. Understanding these challenges isn't just academic; it's crucial for anyone hoping to maximize their learning experience and outcomes. This section delves into significant issues that learners face when pursuing data science courses online, which can influence their ability to succeed.
Self-Motivation and Discipline
Online learning requires a significant amount of self-discipline and intrinsic motivation. Unlike traditional classrooms where a teacher keeps you accountable, in the realm of online courses, it's often up to the individual to stay committed. This lack of external structure can lead to procrastination and a slippery slope of falling behind.
- Setting a Routine: Establishing a learning schedule can greatly enhance motivation. Whether it's watching video lectures at the same time every day or dedicating weekends for projects, consistency helps.
- Goal Setting: Another effective tactic is to set achievable goals. Breaking down coursework into smaller, manageable tasks can provide a sense of accomplishment and keep spirits high.
- Finding a Study Buddy: Collaborating with peers can also inject some motivation. Discussing lessons or working on projects with someone else can create a supportive learning environment.
Ultimately, self-motivation and discipline are key ingredients for success in any online learning endeavor. The ability to manage time effectively, set goals, and seek support can drastically enhance the educational experience.
Quality Control of Course Offerings
With over 150,000 courses available on Udemy, the diversity of options can sometimes feel overwhelming – daunting, in fact. However, this variety presents a unique challenge: how to ensure the quality of these courses? Not every course is created equal, and learners need a way to navigate through the offerings to select courses that truly meet their educational needs.
- Course Ratings and Reviews: One of the best ways to gauge a course's quality is through student reviews and ratings. Prospective learners should take the time to read comments carefully, paying attention to both the positive and the negative feedback. High ratings might suggest a well-structured course, but transparency in reviews can provide deeper insights.
- Instructor Credentials: Before enrolling, checking the instructor’s background can save a lot of future headaches. Experienced instructors who have been in the field, such as those who have worked on real-world data science projects, typically offer more valuable insights than those without hands-on experience.
"The sheer volume of courses is a double-edged sword; it empowers learners but also demands due diligence in research."
- Content Updates: In a fast-evolving field like data science, it's paramount to ensure that course content is current and relevant. Courses that have not been updated in years may not cover modern tools or methods, rendering them less useful.
In sum, taking the time to evaluate course offerings critically not only helps in avoiding poor quality courses but can also lead to a richer, more fulfilling online learning experience.
Concluding Thoughts
In the ever-evolving realm of data science education, a structured summary is not just helpful; it's essential. This concluding segment encapsulates the critical insights gleaned throughout the exploration of data science courses on Udemy. As prospective students sift through countless options, understanding the pivotal elements discussed is crucial for making well-informed choices.
Summarizing Key Takeaways
The key to navigating the vast landscape of Udemy's data science offerings lies in a few notable points:
- Diverse Course Options: Udemy showcases a wide range of data science courses encompassing everything from basics to advanced techniques. Whether you are a newbie or an experienced individual looking to polish your skills, there's something tailored for each learner.
- Instructor Expertise: The qualifications and experiences of instructors play a significant role in how effectively students learn. Analyzing instructor ratings and feedback can guide learners towards reputable teachers.
- User Engagement: Feedback from past students provides insights into course quality, structure, and teaching methods, enabling prospective learners to align their expectations with reality.
- Accessibility: The user-friendly platform and the range of devices compatible with Udemy courses empower learners to learn at their convenience.
- Cost-Efficiency: Udemy's pricing model often makes quality education more accessible, with frequent sales and discounts making courses affordable.
In short, these elements not only equip students with valuable insights into what to expect but also help ensure that their educational journey aligns with their professional aspirations.
Final Recommendations for Prospective Students
When diving into the sea of data science courses on Udemy, here are some recommendations:
- Identify Your Goals: Before selecting a course, it's essential to determine what specific skills or knowledge you aim to acquire. This will help narrow down choices effectively.
- Research Instructors: Spend time reviewing instructors’ credentials and student feedback. Look beyond just the course ratings; understanding the teaching style can greatly impact your learning experience.
- Try Sample Lectures: Many courses offer introductory lectures or previews. Take advantage of these to gauge whether the teaching style resonates with you.
- Look for Learner Projects: Opt for courses with hands-on projects that mimic real-world scenarios. Such practical experience enhances learning and builds a robust portfolio.
- Engage with Course material: Don’t just watch videos – participate in discussions, forums, or groups related to the course content. Engaging with fellow learners can enrich your understanding.
Taking these recommendations into account, prospective students can navigate the myriad of available courses more effectively, ultimately leading to a more fruitful educational experience.